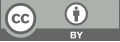
Bridging the gap in online hate speech detection: A comparative analysis of BERT and traditional models for homophobic content identification on X/Twitter
- 1 Department of Computer Science and Information Systems, University of Limerick
- 2 Department of Computer Science and Information Systems, University of Limerick
* Author to whom correspondence should be addressed.
Abstract
Our study addresses a significant gap in online hate speech detection research by focusing on homophobia, an area often neglected in sentiment analysis research. Utilising advanced sentiment analysis models, particularly BERT, and traditional machine learning methods, we developed a nuanced approach to identify homophobic content on X/Twitter. This research is pivotal due to the persistent underrepresentation of homophobia in detection models. Our findings reveal that while BERT outperforms traditional methods, the choice of validation technique can impact model performance. This underscores the importance of contextual understanding in detecting nuanced hate speech. By releasing the largest open-source labelled English dataset for homophobia detection known to us, an analysis of various models' performance and our strongest BERT-based model, we aim to enhance online safety and inclusivity. Future work will extend to broader LGBTQIA+ hate speech detection, addressing the challenges of sourcing diverse datasets. Through this endeavour, we contribute to the larger effort against online hate, advocating for a more inclusive digital landscape. Our study not only offers insights into the effective detection of homophobic content by improving on previous research results, but it also lays groundwork for future advancements in hate speech analysis.
Keywords
Homophobia detection, Sentiment analysis, Hate speech classification, Machine learning, Natural language processing (NLP), X/Twitter
[1]. Renkin, H. Z., & Trofimov, V. 2023. The Global Dialectics of Homonationalism and Homophobia. Sexuality & Culture, 27(6), 1987–95.
[2]. Jha, A., & Mamidi, R. 2017. When does a compliment become sexist? Analysis and classification of ambivalent sexism using twitter data. In D. Hovy, S. Volkova, D. Bamman, D. Jurgens, B. O’Connor, O. Tsur, et al. (Eds.), Proceedings of the Second Workshop on NLP and Computational Social Science, 7–16, Association for Computational Linguistics.
[3]. Kwok, I., & Wang, Y. 2013. Locate the Hate: Detecting Tweets against Blacks. Proceedings of the AAAI Conference on Artificial Intelligence, 27(1), 1621–2.
[4]. Gray, C., Russell, P., & Blockley, S. 1991. The effects upon helping behaviour of wearing pro-gay identification. British Journal of Social Psychology, 30(2), 171–178.
[5]. Glick, P., & Fiske, S. T. 1997. Hostile and benevolent sexism: Measuring ambivalent sexist attitudes toward women. Psychology of Women Quarterly, 21(1), 119–35.
[6]. Kumaresan, P. K., Ponnusamy, R., Priyadharshini, R., Buitelaar, P., & Chakravarthi, B. R. 2023. Homophobia and transphobia detection for low-resourced languages in social media comments. Natural Language Processing Journal, 5, 100041.
[7]. Fasoli, F., Paladino, M. P., Carnaghi, A., Jetten, J., Bastian, B., & Bain, P. G. 2016. Not “just words”: Exposure to homophobic epithets leads to dehumanizing and physical distancing from gay men. European Journal of Social Psychology, 46(2), 237–48.
[8]. Vigna, F. D., Cimino, A., Dell’Orletta, F., Petrocchi, M., & Tesconi, M. 2017. Hate Me, Hate Me Not: Hate Speech Detection on Facebook. In Proceedings of the First Italian Conference on Cybersecurity (ITASEC17), Venice, Italy, 86-95.
[9]. Tang, D., Qin, B., & Liu, T. 2015. Document Modeling with Gated Recurrent Neural Network for Sentiment Classification. In L. Màrquez, C. Callison-Burch, & J. Su (Eds.), Proceedings of the 2015 Conference on Empirical Methods in Natural Language Processing, 1422–32, Association for Computational Linguistics.
[10]. Gao, Z., Feng, A., Song, X., & Wu, X. 2019. Target-Dependent Sentiment Classification With BERT. IEEE Access, 7, 154290–9.
[11]. Rodriguez-Sanchez, F., Carrillo-de-Albornoz, J., & Plaza, L. 2020. Automatic Classification of Sexism in Social Networks: An Empirical Study on Twitter Data. IEEE Access, 8, 219563–76.
[12]. Devlin, J., Chang, M. W., Lee, K., & Toutanova, K. 2019. BERT: Pre-training of Deep Bidirectional Transformers for Language Understanding. In J. Burstein, C. Doran, & T. Solorio (Eds.), Proceedings of the 2019 Conference of the North American Chapter of the Association for Computational Linguistics: Human Language Technologies, Volume 1 (Long and Short Papers, 4171–86, Association for Computational Linguistics.
[13]. Wolf, T., Debut, L., Sanh, V., Chaumond, J., Delangue, C., Moi, A., et al. 2020. Transformers: State-of-the-Art Natural Language Processing. In Q. Liu & D. Schlangen (Eds.), Proceedings of the 2020 Conference on Empirical Methods in Natural Language Processing: System Demonstrations, 38–45, Association for Computational Linguistics.
Cite this article
McGiff,J.;Nikolov,N.S. (2024). Bridging the gap in online hate speech detection: A comparative analysis of BERT and traditional models for homophobic content identification on X/Twitter. Applied and Computational Engineering,64,63-68.
Data availability
The datasets used and/or analyzed during the current study will be available from the authors upon reasonable request.
Disclaimer/Publisher's Note
The statements, opinions and data contained in all publications are solely those of the individual author(s) and contributor(s) and not of EWA Publishing and/or the editor(s). EWA Publishing and/or the editor(s) disclaim responsibility for any injury to people or property resulting from any ideas, methods, instructions or products referred to in the content.
About volume
Volume title: Proceedings of the 6th International Conference on Computing and Data Science
© 2024 by the author(s). Licensee EWA Publishing, Oxford, UK. This article is an open access article distributed under the terms and
conditions of the Creative Commons Attribution (CC BY) license. Authors who
publish this series agree to the following terms:
1. Authors retain copyright and grant the series right of first publication with the work simultaneously licensed under a Creative Commons
Attribution License that allows others to share the work with an acknowledgment of the work's authorship and initial publication in this
series.
2. Authors are able to enter into separate, additional contractual arrangements for the non-exclusive distribution of the series's published
version of the work (e.g., post it to an institutional repository or publish it in a book), with an acknowledgment of its initial
publication in this series.
3. Authors are permitted and encouraged to post their work online (e.g., in institutional repositories or on their website) prior to and
during the submission process, as it can lead to productive exchanges, as well as earlier and greater citation of published work (See
Open access policy for details).