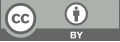
Comparison between transformer, informer, autoformer and non-stationary transformer in financial market
- 1 Shanghai University of International Business and Economics
* Author to whom correspondence should be addressed.
Abstract
This paper delves into the significance of predicting stock prices and carries out comparative experiments using a variety of models, including Support Vector Regression, Long Short-Term Memory model, Transformer, Informer, Autoformer, and Non-Stationary Transformer. These models are used to train and forecast the China Securities Index, Hang Seng Index, and S&P 500 Index. The results of the experiments are measured using indicators such as Mean Absolute Error and Root Mean Square Error. The findings show that the Non-Stationary Transformer model has the highest prediction accuracy. Additionally, a simple trading strategy is designed for each model and their Sharpe and Calmar ratios are compared. Since Autoformer has the highest Sharpe and Calmar, it can be concluded that Autoformer is the most practical in financial market among the four models. This research contributes to the field of stock price prediction by providing an empirical study on the application of Transformer and its derivative models which have been less explored in this domain. In conclusion, this paper offers valuable insights and recommendations for data scientists and financial engineer and introduces new methods for predicting stock prices.
Keywords
transformer, informer, autoformer, non-sationary transformer, stock price prediction
[1]. G. Box and G. Jenkins, “Models for Forecasting Seasonal and Non-seasonal Time Series,” Defense Technical Information Center (DTIC), AD0656685, 1967. DOI:10.1016/S0167-5648(08)70667-1.
[2]. A. A. Ariyo, A. O. Adewumi and C. K. Ayo, “Stock Price Prediction Using the ARIMA Model,” in 2014 UKSim-AMSS 16th International Conference on Computer Modelling and Simulation, pp. 106-112, 2014. DOI: 10.1109/UKSim.2014.67.
[3]. B. Henrique, V. Sobreiro and H. Kimura, “Stock price prediction using support vector regression on daily and up to the minute prices,” The Journal of Finance and Data Science, vol. 4, no. 3, pp. 183-201, 2018. DOI: 10.1016/j.jfds.2018.04.003.
[4]. J. Liu, X. He, J. Gao and J. Han, “Stock price prediction using LSTM, RNN and CNN sl,” in ICACCI, pp. 230-234, 2017. DOI: 10.1109/ICACCI.2017.8126078.
[5]. E. Hoseinzade and S. Haratizadeh, “CNNpred: CNN based stock market prediction using a diverse set of variables,” Expert Systems with Applications, vol. 129, pp. 273-285, 2019. DOI: 10.1016/j.eswa.2019.03.029.
[6]. A.Vaswani et al., “Attention is all you need,” in Advances in Neural Information Processing Systems 30 (NIPS), I.Guyon et al., Eds., pp.5998–6008, 2017. DOI: 10.48550/arXiv:1706:03762.
[7]. H.Y.Zhou et al., “Informer: Beyond Efficient Transformer for Long Sequence Time-Series Forecasting,” arXiv preprint arXiv:2012:07436, 2021. DOI:10:48550/arXiv:2012:07436.
[8]. M.Chen et al., “AutoFormer: Searching Transformers for Visual Recognition,” arXiv preprint arXiv:2107:00651, 2021. DOI: 10:48550/arXiv:2107:00651.
[9]. Z.Wang et al., “Non-stationary Transformers: Exploring the Stationarity in Time Series Forecasting,” arXiv preprint arXiv:2205:14415, 2022. DOI: 10:48550/arXiv:2205:14415.
[10]. D.P.Kingma and J.Ba., “Adam:A method for stochastic optimization,” in Proceedings of the 3rd International Conference for Learning Representations(ICLR), pp1-15, 2015. DOI: 10:48550/arXiv1412:6980.
Cite this article
Wu,Y. (2023). Comparison between transformer, informer, autoformer and non-stationary transformer in financial market. Applied and Computational Engineering,29,68-78.
Data availability
The datasets used and/or analyzed during the current study will be available from the authors upon reasonable request.
Disclaimer/Publisher's Note
The statements, opinions and data contained in all publications are solely those of the individual author(s) and contributor(s) and not of EWA Publishing and/or the editor(s). EWA Publishing and/or the editor(s) disclaim responsibility for any injury to people or property resulting from any ideas, methods, instructions or products referred to in the content.
About volume
Volume title: Proceedings of the 5th International Conference on Computing and Data Science
© 2024 by the author(s). Licensee EWA Publishing, Oxford, UK. This article is an open access article distributed under the terms and
conditions of the Creative Commons Attribution (CC BY) license. Authors who
publish this series agree to the following terms:
1. Authors retain copyright and grant the series right of first publication with the work simultaneously licensed under a Creative Commons
Attribution License that allows others to share the work with an acknowledgment of the work's authorship and initial publication in this
series.
2. Authors are able to enter into separate, additional contractual arrangements for the non-exclusive distribution of the series's published
version of the work (e.g., post it to an institutional repository or publish it in a book), with an acknowledgment of its initial
publication in this series.
3. Authors are permitted and encouraged to post their work online (e.g., in institutional repositories or on their website) prior to and
during the submission process, as it can lead to productive exchanges, as well as earlier and greater citation of published work (See
Open access policy for details).