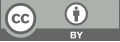
Scalp disease analysis using deep learning models
- 1 Department of CSE, Kongu Engineering College, Tamilnadu, India
- 2 Department of CSE, Kongu Engineering College, Tamilnadu, India
- 3 Department of CSE, Kongu Engineering College, Tamilnadu, India
- 4 Department of CSE, Kongu Engineering College, Tamilnadu, India
- 5 Department of CSE, Kongu Engineering College, Tamilnadu, India
* Author to whom correspondence should be addressed.
Abstract
Alopecia areata, folliculitis, hair loss, and dandruff are common scalp hair disorders caused by nutritional imbalances, stress, and pollution in the environment. Specialized treatments, such as hair physiotherapy, have arisen to address this issue. This paper pro-poses a deep learning model that can analysis the scalp diseases with high accuracy, using this model we can predict the scalp diseases which makes easier for them to treat the dis-ease with good treatment using the mobile phones we can predict the diseases simply us-ing its image. This paper deals with an advanced classification model which predicts scalp disease. More than 1100 images are used as training dataset, CNN is used for the classifi-cation and the high accuracy is achieved using VGG16, VGG19 and MobileNetV2.
Keywords
scalp, diseases, VGG16, VGG19, mobileNetV.
[1]. Esfandiari, A., Kalantari, K. R., & Babaei, A. (2012). Hair loss diagnosis using artificial neural networks. International Journal of Computer Science Issues (IJCSI), 9(5), 174.
[2]. Sathishkumar, V. E., & Cho, Y. (2019, December). Cardiovascular disease analysis and risk assessment using correlation based intelligent system. In Basic & clinical pharmacology & toxicology (Vol. 125, pp. 61-61). 111 RIVER ST, HOBOKEN 07030-5774, NJ USA: WILEY.
[3]. Krishnamoorthy, N., Asokan, R., & Jones, I. (2016). Classification of malignant and benign micro calcifications from mammogram using optimized cascading classifier. Current Signal Transduction Therapy, 11(2), 98-104.
[4]. Sathishkumar, V. E., Venkatesan, S., Park, J., Shin, C., Kim, Y., & Cho, Y. (2020, April). Nutrient water supply prediction for fruit production in greenhouse environment using artificial neural networks. In Basic & Clinical Pharmacology & Toxicology (Vol. 126, pp. 257-258). 111 RIVER ST, HOBOKEN 07030-5774, NJ USA: WILEY.
[5]. Lee, S. H., & Yang, C. S. (2018). An intelligent hair and scalp analysis system using camera sensors and Norwood-Hamilton model. International Journal of Innovative Computing Information and Control, 14(2), 503-518.
[6]. Antoniades, A., Spyrou, L., Martin-Lopez, D., Valentin, A., Alarcon, G., Sanei, S., & Took, C. C. (2018). Deep neural architectures for mapping scalp to intracranial EEG. International journal of neural systems, 28(08), 1850009.
[7]. D'Ovidio, R., Magri, F., Villa, L., Leoncini, P. P., Caro, G., Muscianese, M., & Rossi, A. (2022). Efficacy of a new combination of a microemulsion and oral supplementation for androgenetic alopecia: an interventional multicentric study. Italian Journal of Dermatology and Venereology.
[8]. Krishnamoorthy, N., Nirmaladevi, K., Kumaravel, T., Nithish, K. S., Sarathkumar, S., & Sarveshwaran, M. (2022, April). Diagnosis of Pneumonia Using Deep Learning Techniques. In 2022 Second International Conference on Advances in Electrical, Computing, Communication and Sustainable Technologies (ICAECT) (pp. 1-5). IEEE.
[9]. Shakeel, C. S., Khan, S. J., Chaudhry, B., Aijaz, S. F., & Hassan, U. (2021). Classification framework for healthy hairs and alopecia areata: a machine learning (ml) approach. Computational and mathematical methods in medicine, 2021.
[10]. Benhabiles, H., Hammoudi, K., Yang, Z., Windal, F., Melkemi, M., Dornaika, F., & Arganda-Carreras, I. (2019, November). Deep learning based detection of hair loss levels from facial images. In 2019 Ninth International Conference on Image Processing Theory, Tools and Applications (IPTA) (pp. 1-6). IEEE.
[11]. Devi, K. N., Krishnamoorthy, N., Jayanthi, P., Karthi, S., Karthik, T., & Kiranbharath, K. (2022, January). Machine Learning Based Adult Obesity Prediction. In 2022 International Conference on Computer Communication and Informatics (ICCCI) (pp. 1-5). IEEE.
[12]. Jhong, S. Y., Yang, P. Y., & Hsia, C. H. (2022). An Expert Smart Scalp Inspection System Using Deep Learning. Sensors and Materials, 34(4), 1265-1274.
[13]. Kotha, S. K., Rani, M. S., Subedi, B., Chunduru, A., Karrothu, A., Neupane, B., & Sathishkumar, V. E. (2021). A comprehensive review on secure data sharing in cloud environment. Wireless Personal Communications, 1-28.
[14]. Krishnamoorthy, N., Prasad, L. N., Kumar, C. P., Subedi, B., Abraha, H. B., & Sathishkumar, V. E. (2021). Rice leaf diseases prediction using deep neural networks with transfer learning. Environmental Research, 198, 111275.
[15]. Sathishkumar V E, Changsun Shin, Youngyun Cho, “Efficient energy consumption prediction model for a data analytic-enabled industry building in a smart city”, Building Research & Information, Vol. 49. no. 1, pp. 127-143, 2021.
