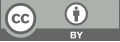
Identification of Molecular Markers to Classify IDC and DCIS/LCIS in Breast Cancer Using Spatial Transcriptomics
- 1 School of Biomedical Engineering and Information Technology, Nanjing Medical University, No. 101, Longmian Avenue, Jiangning District, Nanjing, China
* Author to whom correspondence should be addressed.
Abstract
Breast cancer is one of the most common malignant tumors among women globally, posing significant threats to women's health and lives. Identifying specific molecular markers is crucial for early diagnosis, precision treatment, and accurate prognostic assessment of breast cancer. In this study, spatial transcriptomics technology combined with machine learning methods successfully identified molecular markers capable of effectively distinguishing invasive ductal carcinoma (IDC) from ductal carcinoma in situ (DCIS) and lobular carcinoma in situ (LCIS). By analyzing the gene expression profiles of 36,601 genes across 3,798 cells, significant differentially expressed genes (DEGs) were screened using the DEsingle method. Functional enrichment analysis indicated that these genes are significantly associated with breast cancer-related pathways, breast cell-specific expression, and the regulation of core transcription factors, such as TP53, SP1, and NFKB1. Further classification analysis employing machine learning models including random forest, decision tree, support vector machine, and logistic regression revealed that the random forest model demonstrated the highest performance, achieving an accuracy rate of 95.78%. Ultimately, ten key molecular markers were identified: MGP, ALB, S100G, KRT37, SERPINA3, AC087379.2, ZNF350-AS1, IGHG3, IGHG4, and IGKC. These markers exhibited robust discrimination between IDC and DCIS/LCIS, suggesting their potential roles in tumor invasion and metastasis. This study provides novel molecular evidence for early diagnosis, individualized treatment, and prognostic evaluation of breast cancer, contributing new research insights and theoretical support for precision medicine approaches in breast cancer.
Keywords
breast cancer, spatial transcriptomics, Molecular Markers, biomarker
[1]. S. Libson and M. Lippman, “A review of clinical aspects of breast cancer,” International Review of Psychiatry, vol. 26, no. 1, pp. 4–15, Feb. 2014, doi: 10.3109/09540261.2013.852971.
[2]. J. Wang et al., “Progression from ductal carcinoma in situ to invasive breast cancer: molecular features and clinical significance,” Sig Transduct Target Ther, vol. 9, no. 1, pp. 1–28, Apr. 2024, doi: 10.1038/s41392-024-01779-3.
[3]. L. Moses and L. Pachter, “Museum of spatial transcriptomics,” Nat Methods, vol. 19, no. 5, pp. 534–546, May 2022, doi: 10.1038/s41592-022-01409-2.
[4]. P. Androvic et al., “Spatial Transcriptomics-correlated Electron Microscopy maps transcriptional and ultrastructural responses to brain injury,” Nat Commun, vol. 14, no. 1, p. 4115, Jul. 2023, doi: 10.1038/s41467-023-39447-9.
[5]. D. Dar, N. Dar, L. Cai, and D. K. Newman, “Spatial transcriptomics of planktonic and sessile bacterial populations at single-cell resolution,” Science, vol. 373, no. 6556, p. eabi4882, Aug. 2021, doi: 10.1126/science.abi4882.
[6]. X. Sun et al., “SpaGRA: Graph augmentation facilitates domain identification for spatially resolved transcriptomics,” Journal of Genetics and Genomics, Oct. 2024, doi: 10.1016/j.jgg.2024.09.015.
[7]. Z. Miao, K. Deng, X. Wang, and X. Zhang, “DEsingle for detecting three types of differential expression in single-cell RNA-seq data,” Bioinformatics, vol. 34, no. 18, pp. 3223–3224, Sep. 2018, doi: 10.1093/bioinformatics/bty332.
[8]. J. Piñero et al., “DisGeNET: a comprehensive platform integrating information on human disease-associated genes and variants,” Nucleic Acids Research, vol. 45, no. D1, pp. D833–D839, Jan. 2017, doi: 10.1093/nar/gkw943.
[9]. J.-B. Pan et al., “PaGenBase: A Pattern Gene Database for the Global and Dynamic Understanding of Gene Function,” PLOS ONE, vol. 8, no. 12, p. e80747, Dec. 2013, doi: 10.1371/journal.pone.0080747.
[10]. H. Han et al., “TRRUST: a reference database of human transcriptional regulatory interactions,” Sci Rep, vol. 5, no. 1, p. 11432, Jun. 2015, doi: 10.1038/srep11432.
Cite this article
Zhou,J. (2025). Identification of Molecular Markers to Classify IDC and DCIS/LCIS in Breast Cancer Using Spatial Transcriptomics. Applied and Computational Engineering,150,1-8.
Data availability
The datasets used and/or analyzed during the current study will be available from the authors upon reasonable request.
Disclaimer/Publisher's Note
The statements, opinions and data contained in all publications are solely those of the individual author(s) and contributor(s) and not of EWA Publishing and/or the editor(s). EWA Publishing and/or the editor(s) disclaim responsibility for any injury to people or property resulting from any ideas, methods, instructions or products referred to in the content.
About volume
Volume title: Proceedings of the 3rd International Conference on Software Engineering and Machine Learning
© 2024 by the author(s). Licensee EWA Publishing, Oxford, UK. This article is an open access article distributed under the terms and
conditions of the Creative Commons Attribution (CC BY) license. Authors who
publish this series agree to the following terms:
1. Authors retain copyright and grant the series right of first publication with the work simultaneously licensed under a Creative Commons
Attribution License that allows others to share the work with an acknowledgment of the work's authorship and initial publication in this
series.
2. Authors are able to enter into separate, additional contractual arrangements for the non-exclusive distribution of the series's published
version of the work (e.g., post it to an institutional repository or publish it in a book), with an acknowledgment of its initial
publication in this series.
3. Authors are permitted and encouraged to post their work online (e.g., in institutional repositories or on their website) prior to and
during the submission process, as it can lead to productive exchanges, as well as earlier and greater citation of published work (See
Open access policy for details).