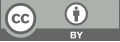
Review on the coupling and promotion of machine learning methods to CFD
- 1 Hohai university, Nanjing, Jiangsu Province, China
* Author to whom correspondence should be addressed.
Abstract
As the computer level advances in 1990s, CFD (the computational fluid dynamics, for short) has promoted fast. The process of using CFD to analyze complex or ideal conditions has a very wide application background. However, confronted by cases with higher accuracy as well as larger number of samples, CFD needs to spend much time and money for the sake of resolving the issues. ML (Machine learning, for short) approach provides a promising choice for CFD. This paper reviews the coupling of ML and CFD and the progress in promoting the application of CFD. This paper briefly introduces CFD along with ML approaches, such as supervised learning, unsupervised learning and reinforcement learning. This article also discusses challenges and issues with the aim of being resolved in the research of ML model based on CFD, such as using multiple machine learning models or hybrid models to solve problems and quantifying the uncertainty of machine learning models. If these problems are solved, ML method can provide a promising development prospect for CFD.
Keywords
Machine Learning, CFD, Promotion
[1]. Umenweke Great C., Afolabi Inioluwa Christianah, Epelle Emmanuel I., Okolie Jude A.. Machine learning methods for modeling conventional and hydrothermal gasification of waste biomass: A review[J]. Bioresource Technology Reports,2022,17.
[2]. Wang J J. Study on optimization of slagging prevention furnace for waste incinerator based on CFD numerical simulation [D]. Zhejiang university,2022. DOI:10.27461/d.cnki.gzjdx.2022.000154.
[3]. Qiu Fang, Zhe Li,Yaonan Wang,Mengxuan Song,Jun Wang. A neural-network enhanced modeling method for real-time evaluation of the temperature distribution in a data center[J]. Neural Computing and Applications,2019,31(12).
[4]. Wakes Sarah J.,Bauer Bernard O.,Mayo Michael. A preliminary assessment of machine learning algorithms for predicting CFD-simulated wind flow patterns over idealised foredunes[J]. Journal of the Royal Society of New Zealand,2021,51(2).
[5]. Moiz A A, Pal P, Probst D, et al. A machine learning-genetic algorithm (ML-GA) approach for rapid optimization using high-performance computing[J]. Society of Automotive Engineers Technical Paper Series, 2018, 11(5).
[6]. Xu P, Babanezhad M, Yarmand H, et al. Flow visualization and analysis of thermal distribution for the nanofluid by the integration of fuzzy c-means clustering ANFIS structure and CFD methods[J]. Journal of Visualization, 2020, 23(1): 97-110.
[7]. Singh Samrendra K.,Braginsky Daniel,Tamamidis Panos,Gennaro Monacelli. Designing Next Generation Exhaust Aftertreatment Systems Using Machine Learning[J]. SAE International Journal of Commercial Vehicles,2020,13(3).
[8]. Li Haochen,Sansalone John. A CFD-ML augmented alternative to residence time for clarification basin scaling and design.[J]. Water research,2021,209.
[9]. Qidwai Mohammad Owais,Badruddin Irfan Anjum,Khan Noor Zaman,Khan Mohammad Anas,Alshahrani Saad. Optimization of Microjet Location Using Surrogate Model Coupled with Particle Swarm Optimization Algorithm[J]. Mathematics,2021,9(17).
[10]. Yang Siqi,Fan Jianchun,Zhang Laibin,Sun Bingcai. Performance prediction of erosion in elbows for slurry flow under high internal pressure[J]. Tribology International,2021(prepublish).
[11]. Song Dooguen,Lee Kwangho,Phark Chuntak,Jung Seungho. Spatiotemporal and Layout-adaptive Prediction of Leak Gas Dispersion by Encoding-Prediction Neural Network[J]. Process Safety and Environmental Protection,2021(prepublish).
Cite this article
Zheng,H. (2023). Review on the coupling and promotion of machine learning methods to CFD. Applied and Computational Engineering,4,52-57.
Data availability
The datasets used and/or analyzed during the current study will be available from the authors upon reasonable request.
Disclaimer/Publisher's Note
The statements, opinions and data contained in all publications are solely those of the individual author(s) and contributor(s) and not of EWA Publishing and/or the editor(s). EWA Publishing and/or the editor(s) disclaim responsibility for any injury to people or property resulting from any ideas, methods, instructions or products referred to in the content.
About volume
Volume title: Proceedings of the 3rd International Conference on Signal Processing and Machine Learning
© 2024 by the author(s). Licensee EWA Publishing, Oxford, UK. This article is an open access article distributed under the terms and
conditions of the Creative Commons Attribution (CC BY) license. Authors who
publish this series agree to the following terms:
1. Authors retain copyright and grant the series right of first publication with the work simultaneously licensed under a Creative Commons
Attribution License that allows others to share the work with an acknowledgment of the work's authorship and initial publication in this
series.
2. Authors are able to enter into separate, additional contractual arrangements for the non-exclusive distribution of the series's published
version of the work (e.g., post it to an institutional repository or publish it in a book), with an acknowledgment of its initial
publication in this series.
3. Authors are permitted and encouraged to post their work online (e.g., in institutional repositories or on their website) prior to and
during the submission process, as it can lead to productive exchanges, as well as earlier and greater citation of published work (See
Open access policy for details).