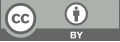
Short-term photovoltaic power generation prediction based on VMD-MSI-GWO-KELM
- 1 North China Electric Power University (Baoding)
* Author to whom correspondence should be addressed.
Abstract
To improve the accuracy of photovoltaic power prediction, a combined prediction model integrating multiple algorithms is proposed. First, key factors are selected, and the Gaussian Mixture Model (GMM) is used to cluster and generate historical days with high correlation to the forecast day. Then, a photovoltaic power prediction model based on Variational Mode Decomposition (VMD) and an improved Grey Wolf Optimizer Kernel Extreme Learning Machine (MSI-GWO-KELM) is constructed. Finally, actual data from a photovoltaic power station in Australia is used as a case study. The results show that the VMD-MSI-GWO-KELM model is suitable for different weather conditions and offers better prediction accuracy than other models.
Keywords
photovoltaic power prediction, GWO, KELM, VMD
[1]. Ai, L., Si, J. L., & Chen, X. J. (2024). Current development status and prospects of China’s photovoltaic power generation industry [J/OL]. Hydropower Generation, 1-6 [2025-03-20]. http://kns.cnki.net/kcms/detail/11.1845.TV.20250319.1742.006.html
[2]. Wang, S. B., Wang, K., & Sun, S. M. (2025). Analysis and review of ultra-short-term photovoltaic power forecasting technology [J]. Shandong Electric Power Technology, 52(02), 55-64. DOI:10.20097/j.cnki.issn1007-9904.2025.02.006
[3]. Wang, R., Zhang, L. T., & Lu, J. (2024). Short-term photovoltaic power forecasting based on novel similar-day selection and VMD-NGO-BiGRU [J]. Journal of Hunan University (Natural Sciences), 51(02), 68-80. DOI:10.16339/j.cnki.hdxbzkb.2024227
[4]. Feng, J. M., Xi, W. A., & Lin, H. (2025). Short-term photovoltaic power forecasting based on clustering SABO-VMD and combined neural network [J]. Acta Energiae Solaris Sinica, 46(02), 357-366. DOI:10.19912/j.0254-0096.tynxb.2023-1681
[5]. Yao, Q. C., Xiang, W. G., & Chen, S. Y. (2025). Photovoltaic power forecasting based on ICEEMDAN-KPCA-ICPA-LSTM [J]. Journal of Power Engineering, 45(03), 374-382. DOI:10.19805/j.cnki.jcspe.2025.230777
[6]. Meng, Y. K., Xu, Y., & Wang, X. P. (2024). Study on combined forecasting model of photovoltaic output based on similar-day selection and PCA-LSTM [J]. Acta Energiae Solaris Sinica, 45(07), 453-461. DOI:10.19912/j.0254-0096.tynxb.2023-0498
[7]. Fang, C. X., Zheng, J. Y., & Zhang, Z. H. (2025). Distributed photovoltaic power forecasting method based on similar-day selection and VMD-DBO-KELM [J/OL]. High Voltage Technology, 1-11 [2025-03-20]. https://doi.org/10.13336/j.1003-6520.hve.20240792
[8]. Liu, Q. B., & Li, J. (2024). Short-term photovoltaic power forecasting based on SOM-FCM and KELM combination method (English) [J]. Journal of Measurement Science and Instrumentation, 15(02), 204-215
[9]. Shang, L. Q., Li, H. B., Hou, & Y. D. (2022). Short-term photovoltaic power forecasting based on VMD-ISSA-KELM [J]. Power System Protection and Control, 50(21), 138-148. DOI:10.19783/j.cnki.pspc.220140
[10]. Long, J., Zuo, S. L., & Xu, L. (2024). Dam deformation prediction model based on influencing factor selection and GWO-KELM [J]. China Rural Water and Hydropower, (08), 194-199+207
[11]. Chen, M., Chen, Y., & Niu, X. L. (2022). Multi-strategy improved grey wolf algorithm for solving global optimization problems [J]. Foreign Electronic Measurement Technology, 41(11), 22-29. DOI:10.19652/j.cnki.femt.2204260
[12]. Zhai, M. Q. (2022). Two-stage structural damage identification method based on improved grey wolf algorithm and artificial neural network [D]. Xiamen University. DOI:10.27424/d.cnki.gxmdu.2022.001599
[13]. Yang, C., Niu, F. J., & Han, M. L. (2025). Research on photovoltaic array fault diagnosis method based on improved grey wolf algorithm optimized extreme learning machine [J]. Power Generation Technology, 46(01), 72-82
Cite this article
Li,X. (2025). Short-term photovoltaic power generation prediction based on VMD-MSI-GWO-KELM. Advances in Engineering Innovation,16(3),75-82.
Data availability
The datasets used and/or analyzed during the current study will be available from the authors upon reasonable request.
Disclaimer/Publisher's Note
The statements, opinions and data contained in all publications are solely those of the individual author(s) and contributor(s) and not of EWA Publishing and/or the editor(s). EWA Publishing and/or the editor(s) disclaim responsibility for any injury to people or property resulting from any ideas, methods, instructions or products referred to in the content.
About volume
Journal:Advances in Engineering Innovation
© 2024 by the author(s). Licensee EWA Publishing, Oxford, UK. This article is an open access article distributed under the terms and
conditions of the Creative Commons Attribution (CC BY) license. Authors who
publish this series agree to the following terms:
1. Authors retain copyright and grant the series right of first publication with the work simultaneously licensed under a Creative Commons
Attribution License that allows others to share the work with an acknowledgment of the work's authorship and initial publication in this
series.
2. Authors are able to enter into separate, additional contractual arrangements for the non-exclusive distribution of the series's published
version of the work (e.g., post it to an institutional repository or publish it in a book), with an acknowledgment of its initial
publication in this series.
3. Authors are permitted and encouraged to post their work online (e.g., in institutional repositories or on their website) prior to and
during the submission process, as it can lead to productive exchanges, as well as earlier and greater citation of published work (See
Open access policy for details).