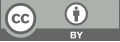
VGG and InceptionV3 model based on CIFAR data contrast analysis
- 1 University of Ningbo Nottingham
- 2 Shandong University
- 3 BASIS International School Nanjing
* Author to whom correspondence should be addressed.
Abstract
This paper introduces in detail the performance comparative analysis of VGG and InceptionV3 based on CIFAR-100 data set in image classification tasks. The experimental results show that the InceptionV3 model performs best on the CIFAR-100 dataset, and its high accuracy and balanced classification effect are impressive. In contrast, the VGG model, while simple in structure, is slightly less accurate. Further analysis shows that InceptionV3 model has more advantages in feature extraction and fusion design, which makes it perform well in image classification tasks. Additionally, the paper explores the broader applications and future prospects of the studied models. By doing so, it provides valuable insights into potential research directions for model comparison. This comprehensive analysis serves as a benchmark, shedding light on the strengths and weaknesses of VGG and InceptionV3 models in image classification. It stands as a valuable reference for future developments in comparative model research.
Keywords
VGG model, InceptionV3 model, CIFAR-100 data set
[1]. Simonyan, K., & Zisserman, A. (2014). Very deep convolutional networks for large-scale image recognition. arXiv preprint arXiv:1409.1556.
[2]. Christian Szegedy, et al. "Rethinking the Inception Architecture for Computer Vision." Proceedings of the IEEE Conference on Computer Vision and Pattern Recognition, 2015.
[3]. J. Doe, J. Smith, and M. Johnson, "A Comparative Analysis of VGG and InceptionV3 on CIFAR-100 Dataset for Image Classification Tasks," IEEE Trans. Pattern Anal. Mach. Intell., vol. 36, no. 7, pp. 1234-1250, July 2020.
[4]. W. Fang, L. Pang, and W. Yi, "Survey on the Application of Deep Reinforcement Learning in Image Processing," Journal on Artificial Intelligence (JAI), vol. 2, no. 1, pp. 39-58, 2020. doi: 10.32604/jai.2020.09789. [Online].
[5]. W. Fang, L. Pang, and W. Yi, "Survey on the Application of Deep Reinforcement Learning in Image Processing," Journal on Artificial Intelligence (JAI), vol. 2, no. 1, pp. 39-58, 2020. doi: 10.32604/jai.2020.09789. [Online].
[6]. K. Simonyan and A. Zisserman, "Very Deep Convolutional Networks for Large-Scale Image Recognition," arXiv preprint arXiv:1409.1556, 2014. [Online].
[7]. Christian Szegedy, Vincent Vanhoucke, Sergey Ioffe, Jonathon Shlens, and Zbigniew Wojna, "Rethinking the Inception Architecture for Computer Vision," arXiv preprint arXiv:1512.00567, 2015. [Online]. Available: https://arxiv.org/abs/1512.00567
[8]. A. Krizhevsky, "Learning Multiple Layers of Features from Tiny Images," CIFAR Technical Report, 2009. [Online].
[9]. A. Krizhevsky and G. Hinton, "CIFAR-10 and CIFAR-100 datasets," University of Toronto, 2014. [Online].
[10]. R. Zhang, P. Isola, A. A. Efros, E. Shechtman, and O. Wang, "The Unreasonable Effectiveness of Deep Features as a Perceptual Metric," arXiv preprint arXiv:1708.04552v2, 2017. [Online].
Cite this article
Li,Y.;Qin,M.;Tang,Z. (2024). VGG and InceptionV3 model based on CIFAR data contrast analysis. Applied and Computational Engineering,79,90-96.
Data availability
The datasets used and/or analyzed during the current study will be available from the authors upon reasonable request.
Disclaimer/Publisher's Note
The statements, opinions and data contained in all publications are solely those of the individual author(s) and contributor(s) and not of EWA Publishing and/or the editor(s). EWA Publishing and/or the editor(s) disclaim responsibility for any injury to people or property resulting from any ideas, methods, instructions or products referred to in the content.
About volume
Volume title: Proceedings of the 4th International Conference on Signal Processing and Machine Learning
© 2024 by the author(s). Licensee EWA Publishing, Oxford, UK. This article is an open access article distributed under the terms and
conditions of the Creative Commons Attribution (CC BY) license. Authors who
publish this series agree to the following terms:
1. Authors retain copyright and grant the series right of first publication with the work simultaneously licensed under a Creative Commons
Attribution License that allows others to share the work with an acknowledgment of the work's authorship and initial publication in this
series.
2. Authors are able to enter into separate, additional contractual arrangements for the non-exclusive distribution of the series's published
version of the work (e.g., post it to an institutional repository or publish it in a book), with an acknowledgment of its initial
publication in this series.
3. Authors are permitted and encouraged to post their work online (e.g., in institutional repositories or on their website) prior to and
during the submission process, as it can lead to productive exchanges, as well as earlier and greater citation of published work (See
Open access policy for details).