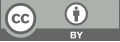
Machine learning anti-cheating algorithm and a test against computer vision aimbot
- 1 College of Engineering, School of Computing, University of Utah, Salt Lake City, 84112, USA
- 2 Champaign Central High School, Chapaign, 61822, USA
- 3 Crean Lutheran High School, Irvine, 92618, USA
- 4 Computer science, University of Bristol, Bristol, BS8 1QU, UK
* Author to whom correspondence should be addressed.
Abstract
In this article, we introduced a recurrent neural network (RNN) serves as a cheating detection method in CS: GO against traditional and AI cheating methods. Cheating methods today are growingly stronger, making traditional anti-cheating methods less and less helpful. The CV AimBot, which does not include any illegal operation but only reading the game footage, has made all traditional anti-cheating methods de facto useless. We developed a CV AimBot from scratch and used it to generate the sample data. We gathered datasets from traditional AimBot and newly invented CV AimBot and run it through an RNN. Our RNN shows high accuracy against both traditional and CV AimBot. It also shows that introducing data from CV AimBot with significantly lower the accuracy of the result from RNN.
Keywords
Machine Learning, Anti-Cheating, Aimbot, RNN.
[1]. Panicos Karkallis, Jorge Blasco, Guillermo Suarez-Tangil, Sergio Pastrana, Detection video-game injectors exchanged in game cheating communities, 2021
[2]. Nvidia Capture SDK. NVIDIA Developer. (2021, December 9). Retrieved September 24, 2022, from https://developer.nvidia.com/capture-sdk
[3]. Aditya Jonnalagadda, Luri Frosio, Seth Schneider, Morgan McGuire, Joohwan Kim, Robust Vision -Based Cheat Detection in Competitive Gaming, 2021
[4]. Harry Dunham, Cheat Detection using Machine Learning within Counter-Strike Global Offensive, 2020
[5]. Hochreiter, S., & Schmidhuber, J. (1997). Long short-term memory. Neural Computation, 9(8), 1735–1780. https://doi.org/10.1162/neco.1997.9.8.1735
[6]. Kingma, D. , & Ba, J. . (2014). Adam: a method for stochastic optimization. Computer Science.
[7]. Kanervisto, A. , Kinnunen, T. , & Hautamki, V. . (2022). Gan-aimbots: using machine learning for cheating in first person shooters.
[8]. Valve Anti-Cheat (VAC) system. Steam Support. (n.d.). Retrieved September 24, 2022, from https://help.steampowered.com/en/faqs/view/571A-97DA-70E9-FF74
[9]. Redmon, J., & Farhadi, A. (2018). Yolov3: An incremental improvement. arXiv preprint arXiv:1804.02767.
Cite this article
Yang,G.;Zhang,Y.;Liu,F.;Gao,Z. (2023). Machine learning anti-cheating algorithm and a test against computer vision aimbot. Applied and Computational Engineering,6,1048-1054.
Data availability
The datasets used and/or analyzed during the current study will be available from the authors upon reasonable request.
Disclaimer/Publisher's Note
The statements, opinions and data contained in all publications are solely those of the individual author(s) and contributor(s) and not of EWA Publishing and/or the editor(s). EWA Publishing and/or the editor(s) disclaim responsibility for any injury to people or property resulting from any ideas, methods, instructions or products referred to in the content.
About volume
Volume title: Proceedings of the 3rd International Conference on Signal Processing and Machine Learning
© 2024 by the author(s). Licensee EWA Publishing, Oxford, UK. This article is an open access article distributed under the terms and
conditions of the Creative Commons Attribution (CC BY) license. Authors who
publish this series agree to the following terms:
1. Authors retain copyright and grant the series right of first publication with the work simultaneously licensed under a Creative Commons
Attribution License that allows others to share the work with an acknowledgment of the work's authorship and initial publication in this
series.
2. Authors are able to enter into separate, additional contractual arrangements for the non-exclusive distribution of the series's published
version of the work (e.g., post it to an institutional repository or publish it in a book), with an acknowledgment of its initial
publication in this series.
3. Authors are permitted and encouraged to post their work online (e.g., in institutional repositories or on their website) prior to and
during the submission process, as it can lead to productive exchanges, as well as earlier and greater citation of published work (See
Open access policy for details).