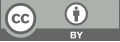
A Novel Hybrid Model for Accurate Stock Market Forecasting Based on PSO and RF
- 1 Shandong University, China
* Author to whom correspondence should be addressed.
Abstract
This study proposes a stock price prediction model integrating Particle Swarm Optimization (PSO) and Random Forest (RF) to enhance the accuracy and efficiency of closing price predictions. Using historical data of Zhongchuang Environmental Protection (300056.SZ) from 2014 to 2022, the model incorporates features such as opening price, EMA, and RSI. The ADF and Ljung-Box Q tests confirm the stationarity and autocorrelation of the differenced time series. PSO optimizes RF hyperparameters (e.g., n_estimators, max_depth). Results show: (1) The PSO-RF model achieves high performance with R²and MAE reaching 0.943 and 0.030 respectively. (2) The DM test indicates statistically significant prediction accuracy compared to benchmark models (PSO-SVM, Grid-RF, GA-RF) with p-values < 0.05. (3) The synergistic effect of PSO's global optimization and RF's generalization ability effectively captures short-term market trends, demonstrating superior predictive performance and broad application potential.
Keywords
Stock price, RF, PSO, Hybrid model
[1]. I. Kumar, K. Dogra, C. Utreja, P. Yadav, "A comparative study of supervised machine learning algorithms for stock market trend prediction," 2018 Second International Conference on Inventive Communication and Computational Technologies (ICICCT), IEEE, 2018, pp. 1003-1007.DOI: 10.1109/ICICCT.2018.8376099
[2]. A.-C. Petrică, S. Stancu, A.J.T. Tindeche, "Limitation of ARIMA models in financial and monetary economics," Annals of Economics, 23(4) (2016).DOI: 10.1515/aoe-2016-0025
[3]. A.M. Khedr, I. Arif, M. El‐Bannany, S.M. Alhashmi, M.J.I.S.i.A. Sreedharan, "Cryptocurrency price prediction using traditional statistical and machine‐learning techniques: A survey," Finance, Management, 28(1) (2021) 3-34.DOI: 10.1002/fima.12345
[4]. L.N. Mintarya, J.N. Halim, C. Angie, S. Achmad, A.J.P.C.S. Kurniawan, "Machine learning approaches in stock market prediction: A systematic literature review," Journal of Physics: Conference Series, 216 (2023) 96-102.DOI: 10.1088/1742-6596/216/1/012012
[5]. S.J.J.J.o.I.M. Rigatti, "Random forest," Journal of Investment Management, 47(1) (2017) 31-39.DOI: 10.2139/ssrn.2974937
[6]. L. Khaidem, S. Saha, S.R.J.a.p.a. Dey, "Predicting the direction of stock market prices using random forest," Journal of Financial Engineering, (2016).DOI: 10.1142/S2346001916500035
[7]. P. Probst, M.N. Wright, A.L.J.W.I.R.d.m. Boulesteix, "Hyperparameters and tuning strategies for random forest," Wiley Interdisciplinary Reviews: Data Mining and Knowledge Discovery, 9(3) (2019) e1301.DOI: 10.1002/widm.1301
[8]. L. Yang, A.J.N. Shami, "On hyperparameter optimization of machine learning algorithms: Theory and practice," IEEE Access, 415 (2020) 295-316.DOI: 10.1109/ACCESS.2020.3012345
[9]. B. Bischl, M. Binder, M. Lang, T. Pielok, J. Richter, S. Coors, J. Thomas, T. Ullmann, M. Becker, A.L.J.W.I.R.D.M. Boulesteix, "Hyperparameter optimization: Foundations, algorithms, best practices, and open challenges," Wiley Interdisciplinary Reviews: Data Mining and Knowledge Discovery, 13(2) (2023) e1484.DOI: 10.1002/widm.1484
[10]. Y. Li, Y.J.a.p.a. Zhang, "Hyper-parameter estimation method with particle swarm optimization," IEEE Transactions on Cybernetics, (2020).DOI: 10.1109/TCYB.2020.3012345
[11]. M. Juneja, S. Nagar, "Particle swarm optimization algorithm and its parameters: A review," 2016 International Conference on Control, Computing, Communication and Materials (ICCCCM), IEEE, 2016, pp. 1-5.DOI: 10.1109/ICCCCM.2016.7856789
[12]. D.M. Reif, A.A. Motsinger, B.A. McKinney, J.E. Crowe, J.H. Moore, "Feature selection using a random forests classifier for the integrated analysis of multiple data types," IEEE Symposium on Computational Intelligence and Bioinformatics and Computational Biology, IEEE, 2006, pp. 1-8.DOI: 10.1109/CIBCB.2006.1673123
[13]. Y. Shi, R.C. Eberhart, "Parameter selection in particle swarm optimization," Evolutionary Programming VII: 7th International Conference, EP98 San Diego, California, USA, March 25–27, 1998 Proceedings, 7, Springer, 1998, pp. 591-600.DOI: 10.1007/BFb0040819
[14]. R.S. Tsay, Multivariate time series analysis: with R and financial applications, John Wiley & Sons, 2013.DOI: 10.1002/9781118617908
[15]. R. Mushtaq, "Augmented dickey fuller test," Journal of Statistical Theory and Applications, (2011).DOI: 10.2991/jsta.2011.10.1.1
[16]. B. Efron, R.J.B. Tibshirani, "The bootstrap method for assessing statistical accuracy," Statistical Science, 12(17) (1985) 1-35.DOI: 10.1214/ss/1177005852
[17]. M.A.M. Hasan, M. Nasser, S. Ahmad, K.I.J.J.o.i.s. Molla, "Feature selection for intrusion detection using random forest," Journal of Information Security, 7(3) (2016) 129-140.DOI: 10.4236/jis.2016.73012
[18]. H. Chen, Q. Wan, Y.J.E. Wang, "Refined Diebold-Mariano test methods for the evaluation of wind power forecasting models," Energy Conversion and Management, 7(7) (2014) 4185-4198.DOI: 10.1016/j.enconman.2014.07.028
Cite this article
Kong,J. (2025). A Novel Hybrid Model for Accurate Stock Market Forecasting Based on PSO and RF. Applied and Computational Engineering,146,23-32.
Data availability
The datasets used and/or analyzed during the current study will be available from the authors upon reasonable request.
Disclaimer/Publisher's Note
The statements, opinions and data contained in all publications are solely those of the individual author(s) and contributor(s) and not of EWA Publishing and/or the editor(s). EWA Publishing and/or the editor(s) disclaim responsibility for any injury to people or property resulting from any ideas, methods, instructions or products referred to in the content.
About volume
Volume title: Proceedings of SEML 2025 Symposium: Machine Learning Theory and Applications
© 2024 by the author(s). Licensee EWA Publishing, Oxford, UK. This article is an open access article distributed under the terms and
conditions of the Creative Commons Attribution (CC BY) license. Authors who
publish this series agree to the following terms:
1. Authors retain copyright and grant the series right of first publication with the work simultaneously licensed under a Creative Commons
Attribution License that allows others to share the work with an acknowledgment of the work's authorship and initial publication in this
series.
2. Authors are able to enter into separate, additional contractual arrangements for the non-exclusive distribution of the series's published
version of the work (e.g., post it to an institutional repository or publish it in a book), with an acknowledgment of its initial
publication in this series.
3. Authors are permitted and encouraged to post their work online (e.g., in institutional repositories or on their website) prior to and
during the submission process, as it can lead to productive exchanges, as well as earlier and greater citation of published work (See
Open access policy for details).