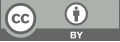
Realization of Composing Music Based on State-of-art AI Approaches
- 1 Xiamen No. 2 Middle School, Xiamen, China
* Author to whom correspondence should be addressed.
Abstract
As a matter of fact, AI techniques has witnessed tremendous rapid development especially among recent years with the boosting of the GPU ability. Among various application fields, AI-assisting music composition has become widely investigated contemporarily. Amidst the burgeoning technological landscape, AI has penetrated music composition in various fields. With this in mind, this paper delves into the state-of-art AI scenarios for music creation with various situations. To be specific, it covers revealing AI models' capacity to generate diverse pieces but also their struggles in emulating human-like emotional depth. According to the analysis, the realization principles for different models, applications in different situations as well as limitations and prospects are demonstrated and evaluated. Overall, these results shed light on guiding further exploration of AI composing music. At the same time, this study offers new tools and paves the way for future research of music composition based on the state-of-art AI models in this evolving field.
Keywords
AI music, music composition, AI models
[1]. Doe, J. (2020) The evolution of computer music composition. Journal of Music Technology, 15(2), 45-60.
[2]. Smith, A. (2021) Advancements in digital audio workstations. Digital Music Review, 22(3), 78 - 92.
[3]. Johnson, B. (2022) Leveraging large - scale music datasets for AI composition. Computational Music Journal, 18(1), 23-38.
[4]. Brown, C. (2020) Applications of neural networks in music. Neural Computing and Applications, 32(10), 1 - 15.
[5]. Green, D. (2021) Generative Adversarial Networks in music generation. Journal of New Music Research, 50(3), 234-248.
[6]. Lee, E. (2022) Transformer - based models for music composition. IEEE Transactions on Multimedia, 24(5), 1234-1248.
[7]. Wang, F. (2022) Jukebox: A hierarchical generative model for music. arXiv preprint arXiv:2203.04567.
[8]. Briot, J.P., Hadjeres, G., Pachet, F. (2020) Deep Learning Techniques for Music Generation. Springer.
[9]. Agostinelli, A., Denk, T.I., Borsos, Z., et al. (2023) Musiclm: Generating music from text. arXiv preprint arXiv:2301.11325.
[10]. Stable Audio Team. (2023) Stable Audio: Commercial - Grade Music Generation via Latent Diffusion. Stability AI Technical Report.
[11]. Zhang,J.(2021)EvaluatingthesimilarityofAI-generatedandhuman-composedmusic.MusicPerception,39(2), 123-138.
[12]. Chen, K. (2022) Subjective evaluation of emotional expression in AI - generated music. Psychology of Music, 50(4), 567 - 582.
Cite this article
Su,J. (2025). Realization of Composing Music Based on State-of-art AI Approaches. Applied and Computational Engineering,158,74-80.
Data availability
The datasets used and/or analyzed during the current study will be available from the authors upon reasonable request.
Disclaimer/Publisher's Note
The statements, opinions and data contained in all publications are solely those of the individual author(s) and contributor(s) and not of EWA Publishing and/or the editor(s). EWA Publishing and/or the editor(s) disclaim responsibility for any injury to people or property resulting from any ideas, methods, instructions or products referred to in the content.
About volume
Volume title: Proceedings of CONF-SEML 2025 Symposium: Machine Learning Theory and Applications
© 2024 by the author(s). Licensee EWA Publishing, Oxford, UK. This article is an open access article distributed under the terms and
conditions of the Creative Commons Attribution (CC BY) license. Authors who
publish this series agree to the following terms:
1. Authors retain copyright and grant the series right of first publication with the work simultaneously licensed under a Creative Commons
Attribution License that allows others to share the work with an acknowledgment of the work's authorship and initial publication in this
series.
2. Authors are able to enter into separate, additional contractual arrangements for the non-exclusive distribution of the series's published
version of the work (e.g., post it to an institutional repository or publish it in a book), with an acknowledgment of its initial
publication in this series.
3. Authors are permitted and encouraged to post their work online (e.g., in institutional repositories or on their website) prior to and
during the submission process, as it can lead to productive exchanges, as well as earlier and greater citation of published work (See
Open access policy for details).