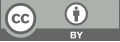
A bibliometric analysis of semantic segmentation in remote sensing images from 1981 to 2024
- 1 Wuhan University
* Author to whom correspondence should be addressed.
Abstract
Semantic segmentation of remote sensing images plays a crucial role in various fields, such as disaster assessment, forestry measurement, and environmental monitoring. This study conducts a bibliometric analysis to investigate the research trends and development of semantic segmentation in remote sensing from 1981 to 2024. Utilizing the Web of Science Core Collection database, we retrieved representative literature data and analyzed the publication trends, main source journals, institutional cooperation, and keyword co-occurrence networks. The results show that the number of publications in this field has increased significantly since 2000, with an exponential growth pattern. Remote sensing is the most active research topic, followed by imaging science, photographic technology, and electrical and electronic engineering. The analysis of institutional cooperation reveals that Chinese research institutions, such as the Chinese Academy of Sciences and Wuhan University, play a leading role in this field and maintain close collaboration with research institutions worldwide. The keyword co-occurrence network analysis demonstrates the evolution of research focus from basic remote sensing and image processing techniques in the early stage to advanced image processing algorithms in the middle stage and deep learning and neural network technologies in the recent stage. The findings provide valuable insights into the current status and future trends of semantic segmentation in remote sensing, highlighting the importance of international cooperation and interdisciplinary exchange in driving technological innovation.
Keywords
remote sensing, semantic segmentation, bibliometric analysis, research trends, international cooperation, interdisciplinary research
[1]. Jia, K., Yao, Y., Wei, X., Gao, S., Jiang, B. and Zhao, X. (2013). A review on fractional vegetation cover estimation using remote sensing. Advances in Earth Science, 28(7), p.774.
[2]. Li, Y. S., Wu, K., Ouyang, S., Yang, K., Li, H. P., and Zhang, Y. J. (2024). Geographic knowledge graph-guided remote sensing image semantic segmentation. National Remote Sensing Bulletin, 28(2), 455-469. doi:10.11834/jrs.20231110.
[3]. Khudzari, J.M., Kurian, J., Tartakovsky, B., and Raghavan, G.V. (2018). Bibliometric analysis of global research trends on microbial fuel cells using Scopus database. Biochemical Engineering Journal, 136, 51-60.
[4]. Zou, X., Yue, W.L., and Le Vu, H. (2018). Visualization and analysis of mapping knowledge domain of road safety studies. Accident Analysis & Prevention, 118, 131-145.
[5]. Geng, Y., Chen, W., Liu, Z., Chiu, A.S., Han, W., Liu, Z., Zhong, S., Qian, Y., You, W., and Cui, X. (2017). A bibliometric review: Energy consumption and greenhouse gas emissions in the residential sector. Journal of Cleaner Production, 159, 301-316.
[6]. Hu, K., Qi, K., Guan, Q., Wu, C., Yu, J., Qing, Y., Zheng, J., Wu, H., and Li, X. (2017). A scientometric visualization analysis for night-time light remote sensing research from 1991 to 2016. Remote Sensing, 9(8), p.802.
[7]. Yang, B., Mao, Y., Chen, J., Liu, J. Q., Chen, J., and Yan, K. (2023). Review of remote sensing change detection in deep learning: Bibliometric and analysis. National Remote Sensing Bulletin, 27(9), 1988-2005. doi:10.11834/jrs.20222156.
[8]. Yan, K., Chen, H. M., Fu, D. J., Zeng, Y. L., Dong, J. W., Li, S. W., Wu, Q. S., Li, H. L., and Du, S. Y. (2022). Bibliometric visualization analysis related to remote sensing cloud computing platforms. National Remote Sensing Bulletin, 26(2), 310-323. doi:10.11834/jrs.20211328.
[9]. Huang, M. R., Jian, H. D., Xu, C., Hao, J. S., Yan, J., Liu, L. Y., Fan, X. T., and Guo, H. D. (2023). Bibliometrics spatial-temporal evolution analysis of the development of remote sensing. National Remote Sensing Bulletin, 27(11), 2449-2466. doi:10.11834/jrs.20232659.
[10]. Mao, D. H., Wang, Z. M., Jia, M. M., Luo, L., Niu, Z. G., Jiang, W. G., and Sun, W. W. (2023). Review of global studies on the remote sensing of wetlands from 1975 to 2020. National Remote Sensing Bulletin, 27(6), 1270-1280. doi:10.11834/jrs.20231022.
