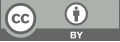
Prediction of patient breast cancer probability
- 1 Concordia University
* Author to whom correspondence should be addressed.
Abstract
Breast cancer has a significant global impact; in 2015, it caused 570,000 deaths and 1.5 million yearly diagnoses. A challenge is that it has a poor prognosis for cure and is metastatic. The 21st century's health-conscious atmosphere emphasizes the need to lower the death toll from cancer, which will account for approximately one in six fatalities in 2020. According to malignancy, an estimated 7.8 million women are projected to be diagnosed with breast cancer throughout the upcoming five-year period. For the identification and prevention of cancer, proactive measures are required. Python algorithms, particularly linear regression, are extraordinarily useful for analyzing complex datasets. Using linear regression in Python to analyze data yields illuminating models that reveal morbidity trends. With the insights gained from these models, healthcare providers can provide patients with more individualized care. This proactive approach and implementing Python's linear regression algorithms enhance the understanding of cancer risk and allow for effective preventative measures. Greater public awareness of health issues has resulted in an emphasis on preventative measures against breast cancer and other cancers. With the help of Python's data-driven algorithms, society may acquire a more accurate understanding of cancer risks and make decisions that will enhance patient welfare.
Keywords
Breast Cancer, Early Detection, Risk factors, Linear regression
[1]. Sun, Y. S., Zhao, Z., Yang, Z. 2017 Risk factors and preventions of breast cancer Inter. J. of Bio. Sci. 13(11) 1387
[2]. Misganaw, M., Zeleke, H., Mulugeta, H., and Assefa, B 2023 Mortality rate and predictors among patients with breast cancer at a referral hospital in northwest Ethiopia: A retrospective follow-up study Plos. One. 18(1) e0279656
[3]. Cappello, N. M., Richetelli, D., and Lee, C. I. 2019 The impact of breast density reporting laws on women’s awareness of density-associated risks and conversations regarding supplemental screening with providers J. Am. Coll. Radiol. 16(2) 139-146
[4]. Wiseman, M. J. 2019 Nutrition and cancer: prevention and survival Brit. J. Nutr. 122(5) 481-487
[5]. Shah, S., Mahamat-Saleh, Y., Ait-Hadad, W., Koemel, N. A., Varraso, R., Boutron-Ruault, M. C., and Laouali, N 2023 Long-term adherence to healthful and unhealthful plant-based diets and breast cancer risk overall and by hormone receptor and histologic subtypes among postmenopausal females The Am. J. of Clin. Nutr. 17(3) 467-476
[6]. Botlagunta, M., Botlagunta, M. D., Myneni, M. B., Lakshmi, D., Nayyar, A., Gullapalli, J. S., and Shah, M. A. 2023 Classification and diagnostic prediction of breast cancer metastasis on clinical data using machine learning algorithms Sci. Rep. 13(1) 485
[7]. Li, T., Nickel, B., Ngo, P., McFadden, K., Brennan, M., Marinovich, M. L., and Houssami, N. 2023 A systematic review of the impact of the COVID-19 pandemic on breast cancer screening and diagnosis The Breast
[8]. Torres-Román, J. S., Ybaseta-Medina, J., Loli-Guevara, S. 2023 Public Health Disparities in breast cancer mortality among Latin American women: trends and predictions for 2030 Y BMC 23(1) 1-9
[9]. Celeste Damiani, Grigios Kalliatakis, Muthyala Sreenivas, 13 June 2023 Evaluation of an AI model to assess future breast cancer risk Evaluation of an AI Model to Assess Future Breast Cancer Risk
[10]. Bertucci, F. and Birnbaum, D 2008 Reasons for breast cancer heterogeneity J. of bio. 7 1-4
Cite this article
Qiu,W. (2024). Prediction of patient breast cancer probability. Applied and Computational Engineering,47,207-212.
Data availability
The datasets used and/or analyzed during the current study will be available from the authors upon reasonable request.
Disclaimer/Publisher's Note
The statements, opinions and data contained in all publications are solely those of the individual author(s) and contributor(s) and not of EWA Publishing and/or the editor(s). EWA Publishing and/or the editor(s) disclaim responsibility for any injury to people or property resulting from any ideas, methods, instructions or products referred to in the content.
About volume
Volume title: Proceedings of the 4th International Conference on Signal Processing and Machine Learning
© 2024 by the author(s). Licensee EWA Publishing, Oxford, UK. This article is an open access article distributed under the terms and
conditions of the Creative Commons Attribution (CC BY) license. Authors who
publish this series agree to the following terms:
1. Authors retain copyright and grant the series right of first publication with the work simultaneously licensed under a Creative Commons
Attribution License that allows others to share the work with an acknowledgment of the work's authorship and initial publication in this
series.
2. Authors are able to enter into separate, additional contractual arrangements for the non-exclusive distribution of the series's published
version of the work (e.g., post it to an institutional repository or publish it in a book), with an acknowledgment of its initial
publication in this series.
3. Authors are permitted and encouraged to post their work online (e.g., in institutional repositories or on their website) prior to and
during the submission process, as it can lead to productive exchanges, as well as earlier and greater citation of published work (See
Open access policy for details).