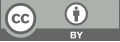
BERT-based cross-project and cross-version software defect prediction
- 1 The Hong Kong Polytechnic University
* Author to whom correspondence should be addressed.
Abstract
In recent years, deep learning-based software defect prediction has gained significant attention in software engineering research. This study aims to explore the application of the BERT model in the field of software defect detection. Traditional methods are constrained by manually designed rules and expert knowledge, which leads to limited accuracy and generalization ability. The strengths of deep learning methods lie in their capacity to capture complex semantic and contextual information in code. However, the effectiveness of deep learning models is hindered by the small scale of software defect datasets. To address this issue, we introduce BERT as a pre-trained model and construct a downstream task neural network, comprising a single-layer fully connected layer and a softmax classifier. Additionally, we evaluate four variants of BERT to enhance predictive performance. Through empirical studies on software defect prediction across different versions and projects, we find that utilizing the BERT pre-trained model significantly enhances predictive performance. The experimental results demonstrate that our model outperforms TextCNN by 8.99% in terms of AUC score and LSTM by 5.66%. In terms of the F1 score, our model surpasses TextCNN by 4.51% and LSTM by 15.57%. The primary contribution of this paper is the proposal of a cross-version and cross-project software defect prediction method, leveraging a lightweight BERT-based neural network. We also discuss the reasons for the observed variations in the performance of the four BERT variants during testing.
Keywords
software defect prediction, BERT model, deep learning
[1]. X. Sun, W. Zhou, B. Li, Z. Ni, and J. Lu, “Bug Localization for Version Issues With Defect Patterns,” IEEE Access, vol. 7, pp. 18811–18820, 2019, doi: 10.1109/ACCESS.2019.2894976.
[2]. Association for Computing Machinery, PROMISE : 7th International Conference on Predictive Models in Software Engineering : Banff, Canada, Sept 20-21, 2011 : co-located with ESEM 2011.
[3]. J. (Jean) Bézivin, J.-Marie. Favre, Bernhard. Rumpe, Association for Computing Machinery., and ACM Sigsoft., GaMMa ’06: : proceedings of the 2006 International Workshop on Global Integrated Model Management. Association for Computing Machinery, 2006.
[4]. T. J. Mccabe, “A Complexi-ty Measure,” 1976.
[5]. R. Harrison, S. J. Counsell, and R. V Nithi, “An Evaluation of the MOOD Set of Object-Oriented Software Metrics,” 1998.
[6]. M. Jureczko and D. D. Spinellis, “Using Object-Oriented Design Metrics to Predict Software Defects 1*.” [Online]. Available: http://gromit.iiar.pwr.wroc.pl/p_inf/ckjm
[7]. M. A. Elsabagh, M. S. Farhan, and M. G. Gafar, “Cross-projects software defect prediction using spotted hyena optimizer algorithm,” SN Appl Sci, vol. 2, no. 4, Apr. 2020, doi: 10.1007/s42452-020-2320-4.
[8]. A. B. Nasser et al., “A Robust Tuned K-Nearest Neighbours Classifier for Software Defect Prediction Lower Limb Exoskeleton View project Artificial Image processing based decision making for grading and sorting of rotatiinally symmetric products View project A Robust Tuned K-Nearest Neighbours Classifier for Software Defect Prediction.” [Online]. Available: https://www.researchgate.net/publication/362850366
[9]. H. A. Alhija, M. Azzeh, and F. Almasalha, “Software Defect Prediction Using Support Vector Machine.”
[10]. Y. Kim, “Convolutional Neural Networks for Sentence Classification,” Aug. 2014, [Online]. Available: http://arxiv.org/abs/1408.5882
[11]. “LSTM”.
[12]. T. B. Brown et al., “Language Models are Few-Shot Learners.” [Online]. Available: https://commoncrawl.org/the-data/
[13]. A. Vaswani et al., “Attention Is All You Need.”
[14]. J. Devlin, M.-W. Chang, K. Lee, and K. Toutanova, “BERT: Pre-training of Deep Bidirectional Transformers for Language Understanding,” Oct. 2018, [Online]. Available: http://arxiv.org/abs/1810.04805
[15]. M. Shepperd, Q. Song, Z. Sun, and C. Mair, “Data quality: Some comments on the NASA software defect datasets,” IEEE Transactions on Software Engineering, vol. 39, no. 9, pp. 1208–1215, 2013, doi: 10.1109/TSE.2013.11.
[16]. Gunes. Koru and Association for Computing Machinery., PROMISE 2010 : 6th International Conference on Predictive Models in Software Engineering : Timișoara, Romania, September 12-13, 2010. ACM, 2010.
[17]. T. Zhou, X. Sun, X. Xia, B. Li, and X. Chen, “Improving defect prediction with deep forest,” Inf Softw Technol, vol. 114, pp. 204–216, Oct. 2019, doi: 10.1016/j.infsof.2019.07.003.
[18]. C. Pan, M. Lu, and B. Xu, “An empirical study on software defect prediction using codebert model,” Applied Sciences (Switzerland), vol. 11, no. 11, Jun. 2021, doi: 10.3390/app11114793.
[19]. Z. Feng et al., “CodeBERT: A Pre-Trained Model for Programming and Natural Languages,” Feb. 2020, [Online]. Available: http://arxiv.org/abs/2002.08155
[20]. M. N. Uddin, B. Li, Z. Ali, P. Kefalas, I. Khan, and I. Zada, “Software defect prediction employing BiLSTM and BERT-based semantic feature,” Soft comput, vol. 26, no. 16, pp. 7877–7891, Aug. 2022, doi: 10.1007/s00500-022-06830-5.
Cite this article
Sun,B. (2024). BERT-based cross-project and cross-version software defect prediction. Applied and Computational Engineering,73,33-41.
Data availability
The datasets used and/or analyzed during the current study will be available from the authors upon reasonable request.
Disclaimer/Publisher's Note
The statements, opinions and data contained in all publications are solely those of the individual author(s) and contributor(s) and not of EWA Publishing and/or the editor(s). EWA Publishing and/or the editor(s) disclaim responsibility for any injury to people or property resulting from any ideas, methods, instructions or products referred to in the content.
About volume
Volume title: Proceedings of the 2nd International Conference on Software Engineering and Machine Learning
© 2024 by the author(s). Licensee EWA Publishing, Oxford, UK. This article is an open access article distributed under the terms and
conditions of the Creative Commons Attribution (CC BY) license. Authors who
publish this series agree to the following terms:
1. Authors retain copyright and grant the series right of first publication with the work simultaneously licensed under a Creative Commons
Attribution License that allows others to share the work with an acknowledgment of the work's authorship and initial publication in this
series.
2. Authors are able to enter into separate, additional contractual arrangements for the non-exclusive distribution of the series's published
version of the work (e.g., post it to an institutional repository or publish it in a book), with an acknowledgment of its initial
publication in this series.
3. Authors are permitted and encouraged to post their work online (e.g., in institutional repositories or on their website) prior to and
during the submission process, as it can lead to productive exchanges, as well as earlier and greater citation of published work (See
Open access policy for details).