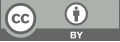
Fault Diagnosis of Transformer Dissolved Gas Based on Random Forest
- 1 School of Electrical and Electronic Engineering, North China Electric Power University, Beijing, China
* Author to whom correspondence should be addressed.
Abstract
To improve the accuracy of transformer fault diagnosis, this paper proposes a transformer fault diagnosis model based on the Random Forest (RF) algorithm. First, the dissolved gas analysis (DGA) method is used to preprocess the concentration data of characteristic gases dissolved in transformer oil. The Random Forest model is then constructed using Bootstrap sampling and random feature selection, achieving high-precision classification of transformer fault types through the integration of multiple decision trees. To validate the effectiveness of the model, actual transformer fault operation data are selected for testing. Additionally, the Random Forest model is compared with five other models: GNB, LDA, KNN, SVM, and GBDT. The results show that the Random Forest model significantly outperforms the other models in terms of both training and testing accuracy, while also demonstrating higher efficiency in training time. The comprehensive performance of the Random Forest model is superior to the comparison models. This study demonstrates that the Random Forest-based transformer fault diagnosis method can effectively handle complex dissolved gas data, accurately identify multiple fault types, and exhibit high generalization ability and robustness, making it suitable for practical transformer fault diagnosis in power systems.
Keywords
Random Forest, transformer, fault diagnosis, DGA
[1]. Wang, X.J. (2024) Research on Fault Diagnosis of Oil-Immersed Transformers. Industrial Innovation Research, (24), 44-46.
[2]. Wan, Q.H., Ye, K.D. and Zhang, S. (2024) Transformer Oil Chromatography Analysis Based on the Three-Ratio Method. Electrical Switchgear, 62(03), 65-67.
[3]. Zheng, H., Li, Y., et al. (2019) A Hybrid Approach for Transformer Fault Diagnosis Using Support Vector Machine and Genetic Algorithm. IEEE Transactions on Power Delivery, 34(2), 789-797.
[4]. Shao, L., Yu, J.J., Liu, H.L., et al. (2025) Transformer Fault Diagnosis Method Based on Hierarchical Clustering and Improved Support Vector Machine. Journal of Tianjin University of Technology, 1-8. Retrieved March 10, 2025, from https://kns-cnki-net.webvpn.ncepu.edu.cn/kcms/detail/12.1374.N.20241202.1110.021.html
[5]. Li, W.J., Li, T.Y. and Su, J. (2025) Series Arc Fault Detection Method for Photovoltaic DC Side Based on Random Forest. Distribution & Utilization, 42(02), 108-115. DOI:10.19421/j.cnki.1006-6357.2025.02.012
Cite this article
Jing,G. (2025). Fault Diagnosis of Transformer Dissolved Gas Based on Random Forest. Applied and Computational Engineering,149,111-115.
Data availability
The datasets used and/or analyzed during the current study will be available from the authors upon reasonable request.
Disclaimer/Publisher's Note
The statements, opinions and data contained in all publications are solely those of the individual author(s) and contributor(s) and not of EWA Publishing and/or the editor(s). EWA Publishing and/or the editor(s) disclaim responsibility for any injury to people or property resulting from any ideas, methods, instructions or products referred to in the content.
About volume
Volume title: Proceedings of CONF-MSS 2025 Symposium: Automation and Smart Technologies in Petroleum Engineering
© 2024 by the author(s). Licensee EWA Publishing, Oxford, UK. This article is an open access article distributed under the terms and
conditions of the Creative Commons Attribution (CC BY) license. Authors who
publish this series agree to the following terms:
1. Authors retain copyright and grant the series right of first publication with the work simultaneously licensed under a Creative Commons
Attribution License that allows others to share the work with an acknowledgment of the work's authorship and initial publication in this
series.
2. Authors are able to enter into separate, additional contractual arrangements for the non-exclusive distribution of the series's published
version of the work (e.g., post it to an institutional repository or publish it in a book), with an acknowledgment of its initial
publication in this series.
3. Authors are permitted and encouraged to post their work online (e.g., in institutional repositories or on their website) prior to and
during the submission process, as it can lead to productive exchanges, as well as earlier and greater citation of published work (See
Open access policy for details).