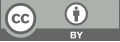
A special target detection called You Only Looks Once
- 1 School of Engineering Science
* Author to whom correspondence should be addressed.
Abstract
This paper proposes a unique target detection called You Only Look Once (YOLO for short) and a recognition method. Unlike the previous idea of many classifiers with object detection functions, the object detection box is set as a spatially separated bounding box and the regression problem of related class probabilities is realized. The neural network model can directly scan the entire image during testing, predicting bounding boxes and class probabilities from the complete picture. At the same time, because the whole detection channel relies on a single neural network, it is more straightforward and concise when upgrading and updating. The unified architecture used in this paper is fast, with smaller versions of the YOLO model processing a staggering 155 frames per second. At the same time, it has also achieved excellent results in mAP. Compared with other detection systems, YOLOv3 has optimized many past problems, including positioning errors. At the same time, the probability of predicting false detections in the absence of false detections is small. Finally, like the YOLO base model, YOLOv3 may produce significant errors when processing abstract works of art and images with a large number of small objects. However, its actual results are still better than detection methods such as Region -Convolutional Neural Networks (R-CNN).
Keywords
Convolutional Neural Network, YOLO, Target Detection
[1]. Zhang, S., Chai, L., and Jin, L., 2020. Vehicle detection in UAV aerial images based on improved yolov3. 2020 IEEE International Conference on Networking, Sensing and Control (ICNSC).
[2]. Zhang, S., Wu, Q., Su, P., and Ma, J., 2022. Design and simulation investigation of 3*9 led matrix headlamp to realize anti-glare function. Advances in Transdisciplinary Engineering.
[3]. Chen, S., Zhao, S., and Lan, Q., 2022. Residual block based nested U-type architecture for Multi-modal brain tumor image segmentation. Frontiers in Neuroscience, 16.
[4]. Shakil A.*, S., and Kureshi, Dr.A., 2020. Object detection and tracking using YOLO V3 framework for increased Resolution Video. International Journal of Innovative Technology and Exploring Engineering, 9(6), pp.118–125.
[5]. Zhang, S., Wu, Q., Su, P., and Ma, J., 2022. Design and simulation investigation of 3*9 led matrix headlamp to realize anti-glare function. Advances in Transdisciplinary Engineering.
[6]. Huang, Z., Zhang, P., Liu, R., and Li, D., 2021. Immature apple detection method based on improved yolov3. ASP Transactions on Internet of Things, 1(1), pp.9–13.
[7]. Auliya, A., Pradani, W., and Haryanto, T., 2022. Kenaf flower detection using yolov3. 2022 3rd International Conference on Artificial Intelligence and Data Sciences (AiDAS).
[8]. Li, S., Li, Y., and Lu, Y., 2020. Comparative optimization and application of rectification schemes for shear wall structure. IOP Conference Series: Earth and Environmental Science, 455(1), p.012023.
[9]. Alisetti, S.N., Purushotham, S., and Mahtani, L., 2019. Guiding and navigation for the blind using deep convolutional neural network based predictive object tracking. International Journal of Engineering and Advanced Technology, 9(1s3), pp.306–313.
[10]. Redmon, J., Divvala, S., Girshick, R., and Farhadi, A., 2016. You only look once: Unified, real-time object detection. 2016 IEEE Conference on Computer Vision and Pattern Recognition (CVPR).
Cite this article
Li,L. (2024). A special target detection called You Only Looks Once. Applied and Computational Engineering,39,92-102.
Data availability
The datasets used and/or analyzed during the current study will be available from the authors upon reasonable request.
Disclaimer/Publisher's Note
The statements, opinions and data contained in all publications are solely those of the individual author(s) and contributor(s) and not of EWA Publishing and/or the editor(s). EWA Publishing and/or the editor(s) disclaim responsibility for any injury to people or property resulting from any ideas, methods, instructions or products referred to in the content.
About volume
Volume title: Proceedings of the 2023 International Conference on Machine Learning and Automation
© 2024 by the author(s). Licensee EWA Publishing, Oxford, UK. This article is an open access article distributed under the terms and
conditions of the Creative Commons Attribution (CC BY) license. Authors who
publish this series agree to the following terms:
1. Authors retain copyright and grant the series right of first publication with the work simultaneously licensed under a Creative Commons
Attribution License that allows others to share the work with an acknowledgment of the work's authorship and initial publication in this
series.
2. Authors are able to enter into separate, additional contractual arrangements for the non-exclusive distribution of the series's published
version of the work (e.g., post it to an institutional repository or publish it in a book), with an acknowledgment of its initial
publication in this series.
3. Authors are permitted and encouraged to post their work online (e.g., in institutional repositories or on their website) prior to and
during the submission process, as it can lead to productive exchanges, as well as earlier and greater citation of published work (See
Open access policy for details).