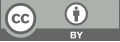
Translation from sketch to realistic photo based on CycleGAN
- 1 Georgia Institute of Technology
* Author to whom correspondence should be addressed.
Abstract
Forensic sketches serve as crucial tools for law enforcement agencies in identifying individuals of interest. However, their effectiveness can be limited due to constraints such as incomplete information and variations in interpretation by sketch artists, often rendering these sketches unrecognizable to the general public. In response to this challenge, this paper introduces an innovative approach—a CycleGAN-based image generation model. This model aims to transform monochrome forensic sketches into images with realistic colors and textures, offering an alternative visual representation that aids the public in identifying wanted individuals. The model is trained on unpaired datasets containing sketches and photographs of human faces, encompassing diverse scenarios. Through this training, it learns to generate images that closely resemble photographs captured in everyday environments. Impressively, the proposed model demonstrates rapid convergence, with both the generator and discriminator reaching optimal performance within just 500 epochs. Consequently, the generated images prove to be significantly more recognizable than the original sketches, thus enhancing the potential for successful identifications.
Keywords
Generative Adversarial Network, Image Generation, Image Translation
[1]. Ahuja A S et al 2023 The digital metaverse: Applications in artificial intelligence, medical education, and integrative health. Integrative Medicine Research 12(1) 100917
[2]. Qiu Y et al 2022 Pose-guided matching based on deep learning for assessing quality of action on rehabilitation training. Biomedical Signal Processing and Control 72 103323
[3]. Soori M Arezoo B and Dastres R 2023 Artificial intelligence, machine learning and deep learning in advanced robotics, A review Cognitive Robotics
[4]. Nikkath Bushra S and Uma Maheswari K 2021 Crime Investigation using DCGAN by Forensic Sketch-to-Face Transformation (STF)- A Review 2021 5th International Conference on Computing Methodologies and Communication (ICCMC) Erode India pp 1343-1348
[5]. Gunjate S Nakhate T Kshirsagar T and Sapat Y 2023 Sketch to Image using GAN International Journal of Innovative Science and Research Technology 8(1) pp 772–777
[6]. Chao W et al 2019 High-Fidelity Face Sketch-To-Photo Synthesis Using Generative Adversarial Network 2019 IEEE International Conference on Image Processing (ICIP) Taipei Taiwan 2019 pp 4699-4703
[7]. Xiaogang W and Xiaoou T 2005 Hallucinating face by eigentransformation in IEEE Transactions on Systems Man and Cybernetics Part C (Applications and Reviews) vol 35 no 3 pp 425-434 Aug
[8]. Kazemi H F et al 2018 Unsupervised facial geometry learning for sketch to photo synthesis in 2018 International Conference of the Biometrics Special Interest Group (BIOSIG) pp 1–5
[9]. Zhu J Park T Isola P and Efros A A 2017 Unpaired Image-to-Image Translation using Cycle-Consistent Adversarial Networks arXiv (Cornell University)
[10]. He K Zhang X Ren S and Sun J 2016 Deep Residual Learning for Image Recognition 2016 IEEE Conference on Computer Vision and Pattern Recognition
Cite this article
Yuan,X. (2024). Translation from sketch to realistic photo based on CycleGAN. Applied and Computational Engineering,50,41-45.
Data availability
The datasets used and/or analyzed during the current study will be available from the authors upon reasonable request.
Disclaimer/Publisher's Note
The statements, opinions and data contained in all publications are solely those of the individual author(s) and contributor(s) and not of EWA Publishing and/or the editor(s). EWA Publishing and/or the editor(s) disclaim responsibility for any injury to people or property resulting from any ideas, methods, instructions or products referred to in the content.
About volume
Volume title: Proceedings of the 4th International Conference on Signal Processing and Machine Learning
© 2024 by the author(s). Licensee EWA Publishing, Oxford, UK. This article is an open access article distributed under the terms and
conditions of the Creative Commons Attribution (CC BY) license. Authors who
publish this series agree to the following terms:
1. Authors retain copyright and grant the series right of first publication with the work simultaneously licensed under a Creative Commons
Attribution License that allows others to share the work with an acknowledgment of the work's authorship and initial publication in this
series.
2. Authors are able to enter into separate, additional contractual arrangements for the non-exclusive distribution of the series's published
version of the work (e.g., post it to an institutional repository or publish it in a book), with an acknowledgment of its initial
publication in this series.
3. Authors are permitted and encouraged to post their work online (e.g., in institutional repositories or on their website) prior to and
during the submission process, as it can lead to productive exchanges, as well as earlier and greater citation of published work (See
Open access policy for details).