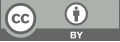
The Segmentation Model for Breast Cancer Ultrasound Image based on Attention U-Net
- 1 Mongolia University of Technology Hohhot, Hohhot City, Inner Mongolia Autonomous Region, 010080, China
* Author to whom correspondence should be addressed.
Abstract
The segmentation of ultrasound image for breast cancer is an important task in the field of biomedical research. The traditional U-Net model, with its simple structure and remarkable performance, this approach has found extensive application in the segmentation of medical images. However, U-Net tends to be affected by background noise when handling images with complex backgrounds or blurry boundaries, which may impact the segmentation accuracy. To address this issue, the Attention U-Net model incorporates an attention mechanism, enabling the model to selectively focus on critical target areas within the image, thereby improving segmentation accuracy. This paper further optimizes the Attention U-Net architecture by increasing the depth of both the encoder and decoder sections, enhancing the model's capacity for feature extraction and image reconstruction. Consequently, both the accuracy and robustness of segmentation are enhanced. The experimental findings indicate that the proposed modified Attention U-Net model significantly outperforms traditional methods in breast ultrasound image segmentation tasks. It effectively handles various types of breast images, particularly those with complex backgrounds, blurred targets, or small sizes, maintaining high segmentation accuracy. This study offers an effective solution for the automated segmentation of breast ultrasound images, with substantial implications for enhancing both the automation and diagnostic efficiency in medical image analysis.
Keywords
Attention U-Net, image segmentation, ultrasound image, breast cancer
[1]. R. Guo, G. Lu, B. Qin, and B. Fei, “Ultrasound imaging technologies for breast cancer detection and management: A review,” Ultrasound in Medicine & Biology, vol. 44, no. 1, pp. 37–70, Jan. 2018, doi: 10.1016/j.ultrasmedbio.2017.09.012.
[2]. O. Ronneberger, P. Fischer, and T. Brox, “U-Net: Convolutional Networks for Biomedical Image Segmentation,” in Medical Image Computing and Computer-Assisted Intervention – MICCAI 2015, N. Navab, J. Hornegger, W. M. Wells, and A. F. Frangi, Eds., Cham: Springer International Publishing, 2015, pp. 234–241. doi: 10.1007/978-3-319-24574-4_28.
[3]. O. Oktay et al., “Attention U-net: Learning where to look for the pancreas,” May 20, 2018, arXiv: arXiv:1804.03999. doi: 10.48550/arXiv.1804.03999.
[4]. M. Zeenath and M. A. Ali, “A review paper on image segmentation and its various techniques in image processing,” International Journal of Research, 2014, Accessed: Feb. 07, 2025. [Online]. Available: https://www.semanticscholar.org/paper/A-Review-Paper-on-Image-Segmentation-and-its-in-Zeenath-Ali/67ac7045ee6f52823929d5caeddb390d06451f0e
[5]. H. G. Kaganami and Z. Beiji, “Region-based segmentation versus edge detection,” in 2009 Fifth International Conference on Intelligent Information Hiding and Multimedia Signal Processing, Sep. 2009, pp. 1217–1221. doi: 10.1109/IIH-MSP.2009.13.
[6]. A. S. Kornilov and I. V. Safonov, “An overview of watershed algorithm implementations in open source libraries,” Journal of Imaging, vol. 4, no. 10, Art. no. 10, Oct. 2018, doi: 10.3390/jimaging4100123.
[7]. J. Long, E. Shelhamer, and T. Darrell, “Fully convolutional networks for semantic segmentation,” presented at the Proceedings of the IEEE Conference on Computer Vision and Pattern Recognition, 2015, pp. 3431–3440. Accessed: Feb. 07, 2025. [Online]. Available: https://openaccess.thecvf.com/content_cvpr_2015/html/Long_Fully_Convolutional_Networks_2015_CVPR_paper.html
[8]. S. Ren, K. He, R. Girshick, and J. Sun, “Faster R-CNN: Towards real-time object detection with region proposal networks,” IEEE Transactions on Pattern Analysis and Machine Intelligence, vol. 39, no. 6, pp. 1137–1149, Jun. 2017, doi: 10.1109/TPAMI.2016.2577031.
[9]. W. Al-Dhabyani, M. Gomaa, H. Khaled, and A. Fahmy, “Dataset of breast ultrasound images,” Data in Brief, vol. 28, p. 104863, Feb. 2020, doi: 10.1016/j.dib.2019.104863.
[10]. R. R. Selvaraju, M. Cogswell, A. Das, R. Vedantam, D. Parikh, and D. Batra, “Grad-CAM: Visual explanations from deep networks via gradient-based localization,” Int J Comput Vis, vol. 128, no. 2, pp. 336–359, Feb. 2020, doi: 10.1007/s11263-019-01228-7.
Cite this article
Cheng,J. (2025). The Segmentation Model for Breast Cancer Ultrasound Image based on Attention U-Net. Applied and Computational Engineering,156,1-10.
Data availability
The datasets used and/or analyzed during the current study will be available from the authors upon reasonable request.
Disclaimer/Publisher's Note
The statements, opinions and data contained in all publications are solely those of the individual author(s) and contributor(s) and not of EWA Publishing and/or the editor(s). EWA Publishing and/or the editor(s) disclaim responsibility for any injury to people or property resulting from any ideas, methods, instructions or products referred to in the content.
About volume
Volume title: Proceedings of CONF-SEML 2025 Symposium: Intefrating AI into Software Engineering
© 2024 by the author(s). Licensee EWA Publishing, Oxford, UK. This article is an open access article distributed under the terms and
conditions of the Creative Commons Attribution (CC BY) license. Authors who
publish this series agree to the following terms:
1. Authors retain copyright and grant the series right of first publication with the work simultaneously licensed under a Creative Commons
Attribution License that allows others to share the work with an acknowledgment of the work's authorship and initial publication in this
series.
2. Authors are able to enter into separate, additional contractual arrangements for the non-exclusive distribution of the series's published
version of the work (e.g., post it to an institutional repository or publish it in a book), with an acknowledgment of its initial
publication in this series.
3. Authors are permitted and encouraged to post their work online (e.g., in institutional repositories or on their website) prior to and
during the submission process, as it can lead to productive exchanges, as well as earlier and greater citation of published work (See
Open access policy for details).