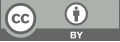
Local highlight and shadow adaptively repairing GAN for illumination-robust makeup transfer
- 1 Beijing University of Posts and Telecommunications
* Author to whom correspondence should be addressed.
Abstract
Recently, makeup transfer task has been widely explored with the development of deep learning. However, existing methods have shortcomings in more complex lighting situations in the real world because they do not consider the interference of lighting factors on facial features. To solve the above problem, we propose a local highlight and shadow adaptively repairing GAN for illumination-robust makeup transfer. We first map the 2D face images to UV representations and perform makeup transfer in the UV texture space, which explicitly removes the spatial misalignment to achieve pose and expression invariant makeup transfer. Furthermore, we take advantage of the face symmetry in the UV texture space to design an illumination repair module. It can adaptively repair the features affected by asymmetric local highlight and shadow based on a process of flipping and multi-layer attention fusion. In addition, the multi-layer attention maps are obtained by a pre-trained illumination classification network and hence have the ability to indicate local highlight and shadow areas. Comprehensive experiment results demonstrate the consistent effectiveness and clear advantages of our method, which significantly improve the robustness against local light effects and generate natural transfer results.
Keywords
facial makeup transfer, deep learning, generative adversarial network
[1]. Goodfellow I, Pouget-Abadie J, Mirza M, Xu B, Warde-Farley D, Ozair S, Courville A and Bengio Y 2014 Generative adversarial nets Advances in Neural Information Processing Systems pp 2672-2680
[2]. Tong W S, Tang C K, Brown M S and Xu Y Q 2007 Example-based cosmetic transfer 15th Pacific Conf. on Computer Graphics and Applications pp 211-218
[3]. Guo D and Sim T 2009 Digital face makeup by example Proc. of the IEEE/CVF Conf. on Computer Vision and Pattern Recognition pp 73-79
[4]. Liu S, Ou X, Qian R, Wang W, and Cao X 2016 Makeup like a superstar: deep localized makeup transfer network Proc. of the 25th Int. Joint Conf. on Artificial Intelligence pp 2568-2575
[5]. Wang S and Fu Y 2016 Face behind makeup Proc. of the AAAI Conf. on Artificial Intelligence pp 58-64
[6]. Zhu J Y, Park T, Isola P, & Efros A A 2017 Unpaired image-to-image translation using cycle-consistent adversarial networks Proc. of the IEEE/CVF Int. Conf. on Computer Vision pp 2223-2232
[7]. Li T, Qian R, Dong C, Liu S, Yan Q, Zhu W and Lin L 2018 Beautygan: Instance-level facial makeup transfer with deep generative adversarial network Proc. of the 26th ACM Int. Conf. on Multimedia pp 645-653
[8]. Chang H, Lu J, Yu F and Finkelstein A 2018 Pairedcyclegan: Asymmetric style transfer for applying and removing makeup Proc. of the IEEE/CVF Conf. on Computer Vision and Pattern Recognition pp 40-48
[9]. Gu Q, Wang G, Chiu M T, Tai Y W and Tang C K 2019 Ladn: Local adversarial disentangling network for facial makeup and de-makeup Proc. of the IEEE/CVF Int. Conf. on Computer Vision pp 10481-10490
[10]. Jiang W, Liu S, Gao C, Cao J, He R, Feng J and Yan S 2020 Psgan: Pose and expression robust spatial-aware gan for customizable makeup transfer Proc. of the IEEE/CVF Conf. on Computer Vision and Pattern Recognition pp 5194-5202
[11]. Deng H, Han C, Cai H, Han G and He S 2021 Spatially-invariant style-codes controlled makeup transfer Proc. of the IEEE/CVF Conf. on Computer Vision and Pattern Recognition pp 6549-6557
[12]. Lyu Y, Dong J, Peng B, Wang W and Tan T 2021 SOGAN: 3D-Aware Shadow and Occlusion Robust GAN for Makeup Transfer Proc. of the 29th ACM Int. Conf. on Multimedia pp 3601-3609
[13]. Nguyen T, Tran A T and Hoai M 2021 Lipstick ain't enough: beyond color matching for in-the-wild makeup transfer Proc. of the IEEE/CVF Conf. on Computer Vision and Pattern Recognition pp 13305-13314
[14]. Sun Z, Chen Y and Xiong S 2022 Ssat: A symmetric semantic-aware transformer network for makeup transfer and removal Proc. of the AAAI Conf. on Artificial Intelligence pp 2325-2334
[15]. Yang C, He W, Xu Y and Gao Y 2022 Elegant: Exquisite and locally editable gan for makeup transfer Proc. of European Conf. on Computer Vision pp 737-754
[16]. Wu S, Rupprecht C and Vedaldi A 2020 Unsupervised learning of probably symmetric deformable 3d objects from images in the wild Proc. of the IEEE/CVF Conf. on Computer Vision and Pattern Recognition pp 1-10
[17]. Feng Y, Wu F, Shao X, Wang Y and Zhou X 2018 Joint 3d face reconstruction and dense alignment with position map regression network Proc. of European Conf. on Computer Vision pp 534-551
[18]. Yu C, Wang J, Peng C, Gao C, Yu G and Sang N 2018 Bisenet: Bilateral segmentation network for real-time semantic segmentation Proc. of European Conf. on Computer Vision pp 325-341
[19]. Simonyan K and Zisserman A 2015 Very deep convolutional networks for large-scale image recognition 3rd Int. Conf. on Learning Representations pp 1-14
[20]. Deng J, Dong W, Socher R, Li L J, Li K and Fei-Fei L 2009 Imagenet: A large-scale hierarchical image database Proc. of the IEEE/CVF Conf. on Computer Vision and Pattern Recognition pp 248-255
[21]. Mao X, Li Q, Xie H, Lau R Y, Wang Z and Paul Smolley S 2017 Least squares generative adversarial networks Proc. of the IEEE/CVF Int. Conf. on Computer Vision pp 2794-2802
Cite this article
Song,Z. (2024). Local highlight and shadow adaptively repairing GAN for illumination-robust makeup transfer. Advances in Engineering Innovation,7,1-8.
Data availability
The datasets used and/or analyzed during the current study will be available from the authors upon reasonable request.
Disclaimer/Publisher's Note
The statements, opinions and data contained in all publications are solely those of the individual author(s) and contributor(s) and not of EWA Publishing and/or the editor(s). EWA Publishing and/or the editor(s) disclaim responsibility for any injury to people or property resulting from any ideas, methods, instructions or products referred to in the content.
About volume
Journal:Advances in Engineering Innovation
© 2024 by the author(s). Licensee EWA Publishing, Oxford, UK. This article is an open access article distributed under the terms and
conditions of the Creative Commons Attribution (CC BY) license. Authors who
publish this series agree to the following terms:
1. Authors retain copyright and grant the series right of first publication with the work simultaneously licensed under a Creative Commons
Attribution License that allows others to share the work with an acknowledgment of the work's authorship and initial publication in this
series.
2. Authors are able to enter into separate, additional contractual arrangements for the non-exclusive distribution of the series's published
version of the work (e.g., post it to an institutional repository or publish it in a book), with an acknowledgment of its initial
publication in this series.
3. Authors are permitted and encouraged to post their work online (e.g., in institutional repositories or on their website) prior to and
during the submission process, as it can lead to productive exchanges, as well as earlier and greater citation of published work (See
Open access policy for details).