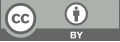
Keywords-based conditional image transformation
- 1 City University of Macau
* Author to whom correspondence should be addressed.
Abstract
In recent years, Generative Adversarial Networks (GANs) and their variants, such as pix2pix, have occupied a significant position in the field of image generation. Despite the impressive performance of the pix2pix model in image-to-image transformation tasks, its reliance on a large amount of paired training data and computational resources has posed a crucial constraint to its broader application. To address these issues, this paper introduces a novel algorithm, Keywords-Based Conditional Image Transformation (KB-CIT). KB-CIT dynamically extracts keywords from the input grayscale images to acquire and generate training data, thus avoiding the need for a large amount of paired data and significantly improving the efficiency of image transformation. Experimental results demonstrate that KB-CIT performs remarkably well in image colorization tasks and can generate high-quality colored images even with limited training data. This algorithm not only simplifies the data collection process but also exhibits significant advantages in terms of computational resource requirements, data utilization efficiency, and personalized real-time training of the model, thereby providing new possibilities for the widespread application of the pix2pix model.
Keywords
Generative Adversarial Network (GAN), pix2pix, Image Generation, Image Colorization
[1]. Goodfellow, I., Pouget-Abadie, J., Mirza, M., Xu, B., Warde-Farley, D., Ozair, S., Courville, A., & Bengio, Y. (2014). Generative Adversarial Nets. Advances in Neural Information Processing Systems, 27, 2672–2680
[2]. Creswell, A., White, T., Dumoulin, V., Arulkumaran, K., Sengupta, B., & Bharath, A. A. (2018). Generative Adversarial Networks: An Overview. IEEE Signal Processing Magazine, 35(1), 53–65
[3]. Wang, Z., She, Q., & Ward, T. E. (2020). Generative Adversarial Networks in Computer Vision: A Survey and Taxonomy (arXiv:1906.01529)
[4]. Wang, K., Gou, C., Duan, Y., Lin, Y., Zheng, X., & Wang, F.-Y. (2017). Generative adversarial networks: Introduction and outlook. IEEE/CAA Journal of Automatica Sinica, 4(4), 588–598
[5]. Radford, A., Metz, L., & Chintala, S. (2016). Unsupervised Representation Learning with Deep Convolutional Generative Adversarial Networks (arXiv:1511.06434)
[6]. Arjovsky, M., Chintala, S., & Bottou, L. (2017). Wasserstein Generative Adversarial Networks. Proceedings of the 34th International Conference on Machine Learning, 214–223
[7]. Denton, E. L., Chintala, S., szlam, arthur, & Fergus, R. (2015). Deep Generative Image Models using a Laplacian Pyramid of Adversarial Networks. Advances in Neural Information Processing Systems, 28, 1486–1494
[8]. Mirza, M., & Osindero, S. (2014). Conditional Generative Adversarial Nets (arXiv:1411.1784)
[9]. Isola, P., Zhu, J.-Y., Zhou, T., & Efros, A. A. (2017). Image-to-Image Translation with Conditional Adversarial Networks. 2017 IEEE Conference on Computer Vision and Pattern Recognition (CVPR), 5967–5976
[10]. Bousmalis, K., Irpan, A., Wohlhart, P., Bai, Y., Kelcey, M., Kalakrishnan, M., Downs, L., Ibarz, J., Pastor, P., Konolige, K., Levine, S., & Vanhoucke, V. (2018). Using Simulation and Domain Adaptation to Improve Efficiency of Deep Robotic Grasping. 2018 IEEE International Conference on Robotics and Automation (ICRA), 4243–4250
[11]. Zhu, J.-Y., Park, T., Isola, P., & Efros, A. A. (2017). Unpaired Image-to-Image Translation Using Cycle-Consistent Adversarial Networks. 2017 IEEE International Conference on Computer Vision (ICCV), 2242–2251
