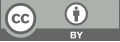
The investigation of artificial intelligence-based applications in music education
- 1 Dalian Maritime University
* Author to whom correspondence should be addressed.
Abstract
The field of music education has garnered significant attention due to the burgeoning advancements in artificial intelligence technology. Currently, there are many works on applying Artificial Intelligence (AI) technology to the field of music education, and this review aims to comprehensively summarize the application of AI methods in multiple subfields of music education. In this paper, according to the focus of AI technology, the areas of music education to which it has been applied are divided into four modules, namely personalized learning, automatic assessment, composition assistance and interactive teaching. Firstly, representative AI methods applied to each of these four subfields are introduced, including but not limited to: the principle of the AI method, the improvement brought by the application. The text then describes the current status of the application of these methods in their domains, discusses the limitations of the application, and makes brief conjectures on how to break through these limitations. The research in this review paper is important for the development of AI technology applied to the field of music education. At the same time, this review also provides directions and suggestions for future research to promote the further development of AI in the field of music education.
Keywords
music education, artificial intelligence, machine learning, deep learning
[1]. Roads C 1996 The computer music tutorial (MIT Press)
[2]. Miranda E R 2012 Musical creativity and the affordances of technology Contemporary (Music Review 31(1)) p 17-35
[3]. Rowe R 2001 Machine Musicianship (MIT Press)
[4]. Li T Ogihara M and Li Q 2008 A survey of content-based music retrieval systems (Journal of Intelligent Information Systems, 30(1)) p 1-40
[5]. Hinton G E and Salakhutdinov R R 2006 Reducing the dimensionality of data with neural networks (Science, 313(5786)) p 504-507.
[6]. LeCun Y Bottou L Bengio Y and Haffner P 1998 Gradient-based learning applied to document recognition (Proceedings of the IEEE, 86(11)) p 2278-2324.
[7]. Hochreiter S Bengio Y Frasconi P and Schmidhuber J 2001 Gradient flow in recurrent nets: The difficulty of learning long-term dependencies. In J. F. Kolen & S. C. Kremer (Eds.), A Field Guide to Dynamical Recurrent Neural Networks pp. 237-244 IEEE Press
[8]. Jie W Boo J Wang Y and Loscos A A 2006 Violin Music Transcriber for Personalized Learning. (In 2006 IEEE International Conference on Multimedia and Expo ) pp 2081-2084 Toronto ON Canada: IEEE. doi 10.1109/ICME.2006.262644
[9]. Chen K Y and Lin Y C 2019 Automatic assessment of tone quality in violin music performance IEEE Transactions on Audio, Speech, and Language Processing, 25(5), p1050-1061.
[10]. Touros G and Giannakopoulos T 2023 Video Soundtrack Evaluation with Machine Learning: Data Availability, Feature Extraction, and Classification. In A. Biswas, E. Wennekes, A. Wieczorkowska, & R. H. Laskar (Eds.), Advances in Speech and Music Technology (pp. 137-157). Signals and Communication Technology. Springer, Cham
[11]. Dong H.-W Hsiao W.-Y Yang L.-C and Yang Y.-H 2018 MuseGAN: Multi-track Sequential Generative Adversarial Networks for Symbolic Music Generation and Accompaniment. Proceedings of the AAAI Conference on Artificial Intelligence, 32(1). https://doi.org/10.1609/aaai.v32i1.11312
Cite this article
Wang,G. (2024). The investigation of artificial intelligence-based applications in music education . Applied and Computational Engineering,36,210-214.
Data availability
The datasets used and/or analyzed during the current study will be available from the authors upon reasonable request.
Disclaimer/Publisher's Note
The statements, opinions and data contained in all publications are solely those of the individual author(s) and contributor(s) and not of EWA Publishing and/or the editor(s). EWA Publishing and/or the editor(s) disclaim responsibility for any injury to people or property resulting from any ideas, methods, instructions or products referred to in the content.
About volume
Volume title: Proceedings of the 2023 International Conference on Machine Learning and Automation
© 2024 by the author(s). Licensee EWA Publishing, Oxford, UK. This article is an open access article distributed under the terms and
conditions of the Creative Commons Attribution (CC BY) license. Authors who
publish this series agree to the following terms:
1. Authors retain copyright and grant the series right of first publication with the work simultaneously licensed under a Creative Commons
Attribution License that allows others to share the work with an acknowledgment of the work's authorship and initial publication in this
series.
2. Authors are able to enter into separate, additional contractual arrangements for the non-exclusive distribution of the series's published
version of the work (e.g., post it to an institutional repository or publish it in a book), with an acknowledgment of its initial
publication in this series.
3. Authors are permitted and encouraged to post their work online (e.g., in institutional repositories or on their website) prior to and
during the submission process, as it can lead to productive exchanges, as well as earlier and greater citation of published work (See
Open access policy for details).