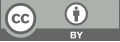
Estimating diabetes risk using Naïve Bayes classifiers
- 1 Northeastern University
* Author to whom correspondence should be addressed.
Abstract
Diabetes is now a common disease for modern people. Diabetes will cause some serious symptoms. Diabetes patients will face a painful life, high cure cost, and even death. So, it is necessary to correctly diagnose diabetes and analyze the factors that mainly cause diabetes to prevent the happen of diabetes. This essay mainly focuses on training the computer to help the doctor to train the computer. Three Naive Bayes classifications will be used to train the computer to do the prediction, including the Gaussian Naïve Bayes, Bernoulli Naïve Bayes, and Multinomial Naïve Bayes. To compare each method’s result, accuracy will be the main index to measure whether a method is good enough to put into use. Not only the accuracy, classification report, and confusion matrix also assist to measure the prediction. Finally, the Gaussian Naïve Bayes has the highest accuracy and when combined with the confusion matrix and the classification report, the Gaussian Naïve Bayes has a huge advantage over the other two models. The accuracy of these models still does not satisfy medical demand. Some deep learning and high-level model are expected to optimize this project.
Keywords
diabetes, machine learning, Naïve Bayes, classification
[1]. Forouhi, N. G., & Wareham, N. J. (2010). Epidemiology of diabetes. Medicine, 38(11), 602-606.
[2]. Tao, Z., Shi, A., & Zhao, J. (2015). Epidemiological perspectives of diabetes. Cell biochemistry and biophysics, 73(1), 181-185.
[3]. Leahy, J. L. (2005). Pathogenesis of type 2 diabetes mellitus. Archives of medical research, 36(3), 197-209.
[4]. Nathan, D. M. (2015). Diabetes: advances in diagnosis and treatment. Jama, 314(10), 1052-1062.
[5]. Faiz, I., Mukhtar, H., & Khan, S. (2014). An integrated approach of diet and exercise recommendations for diabetes patients. In 2014 IEEE 16th International Conference on e-Health Networking, Applications and Services, 537-542.
[6]. Emerging Risk Factors Collaboration. (2011). Diabetes mellitus, fasting glucose, and risk of cause-specific death. New England Journal of Medicine, 364(9), 829-841.
[7]. Webb, G. I., Keogh, E., & Miikkulainen, R. (2010). Naïve Bayes. Encyclopedia of machine learning, 15, 713-714.
[8]. Mehmet, A., (2020). Diabetes Dataset. URL: https://www.kaggle.com/datasets/mathchi/diabetes-data-set
[9]. Perez, A., Larranaga, P., & Inza, I. (2006). Supervised classification with conditional Gaussian networks: Increasing the structure complexity from naive Bayes. International Journal of Approximate Reasoning, 43(1), 1-25.
[10]. Artur, M. (2021). Review the performance of the Bernoulli Naïve Bayes Classifier in Intrusion Detection Systems using Recursive Feature Elimination with Cross-validated selection of the best number of features. Procedia Computer Science, 190, 564-570.
[11]. Jiang, L., Wang, S., Li, C., & Zhang, L. (2016). Structure extended multinomial naive Bayes. Information Sciences, 329, 346-356.
Cite this article
Li,J. (2023). Estimating diabetes risk using Naïve Bayes classifiers. Applied and Computational Engineering,21,28-35.
Data availability
The datasets used and/or analyzed during the current study will be available from the authors upon reasonable request.
Disclaimer/Publisher's Note
The statements, opinions and data contained in all publications are solely those of the individual author(s) and contributor(s) and not of EWA Publishing and/or the editor(s). EWA Publishing and/or the editor(s) disclaim responsibility for any injury to people or property resulting from any ideas, methods, instructions or products referred to in the content.
About volume
Volume title: Proceedings of the 5th International Conference on Computing and Data Science
© 2024 by the author(s). Licensee EWA Publishing, Oxford, UK. This article is an open access article distributed under the terms and
conditions of the Creative Commons Attribution (CC BY) license. Authors who
publish this series agree to the following terms:
1. Authors retain copyright and grant the series right of first publication with the work simultaneously licensed under a Creative Commons
Attribution License that allows others to share the work with an acknowledgment of the work's authorship and initial publication in this
series.
2. Authors are able to enter into separate, additional contractual arrangements for the non-exclusive distribution of the series's published
version of the work (e.g., post it to an institutional repository or publish it in a book), with an acknowledgment of its initial
publication in this series.
3. Authors are permitted and encouraged to post their work online (e.g., in institutional repositories or on their website) prior to and
during the submission process, as it can lead to productive exchanges, as well as earlier and greater citation of published work (See
Open access policy for details).