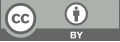
Models for Depression Using Machine Learning Analysis of Indian Survey Data
- 1 The Webb Schools, Claremont, United States
* Author to whom correspondence should be addressed.
Abstract
Understanding the correlation between various risk factors and depression is important for developing personalized and effective treatment plans, especially for those at risk of recurrent or chronic depression. Current literature and predictive models identify pressure and age as primary risk factors, in addition to other factors such as financial stress, employment or student status, and suicidal thoughts. This approach emphasizes the importance of considering a range of interrelated factors that may influence each other and compound over time. Particular attention should be paid to vulnerable and marginalized populations, who are disproportionately affected and have higher prevalence of depression, emphasizing the need for individualized treatment and targeted interventions. High accuracy predictive models are used to assess the impact of individual and combined risk factors, providing a tool for identifying people at risk of depression before symptoms such as somatization become fully manifest. Early identification can lead to more proactive interventions, improved clinical outcomes, and a reduction in the overall impact of depression on society.
Keywords
Depression, risk factors, mental health
[1]. Reinert, Maddy, et al. “The State of Mental Health in America 2024.” Mental Health America, 1 Jul. 2024, http://hdl.handle.net/10713/22688.
[2]. Zhukovsky, Peter, et al. “Generalizability of Treatment Outcome Prediction Across Antidepressant Treatment Trials in Depression.” JAMA Network Open, vol. 8, no. 3, 20 Mar. 2025, JAMA Network, https://doi.org/10.1001/jamanetworkopen.2025.1310.
[3]. Stringaris, Argyris. “Editorial: What is depression?” The Journal of Child Psychology and Psychiatry, vol. 58, no. 12, 17 Nov. 2017, pp. 1287–1289, The Association for Child and Adolescent Mental Health, https://doi.org/10.1111/jcpp.12844.
[4]. Olfson, Mark, et al. “Treatment of Adult Depression in the United States.” JAMA Internal Medicine, vol. 176, no. 10, 29 Aug. 2016, pp. 1482–1491, JAMA Network, https://doi.org/10.1001/jamainternmed.2016.5057.
[5]. Josefsson, T., et al. “Physical exercise intervention in depressive disorders: Meta-analysis and systematic review.” Scandinavian Journal of Medicine & Science in Sports, vol. 24, no. 2, 30 Jan. 2013, John Wiley & Sons, Inc., https://doi.org/10.1111/sms.12050.
[6]. Mofatteh, Mohammad. “Risk factors associated with stress, anxiety, and depression among university undergraduate students.” AIMS public health, vol. 8, no. 1, 25 Dec. 2020, pp. 36–65, National Library of Medicine, https://doi.org/10.3934/publichealth.2021004.
[7]. Hammen, Constance. “Risk Factors for Depression: An Autobiographical Review.” Annual Review of Clinical Psychology, vol. 14, 12 Jan. 2018, pp. 1–28, Annual Reviews, https://doi.org/10.1146/annurev-clinpsy-050817-084811.
[8]. Ramón-Arbués, Enrique, et al. “The Prevalence of Depression, Anxiety and Stress and Their Associated Factors in College Students.” International Journal of Environmental Research and Public Health, vol. 17, no. 19, 24 Sep. 2020, MDPI, https://doi.org/10.3390/ijerph17197001.
[9]. Wang, Jinghui, et al. “Prevalence of depression and depressive symptoms among outpatients: a systematic review and meta-analysis.” BMJ Open, vol. 7, no. 8, 5 July 2017, BMJ Publishing Group Ltd., https://doi.org/10.1136/bmjopen-2017-017173.
[10]. McGorry, Patrick D., and Cristina Mei. “Early intervention in youth mental health: progress and future directions.” BMJ Mental Health, vol. 21, no. 4, 1 Nov. 2018, pp. 182–184, BMJ Publishing Group Ltd., https://doi.org/10.1136/ebmental-2018-300060.
[11]. Ormel, Johan, et al. “More treatment but no less depression: The treatment-prevalence paradox.” Clinical Psychology Review, vol. 91, 24 Dec. 2021, ScienceDirect, https://doi.org/10.1016/j.cpr.2021.102111.
[12]. Fazel, Mina, et al. “Mental health interventions in schools in high-income countries.” The Lancet Psychiatry, vol. 1, no. 5, pp. 377–387, https://doi.org/10.1016/S2215-0366(14)70312-8.
Cite this article
Liu,Y. (2025). Models for Depression Using Machine Learning Analysis of Indian Survey Data. Applied and Computational Engineering,157,1-12.
Data availability
The datasets used and/or analyzed during the current study will be available from the authors upon reasonable request.
Disclaimer/Publisher's Note
The statements, opinions and data contained in all publications are solely those of the individual author(s) and contributor(s) and not of EWA Publishing and/or the editor(s). EWA Publishing and/or the editor(s) disclaim responsibility for any injury to people or property resulting from any ideas, methods, instructions or products referred to in the content.
About volume
Volume title: Proceedings of CONF-CDS 2025 Symposium: Data Visualization Methods for Evaluatio
© 2024 by the author(s). Licensee EWA Publishing, Oxford, UK. This article is an open access article distributed under the terms and
conditions of the Creative Commons Attribution (CC BY) license. Authors who
publish this series agree to the following terms:
1. Authors retain copyright and grant the series right of first publication with the work simultaneously licensed under a Creative Commons
Attribution License that allows others to share the work with an acknowledgment of the work's authorship and initial publication in this
series.
2. Authors are able to enter into separate, additional contractual arrangements for the non-exclusive distribution of the series's published
version of the work (e.g., post it to an institutional repository or publish it in a book), with an acknowledgment of its initial
publication in this series.
3. Authors are permitted and encouraged to post their work online (e.g., in institutional repositories or on their website) prior to and
during the submission process, as it can lead to productive exchanges, as well as earlier and greater citation of published work (See
Open access policy for details).