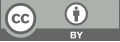
Exploration and evaluation of faster R-CNN-based pedestrian detection techniques
- 1 Institute of Artificial Intelligence and Robotics, Xi’an Jiaotong University, Xi’an, 710049, China
* Author to whom correspondence should be addressed.
Abstract
Presented herein is an exploration into the efficacy of a pedestrian detection model that capitalizes on the Faster region-based convolutional neural network (R-CNN) algorithm. This model, following its training phase on the Caltech Pedestrian dataset, underwent a meticulous evaluation process designed to gauge its proficiency in pedestrian detection tasks. The Average Precision (AP) achieved during testing was an impressive 51.9%, pointing to a high degree of accuracy. In addition to its commendable accuracy, this model demonstrates a remarkable speed of inference. Each image was processed in a mere 0.07 seconds, underlining the model's potential for real-time pedestrian detection in real-world scenarios. Further enhancing its potential for deployment is the model's relatively compact storage footprint, consuming only 158MB of storage space. By providing an in-depth analysis of this Faster R-CNN-based pedestrian detection model, the study offers valuable insights for future developments in the computer vision field, particularly for real-world applications. This paper thus contributes to our understanding of the applicability and effectiveness of the Faster R-CNN algorithm, providing a solid foundation for future research and development.
Keywords
pedestrian detection, faster R-CNN, Caltech Pedestrian
[1]. Liu, S., Huang, D., Wang, C., & Wang, X. (2020). High-level Semantic Feature Detection: A New Perspective for Pedestrian Detection. arXiv preprint arXiv:2005.13662.
[2]. Ren, S., He, K., Girshick, R., & Sun, J. (2015). Faster R-CNN: Towards Real-Time Object Detection with Region Proposal Networks. In Advances in Neural Information Processing Systems (pp. 91-99).
[3]. Dalal, N., & Triggs, B. (2005). Histograms of oriented gradients for human detection. In Proceedings of the IEEE conference on computer vision and pattern recognition (pp. 886-893).
[4]. Zhang, S., Wen, L., Bian, X., Lei, Z., & Li, S. Z. (2018). Single-shot refinement neural network for object detection. In Proceedings of the IEEE conference on computer vision and pattern recognition (pp. 4203-4212).
[5]. Zhu, Y., Chen, C., & Lu, H. (2019). Bi-box regression for pedestrian detection and occlusion estimation. In Proceedings of the IEEE Conference on Computer Vision and Pattern Recognition (pp. 3674-3683).
[6]. Liu T, Stathaki T. Faster R-CNN for robust pedestrian detection using semantic segmentation network[J]. Frontiers in neurorobotics, 2018, 12: 64.
[7]. Dai X, Hu J, Zhang H, et al. Multi-task faster R-CNN for nighttime pedestrian detection and distance estimation[J]. Infrared Physics & Technology, 2021, 115: 103694.
[8]. Yu W, Kim S, Chen F, et al. Pedestrian Detection Based on Improved Mask R-CNN Algorithm[C]//Intelligent and Fuzzy Techniques: Smart and Innovative Solutions: Proceedings of the INFUS 2020 Conference, Istanbul, Turkey, July 21-23, 2020. Springer International Publishing, 2021: 1515-1522.
[9]. Zhai S, Dong S, Shang D, et al. An improved faster R-CNN pedestrian detection algorithm based on feature fusion and context analysis[J]. IEEE Access, 2020, 8: 138117-138128.
[10]. Zhao Z, Ma J, Ma C, et al. An improved faster R-CNN algorithm for pedestrian detection[C]//2021 11th international conference on information technology in medicine and education (ITME). IEEE, 2021: 76-80.
Cite this article
Gao,S. (2024). Exploration and evaluation of faster R-CNN-based pedestrian detection techniques. Applied and Computational Engineering,32,185-190.
Data availability
The datasets used and/or analyzed during the current study will be available from the authors upon reasonable request.
Disclaimer/Publisher's Note
The statements, opinions and data contained in all publications are solely those of the individual author(s) and contributor(s) and not of EWA Publishing and/or the editor(s). EWA Publishing and/or the editor(s) disclaim responsibility for any injury to people or property resulting from any ideas, methods, instructions or products referred to in the content.
About volume
Volume title: Proceedings of the 2023 International Conference on Machine Learning and Automation
© 2024 by the author(s). Licensee EWA Publishing, Oxford, UK. This article is an open access article distributed under the terms and
conditions of the Creative Commons Attribution (CC BY) license. Authors who
publish this series agree to the following terms:
1. Authors retain copyright and grant the series right of first publication with the work simultaneously licensed under a Creative Commons
Attribution License that allows others to share the work with an acknowledgment of the work's authorship and initial publication in this
series.
2. Authors are able to enter into separate, additional contractual arrangements for the non-exclusive distribution of the series's published
version of the work (e.g., post it to an institutional repository or publish it in a book), with an acknowledgment of its initial
publication in this series.
3. Authors are permitted and encouraged to post their work online (e.g., in institutional repositories or on their website) prior to and
during the submission process, as it can lead to productive exchanges, as well as earlier and greater citation of published work (See
Open access policy for details).