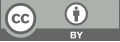
Signal detection algorithms for massive MIMO system
- 1 Jinan University
* Author to whom correspondence should be addressed.
Abstract
As 5G communication networks are maturing, we have higher and higher requirements for the detection of communication signals. In this paper, for the Massive MIMO system signal detection problem, we mainly summarize the detection algorithms that can be used to replace the traditional ZF and MMSE, so as to avoid large-scale matrix inverse and reduce the computational complexity. It mainly includes the general iterative method, typically represented by SSOR, which makes the transmit signal matrix constantly close to the ideal value by iterating; the other is the level expansion class solution method, which takes the order expansion of the level as the initial value of the iteration to accelerate the convergence rate of the algorithm, typically represented by the MLI algorithm. However, today where the demand for communication is gradually increasing and the number of users is constantly getting larger, the performance of the above algorithms may degrade seriously, so the AI signal detection algorithm is a good alternative, which learns autonomously through deep neural networks, including model-driven and data-driven schemes.
Keywords
Massive MIMO, Signal Detection, Traditional Methods, Neural Network, Deep Learning
[1]. B. Dongwoon, P. Cheolhee, L. Jungwon, N. Hoang, J. Singh, A. Gupta, P. Zhouyue, K. Taeyoon, L. Chaiman, K. Min-Goo, and I. Inyup, “LTEadvanced modem design: Challenges and perspectives,” IEEE Commun. Mag., vol. 50, no. 2, pp. 178–186, Feb. 2012.
[2]. H. D. Tiwari, N. B. Huynh, and B. C. Yong, “Flexible LDPC decoder using stream data processing for 802.11n and 802.16e,” IEEE Trans. Consum. Electron., vol. 57, no. 4, pp. 1505–1512, Apr. 2012.
[3]. Li Jia, et al. Research on Massive MIMO Detection Algorithm of Power 5G Network Based on Neural Network”.Proceedings of the 2022 Academic Annual Conference of Jilin Electrical Engineering Society.Ed.. Jilin University Press, 2022, 361-367.
[4]. CHANG M X,CHANG W Y. Maximum-likelihood detection for MIMO systems based on differential metrics[J].IEEE Transactions on Signal Processing,2017,65(14):3718-3732.
[5]. J. Ning, Z. Lu, T. Xie and J. Quan, “Low complexity signal detector based on SSOR method for massive MIMO systems,” 2015 IEEE International Symposium on Broadband Multimedia Systems and Broadcasting, Ghent, Belgium, 2015, pp. 1-4.
[6]. Mingyue Wang, et al. “Improved SOR signal detection algorithm in massive MIMO-TRDMA systems.” Journal of communications 42.10(2021):153-161.
[7]. Z. Wu, C. Zhang, Y. Xue, S. Xu and X. You, “Efficient architecture for soft-output massive MIMO detection with Gauss-Seidel method,” 2016 IEEE International Symposium on Circuits and Systems (ISCAS), Montreal, QC, Canada, 2016, pp. 1886-1889.
[8]. Zhang Xiaohua, Peng Xiao, and Huang Long.” Hybrid linear iterative signal detection algorithm for massive MIMO systems.” Optical Communication Research.02(2022):56-60.
[9]. Jun Li, Tongliang Xin, Bo He et al. A data-driven neural network based OFDM channel estimation and signal detection method[P]. Shandong province:CN112600772B,2022-05-17.
[10]. Cao Qian. Research and design of model-driven deep learning signal detection scheme for large-scale MIMO systems[D]. Nanjing University of Posts and Telecommunications, 2023.
[11]. Zeng Xiangjian, Shen Bin, and Yang Jian.” Massive MIMO signal detection algorithm based on SDNSR-Net deep network.” Electronic Technology Application 48.11(2022):84-88.
