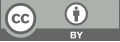
AI-driven software engineering
- 1 Saint Leo University
* Author to whom correspondence should be addressed.
Abstract
The intersection of artificial intelligence (AI) and software engineering marks a transformative phase in the technology industry. This paper delves into AI-driven software engineering, exploring its methodologies, implications, challenges, and benefits. Drawing from data sources such as GitHub and Bitbucket and insights from industry experts, the study offers a comprehensive view of the current landscape. While the results indicate a promising uptrend in the integration of AI techniques in software development, challenges like model interpretability, ethical concerns, and integration complexities emerge as significant. Nevertheless, the transformative potential of AI within software engineering is profound, ushering in new paradigms of efficiency, innovation, and user experience. The study concludes by emphasizing the need for further research, better tooling, ethical guidelines, and education to fully harness the potential of AI-driven software engineering.
Keywords
AI-driven development, software engineering, model interpretability, ethical AI integration, software innovation
[1]. Arnold, V., Benford, T., & Canada, J. (2019). The role of interpretability in ethical software engineering. Ethics and Information Technology, 21(3), 205-216.
[2]. Fraser, G., & Arcuri, A. (2013). Whole test suite generation. IEEE Transactions on Software Engineering, 39(2), 276-291.
[3]. Le Goues, C., & Weimer, W. (2017). Specification mining with few false positives. ACM Transactions on Software Engineering and Methodology (TOSEM), 21(1), 4.
[4]. Mancoridis, S., Mitchell, B. S., Rorres, C., Chen, Y., & Gansner, E. R. (1998). Using automatic clustering to produce high-level system organizations of source code. Proceedings of IWPC, 45-53.
[5]. Menzies, T., Greenwald, J., & Frank, A. (2007). Data mining static code attributes to learn defect predictors. IEEE Transactions on Software Engineering, 34(2), 2-13.
[6]. Nakamura, Y., & Shinozaki, K. (2018). Chatbot as a new business communication tool: The case of NTT east. Journal of Business Research, 100, 516-528.
[7]. White, M., Tufano, M., Vendome, C., & Poshyvanyk, D. (2015). Deep learning code fragments for code clone detection. Proceedings of ASE, 87-98.
[8]. Zhang, F., Mockus, A., Keivanloo, I., & Zou, Y. (2020). Towards building a universal defect prediction model. ACM Transactions on Software Engineering and Methodology (TOSEM), 25(3), 1-26.
[9]. Zowghi, D., & Offen, R. (1997). A logical framework for modeling and reasoning about the evolution of requirements. Proceedings of RE, 247-257.
[10]. Brooks, F. P. (1987). No silver bullet—essence and accidents of software engineering. Computer, 20(4), 10-19.
[11]. Javdani, T., Shamsaei, A., & Shahin, M. (2021). AI-driven software engineering: Challenges and future directions. Software: Practice and Experience, 51(4), 715-741.
[12]. Raschke, P., Bartels, J., & Gruhn, V. (2019). A survey on the application of machine learning in software engineering. arXiv preprint arXiv:1905.13209.
[13]. Sommerville, I. (2016). Software engineering. Pearson.
[14]. Wang, S., Wen, J., Wang, X., & Zhou, R. (2020). Machine learning in software engineering: Models, methods, and applications. ACM Computing Surveys (CSUR), 53(4), 1-38.
Cite this article
Ali,J.M. (2023). AI-driven software engineering. Advances in Engineering Innovation,3,17-21.
Data availability
The datasets used and/or analyzed during the current study will be available from the authors upon reasonable request.
Disclaimer/Publisher's Note
The statements, opinions and data contained in all publications are solely those of the individual author(s) and contributor(s) and not of EWA Publishing and/or the editor(s). EWA Publishing and/or the editor(s) disclaim responsibility for any injury to people or property resulting from any ideas, methods, instructions or products referred to in the content.
About volume
Journal:Advances in Engineering Innovation
© 2024 by the author(s). Licensee EWA Publishing, Oxford, UK. This article is an open access article distributed under the terms and
conditions of the Creative Commons Attribution (CC BY) license. Authors who
publish this series agree to the following terms:
1. Authors retain copyright and grant the series right of first publication with the work simultaneously licensed under a Creative Commons
Attribution License that allows others to share the work with an acknowledgment of the work's authorship and initial publication in this
series.
2. Authors are able to enter into separate, additional contractual arrangements for the non-exclusive distribution of the series's published
version of the work (e.g., post it to an institutional repository or publish it in a book), with an acknowledgment of its initial
publication in this series.
3. Authors are permitted and encouraged to post their work online (e.g., in institutional repositories or on their website) prior to and
during the submission process, as it can lead to productive exchanges, as well as earlier and greater citation of published work (See
Open access policy for details).