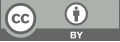
Robot learning-enhanced tree-based algorithms for kinodynamic motion planning: A comparative analysis
- 1 Columbia University
* Author to whom correspondence should be addressed.
Abstract
Kinodynamic motion planning is pivotal in advancing robotics, en- abling autonomous systems to navigate dynamic environments effectively while adhering to both kinematic and dynamic constraints. This study delves into the efficacy of tree sampling-based planners, namely the Rapidly- exploring Random Tree (RRT), Rapidly-exploring Random Tree Star (RRT*), and Dominance Informed Region Trees (DIRT), in kinodynamic motion planning. Through a comparative analysis focusing on both fully informed and uninformed versions of these algorithms, I explore their performance in environments with dynamic constraints. Special emphasis is placed on the integration of learned controls, aiming to enhance maneuver planning. My research reveals significant differences in success rates, iterations, and path costs among the algorithms, underscoring DIRT’s superiority under certain conditions and the beneficial impact of learned controls. These findings contribute valuable insights into the selection and optimization of motion planning algorithms, paving the way for more efficient and adapt- able autonomous systems.
Keywords
Kinodynamic Motion Planning, Tree Sampling-Based Plan- ners, RRT, RRT*, DIRT, Autonomous Systems
[1]. D. Silver et al. “Mastering Go with Deep Neural Networks and Tree Search”. In: Nature 529.7587 (2016), pp. 484–489.
[2]. J. Pan et al. “Motion Planning with Probabilistic Primitives”. In: 2012, pp. 3762–3768.
[3]. L. Jiang et al. “Path Planning in Multi-Obstacle Environment with ImprovedRRT ”. In: IEEE/ASME Trans. Mechatronics 27.6 (2022), pp. 4774–4785. DOI: 10.1109/TMECH.2022.3165845.
[4]. N. Lin et al. “Deep RL-Based Computation Offloading in Multi-UAV IoT Network”. In: IEEE IoT J. (2024). DOI: 10.1109/JIOT.2024.3356725.
[5]. X. Cui et al. “More Quickly-RRT*: Improved Quick RRT Star”. In: Eng. Appl. Artif. Intell. 133 (2024), p. 108246. DOI: 10.1016/j.engappai. 2024.108246.
[6]. R. I. Arnob and G. J. Stein. “Improving Navigation Under Uncertainty with Non-Local Predictions”. In: IEEE/IROS. 2023, pp. 2830–2837. DOI: 10.1109/IROS55552.2023.10342276.
[7]. L. Janson and M. Pavone. “Fast Marching Tree for Optimal Planning”. In: Int. J. Robotics Res. Vol. 34. 7. 2015, pp. 883–921.
[8]. S. Karaman and E. Frazzoli. “Sampling-based Optimal Motion Planning”. In: Int. J. Robotics Res. 30.7 (2011), pp. 846–894.
[9]. S. M. LaValle and J. J. Kuffner. “Randomized Kinodynamic Planning”. In: IEEE/ICRA. 2001, pp. 473–479.
[10]. Z. Littlefield and K. E. Bekris. “Efficient Kinodynamic Planning via Dominance- Informed Regions”. In: IEEE/IROS. 2018. DOI: 10.1109/IROS.2018. 8593672.
Cite this article
Hu,L. (2024). Robot learning-enhanced tree-based algorithms for kinodynamic motion planning: A comparative analysis. Applied and Computational Engineering,76,65-71.
Data availability
The datasets used and/or analyzed during the current study will be available from the authors upon reasonable request.
Disclaimer/Publisher's Note
The statements, opinions and data contained in all publications are solely those of the individual author(s) and contributor(s) and not of EWA Publishing and/or the editor(s). EWA Publishing and/or the editor(s) disclaim responsibility for any injury to people or property resulting from any ideas, methods, instructions or products referred to in the content.
About volume
Volume title: Proceedings of the 2nd International Conference on Software Engineering and Machine Learning
© 2024 by the author(s). Licensee EWA Publishing, Oxford, UK. This article is an open access article distributed under the terms and
conditions of the Creative Commons Attribution (CC BY) license. Authors who
publish this series agree to the following terms:
1. Authors retain copyright and grant the series right of first publication with the work simultaneously licensed under a Creative Commons
Attribution License that allows others to share the work with an acknowledgment of the work's authorship and initial publication in this
series.
2. Authors are able to enter into separate, additional contractual arrangements for the non-exclusive distribution of the series's published
version of the work (e.g., post it to an institutional repository or publish it in a book), with an acknowledgment of its initial
publication in this series.
3. Authors are permitted and encouraged to post their work online (e.g., in institutional repositories or on their website) prior to and
during the submission process, as it can lead to productive exchanges, as well as earlier and greater citation of published work (See
Open access policy for details).