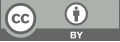
Fruit 360 classification based on the convolutional neural network
- 1 Rutgers university
* Author to whom correspondence should be addressed.
Abstract
This research paper focuses on the Fruit360 Classification challenge, a task aimed at developing a fruit classification model capable of accurately identifying various fruits and distinguishing them from each other. In this study, the Fruit360 dataset is used, consisting of 90380 images of 131 fruits and vegetable classes. Prior to training the CNN model, the images are preprocessed by resizing, normalizing, and augmenting them. The authors employ a pre-trained CNN model called ResNet-50 using the PyTorch deep learning framework and add a custom fully connected layer on top to adapt the model to the specific classification task. The authors conclude that the proposed model achieved excellent performance on the Fruit360 dataset. The study highlights the importance of the Fruit360 Classification challenge in advancing the field of computer vision, specifically in the development of deep learning algorithms for image classification tasks. The proposed model has the potential to improve the efficiency and accuracy of fruit classification, which can benefit the fruit industry in terms of enhanced productivity and cost-effectiveness.
Keywords
fruit classification, convolutional neural network, deep learning
[1]. Zhang Z Zhang Y and Li J 2020 A novel deep learning framework for fruit recognition based on improved convolutional neural networks IEEE Access vol 8 pp 2308-2321
[2]. Chen Y Chen H and Wu Y 2021 Fruit recognition using deep transfer learning in Proc 2021 4th Int Conf on Intelligent and Innovative Computing Applications (ICIIA) p 86-89
[3]. Mureşan H and Oltean M 2018 Fruit recognition from images using deep learning Acta Universitatis Sapientiae Informatica 10(1) 26-42
[4]. Pillow S 2020 Python Imaging Library Handbook Retrieved from https://pillow.readthedocs.io/en/stable/handbook/index.html
[5]. LeCun Y Bengio Y and Hinton G 2015 Deep learning Nature 521(7553) 436-444
[6]. He K Zhang X Ren S & Sun J 2016 Deep residual learning for image recognition In Proceedings of the IEEE conference on computer vision and pattern recognition (p 770-778)
[7]. Yu Q Chang C S Yan J L et al. 2019 Semantic segmentation of intracranial hemorrhages in head CT scans 2019 IEEE 10th International Conference on Software Engineering and Service Science (ICSESS) IEEE 112-115
[8]. Li B, Lima D 2021 Facial expression recognition via ResNet-50 International Journal of Cognitive Computing in Engineering 2: 57-64
[9]. Martinez M Stiefelhagen R 2019 Taming the cross entropy loss Pattern Recognition: 40th German Conference GCPR 2018 Stuttgart, Germany October 9-12 2018 Proceedings 40 Springer International Publishing 628-637
[10]. Pang T Xu K Dong Y et al. 2019 Rethinking softmax cross-entropy loss for adversarial robustness arXiv preprint arXiv:1905.10626
Cite this article
Zhang,D. (2023). Fruit 360 classification based on the convolutional neural network. Applied and Computational Engineering,15,219-222.
Data availability
The datasets used and/or analyzed during the current study will be available from the authors upon reasonable request.
Disclaimer/Publisher's Note
The statements, opinions and data contained in all publications are solely those of the individual author(s) and contributor(s) and not of EWA Publishing and/or the editor(s). EWA Publishing and/or the editor(s) disclaim responsibility for any injury to people or property resulting from any ideas, methods, instructions or products referred to in the content.
About volume
Volume title: Proceedings of the 5th International Conference on Computing and Data Science
© 2024 by the author(s). Licensee EWA Publishing, Oxford, UK. This article is an open access article distributed under the terms and
conditions of the Creative Commons Attribution (CC BY) license. Authors who
publish this series agree to the following terms:
1. Authors retain copyright and grant the series right of first publication with the work simultaneously licensed under a Creative Commons
Attribution License that allows others to share the work with an acknowledgment of the work's authorship and initial publication in this
series.
2. Authors are able to enter into separate, additional contractual arrangements for the non-exclusive distribution of the series's published
version of the work (e.g., post it to an institutional repository or publish it in a book), with an acknowledgment of its initial
publication in this series.
3. Authors are permitted and encouraged to post their work online (e.g., in institutional repositories or on their website) prior to and
during the submission process, as it can lead to productive exchanges, as well as earlier and greater citation of published work (See
Open access policy for details).