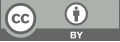
FaceTell: A novel solution to diagnosing Parkinson’s disease with facial expressions and deep learning
- 1 Palo Alto High School
- 2 University of California
* Author to whom correspondence should be addressed.
Abstract
Parkinson’s disease (PD) is a neurodegenerative disease afflicting over 10 million patients worldwide, most commonly the elderly, that causes tremors, stiffness, movement loss, and other symptoms. Since symptoms are often mild and difficult to notice in the early stages of the condition, it can be hard to notice and diagnose until the condition has already become more severe. An earlier diagnosis of PD will allow treatment to begin earlier and lessen the impact of the disease. The goal of this work is to develop an affordable, non-intrusive, and accessible way of diagnosing PD. This neurodegenerative disorder leads to loss of movement control and other symptoms. Since there is no known cure for PD yet, early diagnosis would allow timely treatment and prevent the symptoms from worsening too quickly. Doing so in an affordable and non-intrusive way will minimize costs and maximize efficiency — removing the need for lengthy consulting with doctors and possibly expensive testing and medical equipment. This work presents the FaceTell system, which combines and optimizes traditional machine learning and deep learning to make predictions on the patient’s PD status based on video data of their faces. By analyzing a variety of attributes such as facial expressions and emotion prevalence/intensity, the model was able to achieve a more thorough examination of the patient’s condition and make predictions of similar accuracy compared to prior results. One main innovation was collecting data affordably: sampling publicly available videos from platforms like YouTube. This serves as a proof-of-concept to show that simple, affordable, and non-intrusive data collection methods can still produce viable results. Using methods and tools such as hyperparameter tuning, data cleaning, and Face++, the performance of the system readily improved. The ultimate results obtained include an F1 score of 0.86 and an accuracy of 89%, compared to prior results which reached up to 95% accuracy.
Keywords
Parkinson’s disease, early diagnosis, machine learning, facial expressions
[1]. Parkinson’s Disease: Challenges, Progress, and Promise. (2023, January 30). National Institute of Neurological Disorders and Stroke. https://www.ninds.nih.gov/current-research/focus-disorders/focus-parkinsons-disease-research/parkinsons-disease-challenges-progress-and-promise
[2]. Mayo Clinic Staff. (2023, May 26). Parkinson’s disease. Mayo Clinic. https://www.mayoclinic.org/diseases-conditions/parkinsons-disease/
[3]. National Health Service. (2022, November 11). Parkinson’s disease. The NHS Website. https://www.nhs.uk/conditions/parkinsons-disease/
[4]. Yang, W., Hamilton, J. L., Kopil, C., Beck, J. C., Tanner, C. M., Albin, R. L., ... & Thompson, T. (2020). Current and projected future economic burden of Parkinson’s disease in the US. npj Parkinson's Disease, 6(1), 1-9.
[5]. Jin, B., Qu, Y., Zhang, L., & Gao, Z. (2020). Diagnosing Parkinson Disease Through Facial Expression Recognition: Video Analysis. Journal of medical Internet research, 22(7), e18697. https://doi.org/10.2196/18697
[6]. Ali, M. R., Myers, T., Wagner, E., Ratnu, H., Dorsey, E., & Hoque, E. (2021). Facial expressions can detect Parkinson’s disease: Preliminary evidence from videos collected online. NPJ digital medicine, 4(1), 1-4.
[7]. Hou, X., Zhang, Y., Wang, Y., Wang, X., Zhao, J., Zhu, X., & Su, J. (2021). A Markerless 2D Video, Facial Feature Recognition–Based, Artificial Intelligence Model to Assist With Screening for Parkinson Disease: Development and Usability Study. Journal of Medical Internet Research, 23(11), e29554.
[8]. Yang, L., Chen, X., Guo, Q., Zhang, J., Luo, M., Chen, X., ... & Xu, F. (2022). Changes in facial expressions in patients with Parkinson's disease during the phonation test and their correlation with disease severity. Computer Speech & Language, 72, 10
[9]. Pegolo, E., Volpe, D., Cucca, A., Ricciardi, L., & Sawacha, Z. (2022). Quantitative Evaluation of Hypomimia in Parkinson's Disease: A Face Tracking Approach. Sensors (Basel, Switzerland), 22(4), 1358. https://doi.org/10.3390/s22041358
[10]. U.S. Copyright Office Fair Use Index. (2023, February). U.S. Copyright Office. https://www.copyright.gov/fair-use/
[11]. Megvii. (n.d.). Face Detection. Face++ Cognitive Services. Retrieved April 21, 2023, from https://www.faceplusplus.com/face-detection/
[12]. Scikit-learn: Machine Learning in Python, Pedregosa, F. et al., JMLR 12, pp. 2825-2830, 2011.
[13]. Banoula, M. (2023, May 29). An Overview on Multilayer Perceptron (MLP). Simplilearn. https://www.simplilearn.com/tutorials/deep- learning-tutorial/multilayer-perceptron
[14]. Korstanje, J. (2021, August 31). The F1 score. Towards Data Science. https://towardsdatascience.com/the-f1-score-bec2bbc38aa6
[15]. Hajian-Tilaki, K. (2013). Receiver Operating Characteristic (ROC) Curve Analysis for Medical Diagnostic Test Evaluation. Caspian journal of internal medicine, 4(2), 627–635.
Cite this article
Shi,H.;Wu,B. (2023). FaceTell: A novel solution to diagnosing Parkinson’s disease with facial expressions and deep learning. Applied and Computational Engineering,21,53-62.
Data availability
The datasets used and/or analyzed during the current study will be available from the authors upon reasonable request.
Disclaimer/Publisher's Note
The statements, opinions and data contained in all publications are solely those of the individual author(s) and contributor(s) and not of EWA Publishing and/or the editor(s). EWA Publishing and/or the editor(s) disclaim responsibility for any injury to people or property resulting from any ideas, methods, instructions or products referred to in the content.
About volume
Volume title: Proceedings of the 5th International Conference on Computing and Data Science
© 2024 by the author(s). Licensee EWA Publishing, Oxford, UK. This article is an open access article distributed under the terms and
conditions of the Creative Commons Attribution (CC BY) license. Authors who
publish this series agree to the following terms:
1. Authors retain copyright and grant the series right of first publication with the work simultaneously licensed under a Creative Commons
Attribution License that allows others to share the work with an acknowledgment of the work's authorship and initial publication in this
series.
2. Authors are able to enter into separate, additional contractual arrangements for the non-exclusive distribution of the series's published
version of the work (e.g., post it to an institutional repository or publish it in a book), with an acknowledgment of its initial
publication in this series.
3. Authors are permitted and encouraged to post their work online (e.g., in institutional repositories or on their website) prior to and
during the submission process, as it can lead to productive exchanges, as well as earlier and greater citation of published work (See
Open access policy for details).