[12]. T. L. Marzetta, “Noncooperative cellular wireless with an unlimited number of base station antennas,” IEEE Trans. on Wireless Comm. , vol. 9, no. 11, pp. 3590-3600, Nov. 2010
[13]. L. Dai, J. Wang, Z. Wang, P. Tsiaflakis, and M. Moonen, “Spectrumand energy-efficient OFDM based on simultaneous multi-channel reconstruction,” IEEE Trans. Signal Process., vol. 61, no. 23, pp. 6047–6059, Dec. 2013.
[14]. Linglong Dai, Zhaocheng Wang,and Zhixing Yang.”Time-Frequency Training OFDM with High Spectral Efficiency and Reliable Performance in High Speed Environments..” IEEE Journal on Selected Areas in Communications 30.4(2012).
[15]. Z. Gao, L. Dai, and Z. Wang, “Structured compressive sensing based superimposed pilot design in downlink large-scale MIMO systems,” Electron. Lett., vol. 50, no. 12, pp. 896–898, 2014.
[16]. F. Rusek, D. Persson, B. K. Lau, E. G. Larsson, T. L. Marzetta, O. Edfors, and F. Tufvesson, “Scaling up MIMO: Opportunities and challenges with very large arrays,” IEEE Signal Process. Mag., vol. 30, no. 1, pp. 40–60, Jan. 2013.
[17]. Zhongxiu Hu, Hong Wang,and Rongfang Song.” MIMO signal detection algorithm based on SAMP-Net.” Journal of Nanjing University of Posts and Telecommunications (Natural Science Edition) 40.06(2020):36-41.
[18]. N. Samuel, T. Diskin and A. Wiesel, “Learning to Detect,” in IEEE Transactions on Signal Processing, vol. 67, no. 10, pp. 2554-2564, 15 May15, 2019.
[19]. H. Ye, G. Y. Li and B. -H. Juang, “Power of Deep Learning for Channel Estimation and Signal Detection in OFDM Systems,” in IEEE Wireless Communications Letters, vol. 7, no. 1, pp. 114-117, Feb. 2018.
[20]. J. Tachibana and T. Ohtsuki, “Learning and Analysis of Damping Factor in Massive MIMO Detection Using BP Algorithm With Node Selection,” in IEEE Access, vol. 8, pp. 96859-96866, 2020.
[21]. Xin Xing, et al. “Deep Learning Based Signal Detection for OFDM Satellite Communication Systems”.Proceedings of China Information and Communication Conference 2020 (CICC 2020).Ed. China Gongxin Publishing Group, People’s Posts & Telecom Press (Posts & Telecom Press), 2020, 400-404.
[22]. Sutar M B, Patil V S. LS and MMSE estimation with different fading channels for OFDM system[C]// International Conference of Electronics. 2017: 740-745.
Cite this article
Yang,Y. (2024). Signal detection algorithms for massive MIMO system. Applied and Computational Engineering,49,21-30.
Data availability
The datasets used and/or analyzed during the current study will be available from the authors upon reasonable request.
Disclaimer/Publisher's Note
The statements, opinions and data contained in all publications are solely those of the individual author(s) and contributor(s) and not of EWA Publishing and/or the editor(s). EWA Publishing and/or the editor(s) disclaim responsibility for any injury to people or property resulting from any ideas, methods, instructions or products referred to in the content.
About volume
Volume title: Proceedings of the 4th International Conference on Signal Processing and Machine Learning
© 2024 by the author(s). Licensee EWA Publishing, Oxford, UK. This article is an open access article distributed under the terms and
conditions of the Creative Commons Attribution (CC BY) license. Authors who
publish this series agree to the following terms:
1. Authors retain copyright and grant the series right of first publication with the work simultaneously licensed under a Creative Commons
Attribution License that allows others to share the work with an acknowledgment of the work's authorship and initial publication in this
series.
2. Authors are able to enter into separate, additional contractual arrangements for the non-exclusive distribution of the series's published
version of the work (e.g., post it to an institutional repository or publish it in a book), with an acknowledgment of its initial
publication in this series.
3. Authors are permitted and encouraged to post their work online (e.g., in institutional repositories or on their website) prior to and
during the submission process, as it can lead to productive exchanges, as well as earlier and greater citation of published work (See
Open access policy for details).