[11]. Liu, F., Lin, A., Wang, H., Peng, Y., and Hong, S. (2016). Global research trends of geographical information system from 1961 to 2010: a bibliometric analysis. Scientometrics, 106, 751-768.
[12]. Jiang, Q.X., and Liu, H.P. (2004). Extracting TM image information using texture analysis. Journal of Remote Sensing, (5), 458-464. doi:10.11834/jrs.20040512.
[13]. Zhang, J. T., and Wan, Q. (1999). Study on GIS integration platform framework architecture. Journal of Remote Sensing, (1). doi:10.11834/jrs.19990112.
[14]. Xu, J., and Zheng, J. (1990). A texture analysis technique to wavelength and direction extracting from ocean wave image. Journal of Remote Sensing, (4), 286-296. doi:10.11834/jrs.1990043.
[15]. Zhao, S.H., Feng, X.Z., Du, J.K., et al. (2003). SPIN-2 panchromatic and SPOT-4 multi-spectral image fusion based on support vector machine. Journal of Remote Sensing, (5), 407-411. doi:10.11834/jrs.20030511.
[16]. Liu, Y.X., Li, M.C., and Mao, L. (2006). An algorithm of multi-spectral remote sensing image segmentation based on edge information. Journal of Remote Sensing, (3), 350-356. doi:10.11834/jrs.20060354.
[17]. Qiu, D.D., Ma, H.C., Yang, Y., et al. (2006). A study of dam detection based on multi-source satellite images fusion. Journal of Remote Sensing, (4), 449-455. doi:10.11834/jrs.20060467.
[18]. Yang, Z.G., Huang, X.T., Zhou, Z.M. (2008). A 2-D GLR target detection approach of UWB SAR based on multi-resolution feature. Journal of Remote Sensing, (2), 239-245. doi:10.11834/jrs.20080231.
[19]. Sindhwani, V., Sainath, T., and Kumar, S. (2015). Structured transforms for small-footprint deep learning. Advances in Neural Information Processing Systems, 28.
[20]. Li, C.K., Zeng, Q.G., Fang, J., Wu, N., and Wu, K.H. (2021). Road extraction in rural areas from high resolution remote sensing image using an improved full convolution network. National Remote Sensing Bulletin, 25(9), 1978-1988. doi:10.11834/jrs.20219209.
[21]. Xiao, C.J., Li, Y., Zhang, H.Q., and Chen, J. (2020). Semantic segmentation of remote sensing image based on deep fusion networks and conditional random field. Journal of Remote Sensing (Chinese), 24(3), 254-264. doi:10.11834/jrs.20208298.
[22]. Wu, Q., Ni, K., and Zheng, Z. (XXXX). Remote sensing image scene classification based on two-stage high-order transformer. National Remote Sensing Bulletin, XX(XX), 1-13. doi:10.11834/jrs.20233332.
Cite this article
Lin,Y. (2024). A bibliometric analysis of semantic segmentation in remote sensing images from 1981 to 2024. Advances in Engineering Innovation,9,34-41.
Data availability
The datasets used and/or analyzed during the current study will be available from the authors upon reasonable request.
Disclaimer/Publisher's Note
The statements, opinions and data contained in all publications are solely those of the individual author(s) and contributor(s) and not of EWA Publishing and/or the editor(s). EWA Publishing and/or the editor(s) disclaim responsibility for any injury to people or property resulting from any ideas, methods, instructions or products referred to in the content.
About volume
Journal:Advances in Engineering Innovation
© 2024 by the author(s). Licensee EWA Publishing, Oxford, UK. This article is an open access article distributed under the terms and
conditions of the Creative Commons Attribution (CC BY) license. Authors who
publish this series agree to the following terms:
1. Authors retain copyright and grant the series right of first publication with the work simultaneously licensed under a Creative Commons
Attribution License that allows others to share the work with an acknowledgment of the work's authorship and initial publication in this
series.
2. Authors are able to enter into separate, additional contractual arrangements for the non-exclusive distribution of the series's published
version of the work (e.g., post it to an institutional repository or publish it in a book), with an acknowledgment of its initial
publication in this series.
3. Authors are permitted and encouraged to post their work online (e.g., in institutional repositories or on their website) prior to and
during the submission process, as it can lead to productive exchanges, as well as earlier and greater citation of published work (See
Open access policy for details).