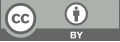
Analysis of reinforce learning in medical treatment
- 1 University of California, Irvine, CA, 92697
* Author to whom correspondence should be addressed.
Abstract
As human approaches the big data period, artificial intelligence becomes dominating in almost every domain. As part of machine learning, reinforcement learning (RL) is intended to utilize mutual communication experiences around the world and assess feedback to strengthen human ability in decision-making. Unlike traditional supervised learning, RL is able to sample, assess and order the delayed feedback decision-making at the same time. This characteristic of RL makes it powerful when it comes to exploring a solution in the medical field. This paper investigates the wide application of RL in the medical field. Including two major parts of the medical field: artificial diagnosis and precision medicine, this paper first introduces several algorithms of RL in each part, then states the inefficiency and unsolved difficulty in this area, together with the future investigation direction of RL. This paper provides researchers with multiple feasible algorithms, supported methods and theoretical analysis, which pave the way for future development of reinforcement learning in medical field.
Keywords
Artificial diagnosis, Precise Medicine, Reinforcement learning, Upper confidence bound, Thompson sampling.
[1]. Chao Yu, Jiming Liu, Shamim Nemti: Reinforcement Learning in Healthcare: A Survey. IEEE, arXiv, 2019, pp.1-23. doi: https://arxiv.org/pdf/1908.08796v4.pdf
[2]. Hongyi Yuan, Sheng Yu: Efficient Symptom Inquiring and Diagnosis via Adaptive Alignment of Reinforcement Learning and Classification. arXiv. 2021, pp.1-7. doi: https://arxiv.org/pdf/2112.00733.pdf.
[3]. Wwwilling, Efficient Symptom Inquiring and Diagnosis via Adaptive Alignment of Reinforcement Learning and Classification. CSDN, 2022. doi: https://blog.csdn.net/qq_43058281/article/details/122903372.
[4]. Samishawl, Upper Confidence Bound Algorithm in Reinforcement Learning. Geeksforgeeks, 2020. doi:https://www.geeksforgeeks.org/upper-confidence-bound-algorithm-in-reinforcement-learning/
[5]. Onur Atan, Mihaela van der Schaar, Discover Relevant Sources: A Multi-armed Bandit Approach. UCLA medianetlab, 2015, pp.1-4. doi: http://medianetlab.ee.ucla.edu/papers/Sourceselection.pdf
[6]. Maryam Aziz, Emilie Kaufmann, Marie-Karelle Riviere: On Multi-armed Bandit Designs for Dose-Finding Clinical Trials. Journal of Machine Learning Research, 2021, pp.1-6. doi: https://www.jmlr.org/papers/volume22/19-228/19-228.pdf.
[7]. Zhijin Zhou, Yingfei Wang, Hamed Mamani: How do Tumor Cytogenetics Inform Cancer Treatments? Dynamic Risk Stratification and Precise Medicine Using Multi-armed Bandits. SSRN, 2019, pp.1-8.
[8]. ADL: An introduction to Q-learning: reinforcement learning. FreeCodeCamp, 2018. doi: https://www.freecodecamp.org/news/an-introduction-to-q-learning-reinforcement-learning-14ac0b4493cc/.
[9]. Yufan Zhao, Michael R, Kosorok, Donglin Zeng: Reinforcement learning design for cancer clinical trials. Onlinelibrary, 2009. doi: https://onlinelibrary.wiley.com/doi/abs/10.1002/sim.3720.
[10]. Regina Padmanabhan, Nader Meskin, Wassim M. Haddad: Reinforcement learning-based control of drug dosing for cancer chemotherapy treatment. Sciencedirect, 2017. doi: https://www.sciencedirect.com/science/article/abs/pii/S0025556417304327.
[11]. Inkyung Ahn. Jooyoung Park: Drug scheduling of cancer chemotherapy based on natural actor-critic approach. Sciencedirect, 2011, pp.121-129. doi: https://www.sciencedirect.com/science/article/abs/pii/S0303264711001365.
Cite this article
Zhang,N. (2023). Analysis of reinforce learning in medical treatment. Applied and Computational Engineering,5,48-53.
Data availability
The datasets used and/or analyzed during the current study will be available from the authors upon reasonable request.
Disclaimer/Publisher's Note
The statements, opinions and data contained in all publications are solely those of the individual author(s) and contributor(s) and not of EWA Publishing and/or the editor(s). EWA Publishing and/or the editor(s) disclaim responsibility for any injury to people or property resulting from any ideas, methods, instructions or products referred to in the content.
About volume
Volume title: Proceedings of the 3rd International Conference on Signal Processing and Machine Learning
© 2024 by the author(s). Licensee EWA Publishing, Oxford, UK. This article is an open access article distributed under the terms and
conditions of the Creative Commons Attribution (CC BY) license. Authors who
publish this series agree to the following terms:
1. Authors retain copyright and grant the series right of first publication with the work simultaneously licensed under a Creative Commons
Attribution License that allows others to share the work with an acknowledgment of the work's authorship and initial publication in this
series.
2. Authors are able to enter into separate, additional contractual arrangements for the non-exclusive distribution of the series's published
version of the work (e.g., post it to an institutional repository or publish it in a book), with an acknowledgment of its initial
publication in this series.
3. Authors are permitted and encouraged to post their work online (e.g., in institutional repositories or on their website) prior to and
during the submission process, as it can lead to productive exchanges, as well as earlier and greater citation of published work (See
Open access policy for details).