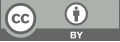
Research on the Application of Speech Recognition Technology Based on Transformer Model
- 1 Computer Science and Communication Engineering College, Jiangsu University, Zhejiang, Jiangsu, China, 210023
* Author to whom correspondence should be addressed.
Abstract
Speech recognition technology has developed from the 1950s to the present, evolving from template matching methods to Hidden Markov Model (HMM) statistical methods, then to machine learning techniques, and finally to the current use of Transformer technology for speech recognition tasks. However, the Transformer model has not yet been widely adopted in the field of speech recognition. This paper explores the characteristics of Transformer model, combines it with the characteristics of speech recognition tasks, analyzes the challenges associated with using Transformer model for these tasks, and provides suggestions for directions of future research, so as to facilitate the application of Transformer models in speech recognition. The paper finds that the reasons for the limited application of Transformer models in speech recognition tasks mainly include their numerous parameters, complex structure, and high computational costs, which have prevented their extensive use in this field. In the future, efforts should focus on enhancing model compression and lightweight design, and improving the attention mechanism to boost the applicability of Transformer models in speech recognition.
Keywords
Transformer, Automatic Speech Recognition, Deep Learning, Computer Science
[1]. Yanhong Wang, Liang Zhao, Guanjun Wang. (2025). Improved voice recognition lightweight design for the Transformer model, Computer Engineering and Applications.
[2]. Junhua Li, Zhikui Duan, Xinmei Yu. (2025). A feature enhancement algorithm based on Transformer model and its application. Journal of Foshan University (Natural Sciences Edition).
[3]. M. A. Anusuya, S. K. Katti. (2009). Speech Recognition by Machine: A Review. International Journal of Computer Science and Information Security, 6(3).
[4]. Santosh K. Gaikwad, Bharti W. GawaIi, Pravin Yannawar. (2010). A Review on speech Recognition Technique. International Journal of computer Applications, 10(3).
[5]. VASWANI A., SHAZEER N., PARMAR N., et al. (2017). Attention is all you need [J]. Advances in Neural Information Processing Systems.
[6]. DONG L., XU S., XU B. (2018). Speech-transformer: a no-recurrence sequence-to-sequence model for speech recognition. In 2018 IEEE International Conference on Acoustics, Speech and Signal Processing (ICASSP). IEEE, 5884-5888.
[7]. GULATI A., QIN J., CHIU C. C., et al. (2020). Conformer: Convolution-augmented Transformer for speech recognition. In Proceedings of Interspeech, 5036-5040.
[8]. WANG J., LIANG Z., ZHANG X., et al. (2024). EfficientASR: Speech Recognition Network Compression via Attention Redundancy and Chunk-Level FFN Optimization [J]. arXiv preprint arXiv: 2404.19214.
[9]. ZENG K., PAIK I. A. (2020). Lightweight transformer with convolutional attention. In 2020 11th International Con- ference on Awareness Science and Technology (iCAST). IEEE, 1-6.
[10]. Yiyang Qian. (2023). Automatic speech recognition and hotword enhancement Algorithm based on Transformer.
Cite this article
Xi,H. (2025). Research on the Application of Speech Recognition Technology Based on Transformer Model. Applied and Computational Engineering,156,34-43.
Data availability
The datasets used and/or analyzed during the current study will be available from the authors upon reasonable request.
Disclaimer/Publisher's Note
The statements, opinions and data contained in all publications are solely those of the individual author(s) and contributor(s) and not of EWA Publishing and/or the editor(s). EWA Publishing and/or the editor(s) disclaim responsibility for any injury to people or property resulting from any ideas, methods, instructions or products referred to in the content.
About volume
Volume title: Proceedings of CONF-SEML 2025 Symposium: Intefrating AI into Software Engineering
© 2024 by the author(s). Licensee EWA Publishing, Oxford, UK. This article is an open access article distributed under the terms and
conditions of the Creative Commons Attribution (CC BY) license. Authors who
publish this series agree to the following terms:
1. Authors retain copyright and grant the series right of first publication with the work simultaneously licensed under a Creative Commons
Attribution License that allows others to share the work with an acknowledgment of the work's authorship and initial publication in this
series.
2. Authors are able to enter into separate, additional contractual arrangements for the non-exclusive distribution of the series's published
version of the work (e.g., post it to an institutional repository or publish it in a book), with an acknowledgment of its initial
publication in this series.
3. Authors are permitted and encouraged to post their work online (e.g., in institutional repositories or on their website) prior to and
during the submission process, as it can lead to productive exchanges, as well as earlier and greater citation of published work (See
Open access policy for details).