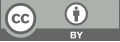
An Improved U-Net Model for Ultrasound Image Segmentation of Breast Cancer
- 1 School of Management and Engineering, Capital University of Economics and Business, No. 121, Zhangjialukou, Huaxiang, Fengtai District, Beijing, 100070, China
* Author to whom correspondence should be addressed.
Abstract
Breast cancer is the most common and one of the most lethal malignant tumors among women worldwide. Early and accurate diagnosis plays a crucial role in improving patient survival rates. As one of the primary imaging modalities, breast ultrasound imaging has been widely employed in clinical screening due to its low cost and lack of radiation exposure. However, limited by its imaging mechanism, ultrasound images often suffer from severe speckle noise interference, blurred boundaries, and complex tissue structures, which significantly hinder the performance of automatic lesion segmentation. To address this challenge, this paper proposes an improved Attention U-Net model. By introducing Attention Gate modules into the conventional U-Net architecture, the model is guided to focus on salient regions associated with lesions while suppressing background interference. Moreover, the network depth is increased to enhance feature representation capabilities. As a result, the proposed model achieves improved segmentation accuracy and boundary fitting performance in complex scenarios.
Keywords
breast cancer, ultrasound imaging, image segmentation, U-Net, attention mechanism, deep learning
[1]. M. Arnold et al., “Current and future burden of breast cancer: Global statistics for 2020 and 2040,” The Breast, vol. 66, pp. 15–23, Dec. 2022, doi: 10.1016/j.breast.2022.08.010.
[2]. O. Ronneberger, P. Fischer, and T. Brox, “U-Net: Convolutional Networks for Biomedical Image Segmentation,” in Medical Image Computing and Computer-Assisted Intervention – MICCAI 2015, N. Navab, J. Hornegger, W. M. Wells, and A. F. Frangi, Eds., Cham: Springer International Publishing, 2015, pp. 234–241. doi: 10.1007/978-3-319-24574-4_28.
[3]. J. Long, E. Shelhamer, and T. Darrell, “Fully Convolutional Networks for Semantic Segmentation,” presented at the Proceedings of the IEEE Conference on Computer Vision and Pattern Recognition, 2015, pp. 3431–3440. Accessed: Mar. 29, 2025. [Online]. Available: https://openaccess.thecvf.com/content_cvpr_2015/html/Long_Fully_Convolutional_Networks_2015_CVPR_paper.html
[4]. W. Al-Dhabyani, M. Gomaa, H. Khaled, and A. Fahmy, “Dataset of breast ultrasound images,” Data in Brief, vol. 28, p. 104863, Feb. 2020, doi: 10.1016/j.dib.2019.104863.
[5]. K. Han et al., “A Survey on Vision Transformer,” IEEE Transactions on Pattern Analysis and Machine Intelligence, vol. 45, no. 1, pp. 87–110, Jan. 2023, doi: 10.1109/TPAMI.2022.3152247.
[6]. Z. Liu et al., “Swin Transformer: Hierarchical Vision Transformer Using Shifted Windows,” presented at the Proceedings of the IEEE/CVF International Conference on Computer Vision, 2021, pp. 10012–10022. Accessed: Mar. 29, 2025. [Online]. Available: https://openaccess.thecvf.com/content/ICCV2021/html/Liu_Swin_Transformer_Hierarchical_Vision_Transformer_Using_Shifted_Windows_ICCV_2021_paper
[7]. A. Kirillov et al., “Segment Anything,” presented at the Proceedings of the IEEE/CVF International Conference on Computer Vision, 2023, pp. 4015–4026. Accessed: Mar. 29, 2025. [Online]. Available: https://openaccess.thecvf.com/content/ICCV2023/html/Kirillov_Segment_Anything_ICCV_2023_paper.html
[8]. K. Weiss, T. M. Khoshgoftaar, and D. Wang, “A survey of transfer learning,” J Big Data, vol. 3, no. 1, p. 9, May 2016, doi: 10.1186/s40537-016-0043-6.
[9]. Z. Li et al., “Cross-modality representation and multi-sample integration of spatially resolved omics data,” Jun. 11, 2024, bioRxiv. doi: 10.1101/2024.06.10.598155.
[10]. R. Krishnan, P. Rajpurkar, and E. J. Topol, “Self-supervised learning in medicine and healthcare,” Nat. Biomed. Eng, vol. 6, no. 12, pp. 1346–1352, Dec. 2022, doi: 10.1038/s41551-022-00914-1.
Cite this article
Guo,P. (2025). An Improved U-Net Model for Ultrasound Image Segmentation of Breast Cancer. Applied and Computational Engineering,150,27-34.
Data availability
The datasets used and/or analyzed during the current study will be available from the authors upon reasonable request.
Disclaimer/Publisher's Note
The statements, opinions and data contained in all publications are solely those of the individual author(s) and contributor(s) and not of EWA Publishing and/or the editor(s). EWA Publishing and/or the editor(s) disclaim responsibility for any injury to people or property resulting from any ideas, methods, instructions or products referred to in the content.
About volume
Volume title: Proceedings of the 3rd International Conference on Software Engineering and Machine Learning
© 2024 by the author(s). Licensee EWA Publishing, Oxford, UK. This article is an open access article distributed under the terms and
conditions of the Creative Commons Attribution (CC BY) license. Authors who
publish this series agree to the following terms:
1. Authors retain copyright and grant the series right of first publication with the work simultaneously licensed under a Creative Commons
Attribution License that allows others to share the work with an acknowledgment of the work's authorship and initial publication in this
series.
2. Authors are able to enter into separate, additional contractual arrangements for the non-exclusive distribution of the series's published
version of the work (e.g., post it to an institutional repository or publish it in a book), with an acknowledgment of its initial
publication in this series.
3. Authors are permitted and encouraged to post their work online (e.g., in institutional repositories or on their website) prior to and
during the submission process, as it can lead to productive exchanges, as well as earlier and greater citation of published work (See
Open access policy for details).