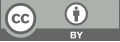
Load Forecasting Method Based on PSO-LSTM
- 1 College of Electronic Information and Automation, Tianjin University of Science and Technology
* Author to whom correspondence should be addressed.
Abstract
Load forecasting plays a crucial role in fields such as energy management and power system planning. Accurate forecasting can effectively reduce energy costs and enhance the stability and reliability of power systems. Traditional forecasting methods struggle to balance forecasting accuracy and efficiency when dealing with complex and variable load data. Although the Long Short-Term Memory network (LSTM) can address the long-term dependency problem in time series and shows certain advantages in load forecasting, the setting of its initial parameters has a significant impact on the forecasting results, and it is prone to getting trapped in local optima. The Particle Swarm Optimization algorithm (PSO), based on swarm intelligence, has powerful global search capabilities and fast convergence. This paper proposes a load forecasting method based on PSO-LSTM, which uses PSO to optimize the parameters of LSTM, thereby enhancing the generalization ability and forecasting accuracy of the model. Through case-based analysis and comparison, it can be seen that compared with traditional forecasting methods, this method effectively improves the accuracy of load forecasting and provides strong support for the efficient and stable operation of power systems.
Keywords
Load Forecasting, PSO, LSTM, Artificial Intelligence
[1]. Wang C, Li Y X, Wang S X, et al. Short - term power load forecasting based on ICEEMDAN decomposition and reconstruction and BiLSTM-KELM [J]. Science Technology and Engineering, 2024, 24(32):13836 - 13843.
[2]. Li X L, Li Z P, Liu J Z. Research on medium - and long - term load forecasting in a certain region based on time - series analysis [J]. Modern Industrial Economy and Informationization, 2024, 14(07):282 - 283+287.
[3]. Fan R Y, Gao Z, Cao H M. Multi - factor prediction model for power monitoring data based on grey theory [J]. Chinese Journal of Electron Devices, 2022, 45(02):427 - 431.
[4]. Wang Y, Li J, Zhang J. Short - term load forecasting method based on the Stacking regression model considering meteorological factors [J]. Electrical Engineering Technology, 2024(17):67 - 70.
[5]. Xu J. Research on a multi - variable short - term load forecasting model optimized by a time - series clustering algorithm [J]. Energy Science and Technology, 2024, 22(02):20 - 23.
[6]. Yang L, Luo X H, Han K. Review of research on power system load forecasting based on artificial intelligence algorithms [J]. Electrical Engineering Technology, 2024(12):57 - 60.
Cite this article
Chen,Z. (2025). Load Forecasting Method Based on PSO-LSTM. Applied and Computational Engineering,149,7-11.
Data availability
The datasets used and/or analyzed during the current study will be available from the authors upon reasonable request.
Disclaimer/Publisher's Note
The statements, opinions and data contained in all publications are solely those of the individual author(s) and contributor(s) and not of EWA Publishing and/or the editor(s). EWA Publishing and/or the editor(s) disclaim responsibility for any injury to people or property resulting from any ideas, methods, instructions or products referred to in the content.
About volume
Volume title: Proceedings of CONF-MSS 2025 Symposium: Automation and Smart Technologies in Petroleum Engineering
© 2024 by the author(s). Licensee EWA Publishing, Oxford, UK. This article is an open access article distributed under the terms and
conditions of the Creative Commons Attribution (CC BY) license. Authors who
publish this series agree to the following terms:
1. Authors retain copyright and grant the series right of first publication with the work simultaneously licensed under a Creative Commons
Attribution License that allows others to share the work with an acknowledgment of the work's authorship and initial publication in this
series.
2. Authors are able to enter into separate, additional contractual arrangements for the non-exclusive distribution of the series's published
version of the work (e.g., post it to an institutional repository or publish it in a book), with an acknowledgment of its initial
publication in this series.
3. Authors are permitted and encouraged to post their work online (e.g., in institutional repositories or on their website) prior to and
during the submission process, as it can lead to productive exchanges, as well as earlier and greater citation of published work (See
Open access policy for details).