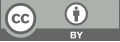
Edge Impulse-based automated machine learning for comedy performance evaluation
- 1 Beijing University of Posts and Telecommunications
* Author to whom correspondence should be addressed.
Abstract
The evaluation of comedic performances presents a challenge due to the subjectivity of humor and various performance factors. This paper proposes a potential solution to this problem by utilizing artificial intelligence (AI), particularly automated machine learning (AutoML), to develop an automatic comedy show scoring system using a diverse Chinese acoustic dataset and the Edge Impulse platform. The proposed method involves analyzing complete video recordings of performances by the renowned Chinese comedy troupe, Deyunshe, to assess audience responses and critical acoustic elements that contribute to a comedic performance's success. The study collected five comedic clips, totaling approximately 50 minutes, segmented and labeled them for training purposes. This study systematically explored various configurations using Edge Impulse to optimize accuracy and minimize loss, yielding encouraging results that demonstrate the potential of AI-driven scoring systems. The study identifies several avenues for future research, including enhancing the quality and quantity of training data, refining classification algorithms, and exploring alternative machine learning techniques to further improve accuracy and loss rates. Moreover, the research highlights the potential benefits of integrating real-time scoring systems, which could facilitate audience engagement and refining comedic material. This innovative approach demonstrates the potential to develop a more sophisticated, accurate, and reliable method of evaluating comedic performances, offering valuable insights into audience preferences, enabling the production of high-quality comedic content, and fostering a more objective evaluation of talent in the competitive world of comedy, which could significantly benefit the entertainment industry.
Keywords
comedy quality evaluation, machine learning, AutoDL
[1]. Hovy E andLavid J 2010 Towards a ‘science’ of corpus annotation: A new methodological challenge for corpus linguistics International Journal of Translation 22(1) 13-36
[2]. Krizhevsky A Sutskever I and Hinton G E 2012 ImageNet classification with deep convolutional neural networks In Advances in neural information processing systems pp. 1097-1105
[3]. Pang B Lee L 2008 Opinion mining and sentiment analysis. Foundations and Trends® in Information Retrieval 2(1-2) 1-135
[4]. Bostrom N Yudkowsky E 2014 The ethics of artificial intelligence. In Cambridge handbook of artificial intelligence pp. 316-334Cambridge University Press
[5]. Bishop C M 2006 Pattern Recognition and Machine Learning Springer
[6]. YuQ Chang C S Yan J L et al. 2019 Semantic segmentation of intracranial hemorrhages in head CT scans2019 IEEE 10th International Conference on Software Engineering and Service Science (ICSESS) IEEE 112-115.
[7]. Dhiman G Juneja S Viriyasitavat W et al. 2022 A novel machine-learning-based hybrid CNN model for tumor identification in medical image processing Sustainability 14(3): 1447
[8]. Jiang F et al2017 Artificial intelligence in healthcare: past, present and future Stroke and Vascular Neurology 2(4) 230-243
[9]. Bojarski M et al 2016 End to end learning for self-driving cars arXiv preprint arXiv:1604.07316
[10]. KamilarisA andPrenafeta-Boldύ F X 2018 Deep learning in agriculture: A survey Computers and Electronics in Agriculture 147 70-90
[11]. Hutter F et al 2019 Automated Machine Learning: Methods, Systems, Challenges Springer
[12]. Zöller M A and Huber M F 2020 Survey on automated machine learning Journal of Artificial Intelligence Research 69 421-441
Cite this article
Xing,S. (2023). Edge Impulse-based automated machine learning for comedy performance evaluation. Applied and Computational Engineering,18,152-156.
Data availability
The datasets used and/or analyzed during the current study will be available from the authors upon reasonable request.
Disclaimer/Publisher's Note
The statements, opinions and data contained in all publications are solely those of the individual author(s) and contributor(s) and not of EWA Publishing and/or the editor(s). EWA Publishing and/or the editor(s) disclaim responsibility for any injury to people or property resulting from any ideas, methods, instructions or products referred to in the content.
About volume
Volume title: Proceedings of the 5th International Conference on Computing and Data Science
© 2024 by the author(s). Licensee EWA Publishing, Oxford, UK. This article is an open access article distributed under the terms and
conditions of the Creative Commons Attribution (CC BY) license. Authors who
publish this series agree to the following terms:
1. Authors retain copyright and grant the series right of first publication with the work simultaneously licensed under a Creative Commons
Attribution License that allows others to share the work with an acknowledgment of the work's authorship and initial publication in this
series.
2. Authors are able to enter into separate, additional contractual arrangements for the non-exclusive distribution of the series's published
version of the work (e.g., post it to an institutional repository or publish it in a book), with an acknowledgment of its initial
publication in this series.
3. Authors are permitted and encouraged to post their work online (e.g., in institutional repositories or on their website) prior to and
during the submission process, as it can lead to productive exchanges, as well as earlier and greater citation of published work (See
Open access policy for details).