[16]. Sathishkumar V E, Youngyun Cho, “A rule-based model for Seoul Bike sharing demand prediction using Weather data”, European Journal of Remote Sensing, Vol. 52, no. 1, pp. 166-183, 2020.
[17]. Sathishkumar V E, Jangwoo Park, Youngyun Cho, “Seoul Bike Trip duration prediction using data mining techniques”, IET Intelligent Transport Systems, Vol. 14, no. 11, pp. 1465-1474, 2020.
[18]. Sathishkumar V E, Jangwoo Park, Youngyun Cho, “Using data mining techniques for bike sharing demand prediction in Metropolitan city”, Computer Communications, Vol. 153, pp. 353-366, 2020.
[19]. Sathishkumar V E, Yongyun Cho, “Season wise bike sharing demand analysis using random forest algorithm”, Computational Intelligence, pp. 1-26, 2020.
[20]. Sathishkumar, V. E., Wesam Atef Hatamleh, Abeer Ali Alnuaim, Mohamed Abdelhady, B. Venkatesh, and S. Santhoshkumar. "Secure Dynamic Group Data Sharing in Semi-trusted Third Party Cloud Environment." Arabian Journal for Science and Engineering (2021): 1-9.
[21]. Chen, J., Shi, W., Wang, X., Pandian, S., & Sathishkumar, V. E. (2021). Workforce optimisation for improving customer experience in urban transportation using heuristic mathematical model. International Journal of Shipping and Transport Logistics, 13(5), 538-553.
[22]. Pavithra, E., Janakiramaiah, B., Narasimha Prasad, L. V., Deepa, D., Jayapandian, N., & Sathishkumar, V. E., Visiting Indian Hospitals Before, During and After Covid. International Journal of Uncertainty, Fuzziness and Knowledge-Based Systems, 30 (1), 111-123, 2022.
[23]. Easwaramoorthy, S., Moorthy, U., Kumar, C. A., Bhushan, S. B., & Sadagopan, V. (2017, January). Content based image retrieval with enhanced privacy in cloud using apache spark. In International Conference on Data Science Analytics and Applications (pp. 114-128). Springer, Singapore.
[24]. Sathishkumar, V. E., Agrawal, P., Park, J., & Cho, Y. (2020, April). Bike Sharing Demand Prediction Using Multiheaded Convolution Neural Networks. In Basic & Clinical Pharmacology & Toxicology (Vol. 126, pp. 264-265). 111 RIVER ST, HOBOKEN 07030-5774, NJ USA: WILEY.
[25]. Subramanian, M., Shanmuga Vadivel, K., Hatamleh, W. A., Alnuaim, A. A., Abdelhady, M., & VE, S. (2021). The role of contemporary digital tools and technologies in Covid‐19 crisis: An exploratory analysis. Expert systems.
[26]. Babu, J. C., Kumar, M. S., Jayagopal, P., Sathishkumar, V. E., Rajendran, S., Kumar, S., ... & Mahseena, A. M. (2022). IoT-Based Intelligent System for Internal Crack Detection in Building Blocks. Journal of Nanomaterials, 2022.
[27]. Subramanian, M., Kumar, M. S., Sathishkumar, V. E., Prabhu, J., Karthick, A., Ganesh, S. S., & Meem, M. A. (2022). Diagnosis of retinal diseases based on Bayesian optimization deep learning network using optical coherence tomography images. Computational Intelligence and Neuroscience, 2022.
[28]. Liu, Y., Sathishkumar, V. E., & Manickam, A. (2022). Augmented reality technology based on school physical education training. Computers and Electrical Engineering, 99, 107807.
[29]. Sathishkumar, V. E., Rahman, A. B. M., Park, J., Shin, C., & Cho, Y. (2020, April). Using machine learning algorithms for fruit disease classification. In Basic & clinical pharmacology & toxicology (Vol. 126, pp. 253-253). 111 RIVER ST, HOBOKEN 07030-5774, NJ USA: WILEY.
Cite this article
Krishnamoorthy,N.;Jayanthi,P.;Kumaravel,T.;Sundareshwar,V.A.;Harris,R.S.J. (2023). Scalp disease analysis using deep learning models. Applied and Computational Engineering,2,225-231.
Data availability
The datasets used and/or analyzed during the current study will be available from the authors upon reasonable request.
Disclaimer/Publisher's Note
The statements, opinions and data contained in all publications are solely those of the individual author(s) and contributor(s) and not of EWA Publishing and/or the editor(s). EWA Publishing and/or the editor(s) disclaim responsibility for any injury to people or property resulting from any ideas, methods, instructions or products referred to in the content.
About volume
Volume title: Proceedings of the 4th International Conference on Computing and Data Science (CONF-CDS 2022)
© 2024 by the author(s). Licensee EWA Publishing, Oxford, UK. This article is an open access article distributed under the terms and
conditions of the Creative Commons Attribution (CC BY) license. Authors who
publish this series agree to the following terms:
1. Authors retain copyright and grant the series right of first publication with the work simultaneously licensed under a Creative Commons
Attribution License that allows others to share the work with an acknowledgment of the work's authorship and initial publication in this
series.
2. Authors are able to enter into separate, additional contractual arrangements for the non-exclusive distribution of the series's published
version of the work (e.g., post it to an institutional repository or publish it in a book), with an acknowledgment of its initial
publication in this series.
3. Authors are permitted and encouraged to post their work online (e.g., in institutional repositories or on their website) prior to and
during the submission process, as it can lead to productive exchanges, as well as earlier and greater citation of published work (See
Open access policy for details).