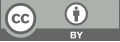
Face detection and recognition of mask wearing in normal environment based on neural network
- 1 School of Information Engineering Shanghai Maritime University, Shanghai, 201306, China
- 2 School of Physics, Xi’an JiaoTong University, Xian, Shaanxi, 710049, China
- 3 University of Liverpool, Liverpool L1 8JX, UK
- 4 epartment of International Baccalaureate Diploma Programme, Shanghai World For-eign Language Academy, Shanghai, 200233, China
* Author to whom correspondence should be addressed.
Abstract
With the outbreak of COVID-19, wearing masks has become a hot topic again. In public places, Not wearing a mask correctly has almost the same harm as not wearing a mask. Mask detection is an extension of face detection. Therefore, it is of great practical signifi-cance to design a system that can correctly identify whether pedestrians wear masks cor-rectly. The face recognition technology based on neural network has been relatively ma-ture, but there is still a lack of work related to mask recognition, especially whether to wear masks correctly. This is not only the promotion of a two-classification problem to a three-classification problem, but also faces many practical problems, including data set acquisition, in this paper, mask recognition is divided into a multi-stage work. In the first link, Yolo network is used to recognize facial areas, and in the second link, RESNET is used to realize mask recognition. Finally, a layer of RESNET network is improved to achieve higher recognition accuracy.
Keywords
COVID-19, face detection, mask detection, Yolo network, RESNET
[1]. Alex P,M Yan,Y T Huang,C Gao,W Z Li,(2021)What shapes people’s willingness to wear a face mask at the beginning of a public health disaster? A qualitative study based on COVID-19 in China. [J]International Journal of Disaster Risk ReductionVolume 65, 2021. PP 102577-102577
[2]. Chowdary G J, Punn N S, Sonbhadra S K, et al. (2020)Face mask detection using transfer learning of inceptionv3[C]//International Conference on Big Data Analytics. Springer, Cham, 2020: 81-90.
[3]. Ristea N C, Ionescu R T. (2006)Are you wearing a mask? Improving mask detection from speech using augmentation by cycle consistent GANs[J]. arXiv preprint arXiv:2006.10147, 2020
[4]. Nagrath P, Jain R, Madan A, et al. (2021) SSDMNV2: A real time DNN-based face mask detection system using single shot multibox detector and MobileNetV2[J]. Sustainable cities and society, 2021, 66: 102692.
[5]. Redmon J, Divvala S, Girshick R, et al. (2017) You Only Look Once: Unified, Real-Time Object Detection[J]. 2015.Redmon J, Farhadi A. YOLO9000: Better, Faster, Stronger[J]. 2017:6517- 6525.
[6]. Howard, A. G., Zhu, M., Chen, B., Kalenichenko, D., Wang, W., Weyand, T., ... Adam, H. (2017). Mobilenets: Efficient convolutional neural networks for mobile vision applications. arXiv preprint arXiv:1704.04861.
[7]. Redmon J, Farhadi A. (2018) YOLOv3: An Incremental Improvement[J]. arXiv e-prints, 2018.
[8]. He K , Zhang X , Ren S , et al.(2016) Deep Residual Learning for Image Recognition[C]// 2016 IEEE Conference on Computer Vision and Pattern Recognition (CVPR). IEEE, 2016.
[9]. Huang G, Liu Z, Laurens V, et al. (2016) Densely Connected Convolutional Networks[J]. IEEE Computer Society, 2016.
[10]. He K, Zhang X, Ren S, et al. (2014) Spatial Pyramid Pooling in Deep Convolutional Networks for Visual Recognition[J]. IEEE Transactions on Pattern Analysis Machine Intelligence, 2014, 37(9):1904-16.
[11]. Viola, P., Jones, M. (2001, December). Rapid object detection using a boosted cascade of simple features. In Proceedings of the 2001 IEEE computer society conference on computer vision and pattern recognition. CVPR 2001 (Vol. 1, pp. I-I). Ieee.
Cite this article
Li,Y.;Xie,S.;Ren,Y.;Li,X. (2023). Face detection and recognition of mask wearing in normal environment based on neural network. Applied and Computational Engineering,2,21-30.
Data availability
The datasets used and/or analyzed during the current study will be available from the authors upon reasonable request.
Disclaimer/Publisher's Note
The statements, opinions and data contained in all publications are solely those of the individual author(s) and contributor(s) and not of EWA Publishing and/or the editor(s). EWA Publishing and/or the editor(s) disclaim responsibility for any injury to people or property resulting from any ideas, methods, instructions or products referred to in the content.
About volume
Volume title: Proceedings of the 4th International Conference on Computing and Data Science (CONF-CDS 2022)
© 2024 by the author(s). Licensee EWA Publishing, Oxford, UK. This article is an open access article distributed under the terms and
conditions of the Creative Commons Attribution (CC BY) license. Authors who
publish this series agree to the following terms:
1. Authors retain copyright and grant the series right of first publication with the work simultaneously licensed under a Creative Commons
Attribution License that allows others to share the work with an acknowledgment of the work's authorship and initial publication in this
series.
2. Authors are able to enter into separate, additional contractual arrangements for the non-exclusive distribution of the series's published
version of the work (e.g., post it to an institutional repository or publish it in a book), with an acknowledgment of its initial
publication in this series.
3. Authors are permitted and encouraged to post their work online (e.g., in institutional repositories or on their website) prior to and
during the submission process, as it can lead to productive exchanges, as well as earlier and greater citation of published work (See
Open access policy for details).