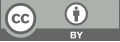
Multi-source serialization cross-domain recommendation algorithm based on deep learning
- 1 Dalian Polytechnic University
- 2 Dalian Polytechnic University
- 3 ,Dalian Polytechnic University
* Author to whom correspondence should be addressed.
Abstract
Cross-domain recommendation is an effective approach to solve the cold start and data sparsity problems in recommendation systems. Sequential recommendation can model user behavior sequences and improve the accuracy of recommendation. Currently, few recommendation algorithms consider both aspects together, and most of them do not utilize multi-source information sufficiently. In view of this, this paper proposes a multi-source serialization cross-domain recommendation model, which fully considers the temporal and contextual relationships in two domains, and fuses multi-source information on the basis of achieving cross-domain recommendation tasks, and reinforce the embedding representation by fitting the interest forgetting function. Finally, use a Multilayer Perceptron as the mapping function to learn the nonlinear mapping relationship between the source domain and the target domain, subsequently enabling recommendations for new users in the target domain. On Amazon dataset, this model can significantly enhance the accuracy of recommendation.
Keywords
Cross-Domain Recommendation, Multi-Source Information, Serialization, Interest Forgetting Curve
[1]. Liu Huiting,Guo Lingling,Li Peipei,Zhao Peng,Wu Xindong. Collaborative filtering with a deep adversarial and attention network for cross-domain recommendation[J]. Information Sciences,2021,565.
[2]. Zhang L, Ge Y, Ma J, et al. Knowledge-aware Neural Collective Matrix Factorization for Cross-domain Recommendation[J]. arXiv preprint arXiv:2206.13255, 2022.
[3]. Li P, Brost B, Tuzhilin A. Adversarial Learning for Cross Domain Recommendations[J]. ACM Transactions on Intelligent Systems and Technology (TIST), 2022.
[4]. Sahu A K. User and item spaces transfer from additional domains for cross-domain recommender systems[J]. Applied Intelligence, 2023, 53(5): 5766-5783.
[5]. Ai Z, Wang S, Jia S, et al. Core Interests Focused Self-attention for Sequential Recommendation[C]//International Conference on Database Systems for Advanced Applications. Springer, Cham, 2022: 306-314.
[6]. Guo J, Ji W, Yuan J, et al. Multi-channel Orthogonal Decomposition Attention Network for Sequential Recommendation[C]//Pacific-Asia Conference on Knowledge Discovery and Data Mining. Springer, Cham, 2022: 288-300.
[7]. Kheldouni A, Boumhidi J. A Study of Bidirectional Encoder Representations from Transformers for Sequential Recommendations[C]//2022 International Conference on Intelligent Systems and Computer Vision (ISCV).IEEE, 2022: 1-5.
[8]. Chen L, Zhu H. Behavior prediction based on a Commodity Utility-Behavior Sequence model[J]. Machine Learning with Applications, 2022, 9: 100314.
[9]. Hou Y, Mu S, Zhao W X, et al. Towards Universal Sequence Representation Learning for Recommender Systems[C]//Proceedings of the 28th ACM SIGKDD Conference on Knowledge Discovery and Data Mining. 2022: 585-593.
[10]. Chen J, Wang C, Wang J. Modeling the interest-forgetting curve for music recommendation[C]//Proceedings of the 22nd ACM international conference on Multimedia. 2014: 921-924.
[11]. Man T, Shen H, Jin X, et al. Cross-domain recommendation: An embedding and mapping approach[C]//IJCAI. 2017, 17: 2464-2470.
[12]. Kang S K, Hwang J, Lee D, et al. Semi-supervised learning for cross-domain recommendation to cold-start users[C]//Proceedings of the 28th ACM International Conference on Information and Knowledge Management. 2019: 1563-1572.
[13]. Zhu Y, Ge K, Zhuang F, et al. Transfer-meta framework for cross-domain recommendation to cold-start users[C]//Proceedings of the 44th International ACM SIGIR Conference on Research and Development in Information Retrieval. 2021: 1813-1817.
Cite this article
Chen,Z.;Wang,Z.;Wang,X. (2024). Multi-source serialization cross-domain recommendation algorithm based on deep learning. Applied and Computational Engineering,44,150-159.
Data availability
The datasets used and/or analyzed during the current study will be available from the authors upon reasonable request.
Disclaimer/Publisher's Note
The statements, opinions and data contained in all publications are solely those of the individual author(s) and contributor(s) and not of EWA Publishing and/or the editor(s). EWA Publishing and/or the editor(s) disclaim responsibility for any injury to people or property resulting from any ideas, methods, instructions or products referred to in the content.
About volume
Volume title: Proceedings of the 2023 International Conference on Machine Learning and Automation
© 2024 by the author(s). Licensee EWA Publishing, Oxford, UK. This article is an open access article distributed under the terms and
conditions of the Creative Commons Attribution (CC BY) license. Authors who
publish this series agree to the following terms:
1. Authors retain copyright and grant the series right of first publication with the work simultaneously licensed under a Creative Commons
Attribution License that allows others to share the work with an acknowledgment of the work's authorship and initial publication in this
series.
2. Authors are able to enter into separate, additional contractual arrangements for the non-exclusive distribution of the series's published
version of the work (e.g., post it to an institutional repository or publish it in a book), with an acknowledgment of its initial
publication in this series.
3. Authors are permitted and encouraged to post their work online (e.g., in institutional repositories or on their website) prior to and
during the submission process, as it can lead to productive exchanges, as well as earlier and greater citation of published work (See
Open access policy for details).