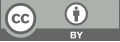
Comparison of Brain Tumor Segmentation Methods Based on Different Algorithms Using MRI Images
- 1 Glasgow College
* Author to whom correspondence should be addressed.
Abstract
Brain tumor is a serious disease for human beings. MRI is the most widely used method for its innocuousness since people do not need to be exposure to radioactivity. The segmentation on MRI images is a vital step in tumor detection. To improve efficiency and accuracy of the segmentation, scientists apply different algorithms in this process. This paper focuses on three particular algorithms including Connected component label algorithm (CCLA), Watershed algorithm (WSA) and Fuzzy C-means clustering algorithm (FCCA). The principles and applied procedures of these three algorithms are introduced. Basing on this background information, algorithms are compared from three aspects. Fuzzy C-means clustering algorithm is considered as more efficient and accurate among these three algorithms. All algorithms have good research prospects, and the segmentation result can be improved through the improvement on algorithms.
Keywords
connected component label algorithm, watershed algorithm, Fuzzy C-means (FCM) clustering algorithm, brain tumor segmentation, MRI images
[1]. P. Mimboro, A. Sunyoto and R. S. Kharisma, "Segmentation of Brain Tumor Objects in Magnetic Resonance Imaging (MRI) Image using Connected Component Label Algorithm," 2021 International Conference on Advanced Mechatronics, Intelligent Manufacture and Industrial Automation (ICAMIMIA), 2021, pp.195-198, doi: 10.1109/ICAMIMIA54022.2021.9807692.
[2]. Siying Technology, Brain tumor grading based on MRI medical images. Feb, 28, 2022. https://cloud.tencent.com/developer/article/1948068
[3]. T. A. Jemimma and Y. J. Vetharaj, "Watershed Algorithm based DAPP features for Brain Tumor Segmentation and Classification," 2018 International Conference on Smart Systems and Inventive Technology (ICSSIT), 2018, pp.155-158, doi: 10.1109/ICSSIT.2018.8748436.
[4]. L. Xuefen and M. Xiamin, “Research on the application of image segmentation in biomedical engineering,” China equipment engineering, 2022, no.12, pp.241-242. https://kns.cnki.net/kcms/detail/detail.aspx?FileName=SBGL202212100&DbName=CJFQ2 022
[5]. D. Yi, “Image segmentation technology based on mark watershed algorithm,” Computer knowledge and technology, 2022, no.18, pp.58-59, doi: 10.14004/j.cnki.ckt.2022.1571.
[6]. B. Srinivas and G. S. Rao, "Unsupervised learning algorithms for MRI brain tumor segmentation," 2018 Conference on Signal Processing And Communication Engineering Systems (SPACES), 2018, pp. 181-184, doi: 10.1109/SPACES.2018.8316341.
[7]. Z.Wengang and F.Fen, “Brain tumor image segmentation based on fast global fuzzy C-means clustering algorithm,” Journal of Jilin University (Science Edition), 2015, no.53, pp.494-498, doi: 10.13413/j.cnki.jdxblxb.2015.03.28.
[8]. Y. Jang, J. Mun, K. Oh and Jaeseok Kim, "Block-Based connected component labeling algorithm with block prediction," 2017 40th International Conference on Telecommunications and Signal Processing (TSP), 2017, pp.578-581, doi: 10.1109/TSP.2017.8076053.
[9]. R. Venkat and K. S. Reddy, "Dealing Big Data using Fuzzy C-Means (FCM) Clustering and Optimizing with Gravitational Search Algorithm (GSA)," 2019 3rd International Conference on Trends in Electronics and Informatics (ICOEI), 2019, pp.465-467, doi: 10.1109/ICOEI.2019.8862673.
[10]. T. Rahman and M. S. Islam, "Image Segmentation Based on Fuzzy C Means Clustering Algorithm and Morphological Reconstruction," 2021 International Conference on Information and Communication Technology for Sustainable Development (ICICT4SD), 2021, pp. 259-263, doi: 10.1109/ICICT4SD50815.2021.9396873.
Cite this article
Yong,J. (2023). Comparison of Brain Tumor Segmentation Methods Based on Different Algorithms Using MRI Images. Applied and Computational Engineering,8,13-17.
Data availability
The datasets used and/or analyzed during the current study will be available from the authors upon reasonable request.
Disclaimer/Publisher's Note
The statements, opinions and data contained in all publications are solely those of the individual author(s) and contributor(s) and not of EWA Publishing and/or the editor(s). EWA Publishing and/or the editor(s) disclaim responsibility for any injury to people or property resulting from any ideas, methods, instructions or products referred to in the content.
About volume
Volume title: Proceedings of the 2023 International Conference on Software Engineering and Machine Learning
© 2024 by the author(s). Licensee EWA Publishing, Oxford, UK. This article is an open access article distributed under the terms and
conditions of the Creative Commons Attribution (CC BY) license. Authors who
publish this series agree to the following terms:
1. Authors retain copyright and grant the series right of first publication with the work simultaneously licensed under a Creative Commons
Attribution License that allows others to share the work with an acknowledgment of the work's authorship and initial publication in this
series.
2. Authors are able to enter into separate, additional contractual arrangements for the non-exclusive distribution of the series's published
version of the work (e.g., post it to an institutional repository or publish it in a book), with an acknowledgment of its initial
publication in this series.
3. Authors are permitted and encouraged to post their work online (e.g., in institutional repositories or on their website) prior to and
during the submission process, as it can lead to productive exchanges, as well as earlier and greater citation of published work (See
Open access policy for details).