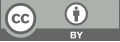
Research on Fraudulent Transaction Detection
- 1 Queen Mary School Hainan, Beijing University of Posts and Telecommunications, Lingshui Li Autonomous County, Hainan Province, China
* Author to whom correspondence should be addressed.
Abstract
With the rapid development of global e-commerce and online payments, the number and complexity of fraudulent transactions have increased significantly, bringing serious economic losses and a crisis of trust to financial institutions and consumers. This paper systematically reviews the characteristics, detection methods and research progress of fraudulent transactions. Firstly, the characteristics of fraudulent transactions are analyzed from the aspects of time, place, transaction mode and technical means. Secondly, the advantages and disadvantages of traditional detection methods (such as rule-based and statistical analysis) and emerging technologies (such as machine learning and deep learning) and their application effects are reviewed. Finally, the limitations of current research in data imbalance, model complexity and multi-type fraud detection are discussed, and future research directions are proposed, including data enhancement techniques, integrated learning frameworks and privacy protection strategies. The research of this paper aims to provide theoretical support and practical guidance for the academic and industrial circles, and promote the further development of the financial security field.
Keywords
Fraudulent transaction detection, Machine learning, Deep learning, Data imbalance, Privacy protection, Financial security
[1]. Xu, K. (2022). Research on the Innovative Development of Motor Vehicle Insurance in the Digital Economy Era [Master's thesis, Guangxi University].
[2]. Al-Zyoud, H., Wang, E. Z., Ali, S., & others. (2024). Can supervisor reminders help prevent fraud in the mutual funds sector. Journal of Financial Crime, 32(2).
[3]. Wang, J., Zhao, S., & Bai, T. (2003). Research on Securities Market Violations and Crimes in Western Regions. Pan Deng, (03), 91–95.
[4]. Cheng, D., Zou, Y., Xiang, S., & others. (2025). Graph neural networks for financial fraud detection: A review. Frontiers of Computer Science, 19(9), 1–15.
[5]. Bintay, R. R., & Joan, T. D. (2024). The risk of fraudulent letter of credit transactions in Bangladesh: A growing threat to Bangladesh’s economy. Journal of Financial Crime, 31(5), 1174–1189.
[6]. Sanzbas, D., Rosal, C. D., Alonso, S. L. N., & others. (2021). Cryptocurrencies and Fraudulent Transactions: Risks, Practices, and Legislation for Their Prevention in Europe and Spain. Laws, 10.
[7]. Sun, X. Q., Shen, H. W., Cheng, X. Q., & others. (2012). Degree-Strength Correlation Reveals Anomalous Trading Behavior. PLOS ONE, 7.
[8]. Manohar, V. G., Bhattacharjee, B., & Pratap, M. (2021). Preventing misuse of discount promotions in e-commerce websites: An application of rule-based systems. International Journal of Services Operations and Informatics, 11(1), 54–74.
[9]. Dong, S. (2024). Research on Fraud Detection Algorithms Based on Graph Neural Networks and Knowledge Reasoning [Master's thesis, Beijing University of Posts and Telecommunications].
[10]. Verma, P., & Tyagi, P. (2022). Credit Card Fraud Detection using Selective Class Sampling and Random Forest Classifier. ECS Transactions, (1), 107.
[11]. Yan, Y. (2016). Analysis and Prevention of Operators' Fraudulent Behavior in Online Transactions. Global Market Information Herald, (33), 1.
[12]. Ding, W. (2025). Research on Credit Card Transaction Fraud Detection Based on Deep Learning Technology [Master's thesis, Shanghai Jiao Tong University].
[13]. Shi, X., & Li, X. (2024). Comparative Study on Detecting Online Fraud Apps Based on Four Machine Learning Algorithms. Information Technology and Informatization, (4), 183–187.
[14]. Yuan, C. (2024). New Ideas for Measurement Management in Fair Trade Markets under the New Situation of Market Supervision. Quality and Market, (07), 39–41.
[15]. Wang, W., Lu, Z., Zhang, J., & others. (2022). Design of Methodology System for Telephone Fraud Behavior Using Random Forest Data Mining Technology. China New Communications, 24(13), 122–124.
[16]. Xiao, W. (2025). Research on Telecom Fraud Identification Based on BP Neural Network [Master's thesis, Central China Normal University].
[17]. Chen, D., & Wu, Y. (2024). Research on the Use of Communication Big Data and AI Artificial Intelligence Technology to Construct Telecom Fraud Prevention Behavior Portrait. Intelligent Decision Technologies: An International Journal, (3), 18.
Cite this article
Cheng,C. (2025). Research on Fraudulent Transaction Detection. Applied and Computational Engineering,158,35-40.
Data availability
The datasets used and/or analyzed during the current study will be available from the authors upon reasonable request.
Disclaimer/Publisher's Note
The statements, opinions and data contained in all publications are solely those of the individual author(s) and contributor(s) and not of EWA Publishing and/or the editor(s). EWA Publishing and/or the editor(s) disclaim responsibility for any injury to people or property resulting from any ideas, methods, instructions or products referred to in the content.
About volume
Volume title: Proceedings of CONF-SEML 2025 Symposium: Machine Learning Theory and Applications
© 2024 by the author(s). Licensee EWA Publishing, Oxford, UK. This article is an open access article distributed under the terms and
conditions of the Creative Commons Attribution (CC BY) license. Authors who
publish this series agree to the following terms:
1. Authors retain copyright and grant the series right of first publication with the work simultaneously licensed under a Creative Commons
Attribution License that allows others to share the work with an acknowledgment of the work's authorship and initial publication in this
series.
2. Authors are able to enter into separate, additional contractual arrangements for the non-exclusive distribution of the series's published
version of the work (e.g., post it to an institutional repository or publish it in a book), with an acknowledgment of its initial
publication in this series.
3. Authors are permitted and encouraged to post their work online (e.g., in institutional repositories or on their website) prior to and
during the submission process, as it can lead to productive exchanges, as well as earlier and greater citation of published work (See
Open access policy for details).