[12]. Nie, D., Trullo, R., Lian, J., Petitjean, C., Ruan, S., Wang, Q., & Shen, D. (2017). Medical Image Synthesis with Context-Aware Generative Adversarial Networks. Medical image computing and computer-assisted intervention : MICCAI ... International Conference on Medical Image Computing and Computer-Assisted Intervention, 10435, 417–425
[13]. Ronneberger, O., Fischer, P., & Brox, T. (2015). U-Net: Convolutional Networks for Biomedical Image Segmentation. In N. Navab, J. Hornegger, W. M. Wells, & A. F. Frangi (Eds.), Medical Image Computing and Computer-Assisted Intervention – MICCAI 2015 (pp. 234–241). Springer International Publishing
[14]. Vinyals, O., Toshev, A., Bengio, S., & Erhan, D. (2015). Show and Tell: A Neural Image Caption Generator (arXiv:1411.4555)
[15]. Anderson, P., He, X., Buehler, C., Teney, D., Johnson, M., Gould, S., & Zhang, L. (2018). Bottom-Up and Top-Down Attention for Image Captioning and Visual Question Answering. 2018 IEEE/CVF Conference on Computer Vision and Pattern Recognition, 6077–6086
[16]. Cornia, M., Stefanini, M., Baraldi, L., & Cucchiara, R. (2020). Meshed-Memory Transformer for Image Captioning. 2020 IEEE/CVF Conference on Computer Vision and Pattern Recognition (CVPR), 10575–10584
[17]. Xu, K., Ba, J., Kiros, R., Cho, K., Courville, A., Salakhudinov, R., Zemel, R., & Bengio, Y. (2015). Show, Attend and Tell: Neural Image Caption Generation with Visual Attention. Proceedings of the 32nd International Conference on Machine Learning, 2048–2057
[18]. You, Q., Jin, H., Wang, Z., Fang, C., & Luo, J. (2016). Image Captioning with Semantic Attention (arXiv:1603.03925)
[19]. Dosovitskiy, A., Beyer, L., Kolesnikov, A., Weissenborn, D., Zhai, X., Unterthiner, T., Dehghani, M., Minderer, M., Heigold, G., Gelly, S., Uszkoreit, J., & Houlsby, N. (2020, October 2). An Image is Worth 16x16 Words: Transformers for Image Recognition at Scale. International Conference on Learning Representations
[20]. Kingma, D. P., & Ba, J. (2017). Adam: A Method for Stochastic Optimization (arXiv:1412.6980)
Cite this article
Liu,J. (2024). Keywords-based conditional image transformation. Applied and Computational Engineering,57,56-65.
Data availability
The datasets used and/or analyzed during the current study will be available from the authors upon reasonable request.
Disclaimer/Publisher's Note
The statements, opinions and data contained in all publications are solely those of the individual author(s) and contributor(s) and not of EWA Publishing and/or the editor(s). EWA Publishing and/or the editor(s) disclaim responsibility for any injury to people or property resulting from any ideas, methods, instructions or products referred to in the content.
About volume
Volume title: Proceedings of the 6th International Conference on Computing and Data Science
© 2024 by the author(s). Licensee EWA Publishing, Oxford, UK. This article is an open access article distributed under the terms and
conditions of the Creative Commons Attribution (CC BY) license. Authors who
publish this series agree to the following terms:
1. Authors retain copyright and grant the series right of first publication with the work simultaneously licensed under a Creative Commons
Attribution License that allows others to share the work with an acknowledgment of the work's authorship and initial publication in this
series.
2. Authors are able to enter into separate, additional contractual arrangements for the non-exclusive distribution of the series's published
version of the work (e.g., post it to an institutional repository or publish it in a book), with an acknowledgment of its initial
publication in this series.
3. Authors are permitted and encouraged to post their work online (e.g., in institutional repositories or on their website) prior to and
during the submission process, as it can lead to productive exchanges, as well as earlier and greater citation of published work (See
Open access policy for details).