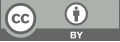
Analysis of implementation for advanced tools in sales prediction
- 1 Shenzhen College of International Education
* Author to whom correspondence should be addressed.
Abstract
As a matter of fact, since the 20th century, companies have started using statistical methods to predict the future sales of their product in order to adjust sales strategy as well as optimize the unitality of the corporations. In recent years, with the help of machine learning thanks to the rapid development of computation ability, firms could predict the future sales of their products with a much higher precision based on the state-of-art scenarios and models. To be specific, machine learning models such as Decision Trees help firms predict the trend of their sales with high efficiency. On this basis and with this in mind, this study will investigate the implementation and application of advanced tools in sales prediction. With the appearance of neuron networks such as LSTM-RNN, firms are now able to predict with high accuracy. According to the analysis, this study concludes the result of research carried out on Decision trees, GBDTs, as well as RNNs. At the same time, the current limitations and prospects are demonstrated and illustrated.
Keywords
Sales prediction, RNN, LSTM, DT, GBDT
[1]. Cheriyan S, Ibrahim S, Mohanan S and Treesa S 2018 Intelligent Sales Prediction Using Machine Learning Techniques International Conference on Computing Electronics and Communications Engineering (iCCECE) pp 53-58.
[2]. O'dell C and Grayson C J 1998 Food Sales Prediction: If Only It Knew What We Know. California management review vol 40(3) pp 154-174.
[3]. Wisesa O, Adriansyah A and Khalaf O I 2020 Prediction Analysis Sales for Corporate Services Telecommunications Company using Gradient Boost Algorithm 2nd International Conference on Broadband Communications Wireless Sensors and Powering (BCWSP) p 15.
[4]. Thomassey S and Fiordaliso A 2006 A hybrid sales forecasting system based on clustering and decision trees Decision Support Systems vol 42(1) pp 408–421.
[5]. Charbuty B and Abdulazeez A 2021 Classification Based on Decision Tree Algorithm for Machine LearningJournal of Applied Science and Technology Trends vol 2(01) pp 20-28.
[6]. Qian H, Wang B, Yuan M, Gao S and Song Y 2022 Financial distress prediction using a corrected feature selection measure and gradient boosted decision tree Expert Systems with Applications vol 190 p 116202.
[7]. Saxena P, Bahad P and Kamal R 2020 Long short-term memory-RNN based model for multivariate car sales forecasting Int J Adv Sci Technol vol 29 pp 4645-4656.
[8]. Zhou Y, Cheng Y, Lin Y and Mengqiu T 2021 IEEE International Conference on Consumer Electronics and Computer Engineering (ICCECE) p 21.
[9]. Deng T, Zhao Y, Wang S and Yu H 2021 Sales Forecasting Using GBDT Based Model And Data Mining Method IEEE International Conference on Consumer Electronics and Computer Engineering (ICCECE) pp 11-18.
[10]. Feng C and Chen Z D 2019 Sales Forecasting Based on LightGBM Computer Systems and Applications vol 28(10) pp 226-232.
[11]. Chandriah K K and Naraganahalli R V 2021 Application of Weighted Combination Model Based on XGBoost and LSTM in Sales Forecasting Multimedia Tools and Applications vol 80(17) pp 26145-26159.
Cite this article
Huang,Y. (2024). Analysis of implementation for advanced tools in sales prediction. Applied and Computational Engineering,55,55-60.
Data availability
The datasets used and/or analyzed during the current study will be available from the authors upon reasonable request.
Disclaimer/Publisher's Note
The statements, opinions and data contained in all publications are solely those of the individual author(s) and contributor(s) and not of EWA Publishing and/or the editor(s). EWA Publishing and/or the editor(s) disclaim responsibility for any injury to people or property resulting from any ideas, methods, instructions or products referred to in the content.
About volume
Volume title: Proceedings of the 4th International Conference on Signal Processing and Machine Learning
© 2024 by the author(s). Licensee EWA Publishing, Oxford, UK. This article is an open access article distributed under the terms and
conditions of the Creative Commons Attribution (CC BY) license. Authors who
publish this series agree to the following terms:
1. Authors retain copyright and grant the series right of first publication with the work simultaneously licensed under a Creative Commons
Attribution License that allows others to share the work with an acknowledgment of the work's authorship and initial publication in this
series.
2. Authors are able to enter into separate, additional contractual arrangements for the non-exclusive distribution of the series's published
version of the work (e.g., post it to an institutional repository or publish it in a book), with an acknowledgment of its initial
publication in this series.
3. Authors are permitted and encouraged to post their work online (e.g., in institutional repositories or on their website) prior to and
during the submission process, as it can lead to productive exchanges, as well as earlier and greater citation of published work (See
Open access policy for details).