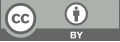
Generating high-quality images from brain EEG signals
- 1 The affiliated high school to jiangsu normal university
* Author to whom correspondence should be addressed.
Abstract
This study presents DreamDiffusion, an innovative approach to produce high-quality images straight from electroencephalogram (EEG) brain signals, eliminating the need for thought-to-text translation. By harnessing pre-trained text-to-image models, DreamDiffusion integrates temporal masked signal modeling to adeptly pre-train the EEG encoder, ensuring accurate and dependable EEG data representation. Moreover, by integrating the CLIP image encoder, this method fine-tunes the alignment of EEG, text, and image embeddings, even with a scant amount of EEG-image pairs. Effectively navigating the complexities inherent in EEG-based image creation, such as data noise, limited content, and personal variances, DreamDiffusion showcases promising outcomes. Both quantitative and qualitative assessments validate its efficacy, marking a considerable advancement in the realm of efficient, affordable "thought-to-image" conversions, with promising implications in both neuroscience and computer vision.
Keywords
High-Quality Images, Brain, EEG Signals, DreamDiffusion
[1]. Yunpeng Bai, Cairong Wang, Shuzhao Xie, Chao Dong, Chun Yuan, and Zhi Wang. Textir: A simple framework for text-based editable image restoration. arXiv preprint arXiv:2302.14736, 2023.
[2]. Suzanna Becker and Geoffrey E Hinton. Self-organizing neural network that discovers surfaces in random-dot stereograms. Nature, 355(6356):161–163, 1992.
[3]. Chris M Bird, Samuel C Berens, Aidan J Horner, and Anna Franklin. Categorical encoding of color in the brain. Proceedings of the National Academy of Sciences,111(12):4590–4595, 2014.
[4]. Andrew Brock, Jeff Donahue, and Karen Simonyan. Large scale gan training for high fidelity natural image synthesis. arXiv preprint arXiv:1809.11096, 2018.
[5]. Andrew Brock, Jeff Donahue, and Karen Simonyan. Large scale GAN training for high fidelity natural image synthesis. In 7th International Conference on Learning Representations, ICLR 2019, New Orleans, LA, USA, May 6-9, 2019. OpenReview.net, 2019.
[6]. Tom Brown, Benjamin Mann, Nick Ryder, Melanie Subbiah, Jared D Kaplan, Prafulla Dhariwal, Arvind Neelakantan, Pranav Shyam, Girish Sastry, Amanda Askell, et al. Language models are few-shot learners. Advances in neural information processing systems, 33:1877–1901, 2020.
[7]. Zijiao Chen, Jiaxin Qing, Tiange Xiang, Wan Lin Yue, and Juan Helen Zhou. Seeing beyond the brain: Conditional diffusion model with sparse masked modeling for vision decoding. arXiv preprint arXiv:2211.06956, 2022.
[8]. Keith M Davis, Carlos de la Torre-Ortiz, and Tuukka Ruotsalo. Brain-supervised image editing. In Proceedings of the IEEE/CVF Conference on Computer Vision and Pattern Recognition, pages 18480–18489, 2022.
[9]. Jacob Devlin, Ming-Wei Chang, Kenton Lee, and Kristina Toutanova. Bert: Pre-training of deep bidirectional transformers for language understanding. arXiv preprint arXiv:1810.04805, 2018.
Cite this article
Yang,D. (2024). Generating high-quality images from brain EEG signals. Applied and Computational Engineering,47,52-56.
Data availability
The datasets used and/or analyzed during the current study will be available from the authors upon reasonable request.
Disclaimer/Publisher's Note
The statements, opinions and data contained in all publications are solely those of the individual author(s) and contributor(s) and not of EWA Publishing and/or the editor(s). EWA Publishing and/or the editor(s) disclaim responsibility for any injury to people or property resulting from any ideas, methods, instructions or products referred to in the content.
About volume
Volume title: Proceedings of the 4th International Conference on Signal Processing and Machine Learning
© 2024 by the author(s). Licensee EWA Publishing, Oxford, UK. This article is an open access article distributed under the terms and
conditions of the Creative Commons Attribution (CC BY) license. Authors who
publish this series agree to the following terms:
1. Authors retain copyright and grant the series right of first publication with the work simultaneously licensed under a Creative Commons
Attribution License that allows others to share the work with an acknowledgment of the work's authorship and initial publication in this
series.
2. Authors are able to enter into separate, additional contractual arrangements for the non-exclusive distribution of the series's published
version of the work (e.g., post it to an institutional repository or publish it in a book), with an acknowledgment of its initial
publication in this series.
3. Authors are permitted and encouraged to post their work online (e.g., in institutional repositories or on their website) prior to and
during the submission process, as it can lead to productive exchanges, as well as earlier and greater citation of published work (See
Open access policy for details).