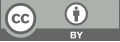
Emerging synergies between large language models and machine learning in e-commerce recommendations
- 1 Independent Researcher, Northern Arizona University, Flagstaff, AZ, USA,86011
- 2 Computer Science, Northeastern University, Boston, MA,02115
- 3 Information Systems, Northeastern University, San Jose, CA,95110
- 4 Computer Science and Technology, Tianjin University of Technology, Tianjin,CN,300384
- 5 Financial Mathematics, University of Southern California, Los Angeles, USA,90089
* Author to whom correspondence should be addressed.
Abstract
This paper explores the integration of large language models (LLMs) into collaborative filtering algorithms to enhance recommendation systems in the e-commerce domain. The proposed approach combines user-based and item-based collaborative filtering with LLMs to improve recommendation accuracy and personalization. Specifically, the study introduces a novel framework called PALR, which leverages LLMs to refine user-item interactions and enrich item representations. PALR utilizes historical user behavior data, such as clicks, purchases, and ratings, to guide candidate retrieval and generate recommended items. This study highlights the importance of integrating LLMs into recommendation systems to deliver more accurate and personalized suggestions, ultimately improving user satisfaction and driving sales in e-commerce platforms.
Keywords
Large language models, collaborative filtering, recommendation systems, e-commerce
[1]. Emanuele Barabino,and Giuseppe Cittadini. "From Search Engines to Large Language Models: A Big Leap for Patient Education!." Cardiovascular and interventional radiology (2024):
[2]. Liu, Bo, et al. "Integration and Performance Analysis of Artificial Intelligence and Computer Vision Based on Deep Learning Algorithms." arXiv preprint arXiv:2312.12872 (2023).
[3]. Zhiyu Li, et al. "BookGPT: A General Framework for Book Recommendation Empowered by Large Language Model." Electronics 12. 22 (2023):
[4]. Yu, L., Liu, B., Lin, Q., Zhao, X., & Che, C. (2024). Semantic Similarity Matching for Patent Documents Using Ensemble BERT-related Model and Novel Text Processing Method. arXiv preprint arXiv:2401.06782.
[5]. Liu, Bo, et al. "Integration and Performance Analysis of Artificial Intelligence and Computer Vision Based on Deep Learning Algorithms." arXiv preprint arXiv:2312.12872 (2023).
[6]. Agathokleous Evgenios, et al. "One hundred important questions facing plant science derived using a large language model.." Trends in plant science (2023):
[7]. Møller Lynge Asbjørn. "Recommended for You: How Newspapers Normalise Algorithmic News Recommendation to Fit Their Gatekeeping Role." Journalism Studies 23. 7 (2022):
[8]. Yu, Liqiang, et al. “Research on Machine Learning With Algorithms and Development”. Journal of Theory and Practice of Engineering Science, vol. 3, no. 12, Dec. 2023, pp. 7-14, doi:10.53469/jtpes.2023.03(12).02.
[9]. Huang, J., Zhao, X., Che, C., Lin, Q., & Liu, B. (2024). Enhancing Essay Scoring with Adversarial Weights Perturbation and Metric-specific AttentionPooling. arXiv preprint arXiv:2401.05433.
[10]. Tan, Kai, et al. “Integrating Advanced Computer Vision and AI Algorithms for Autonomous Driving Systems”. Journal of Theory and Practice of Engineering Science, vol. 4, no. 01, Jan. 2024, pp. 41-48, doi:10.53469/jtpes.2024.04(01).06.
[11]. Liu, B., Zhao, X., Hu, H., Lin, Q., & Huang, J. (2023). Detection of Esophageal Cancer Lesions Based on CBAM Faster R-CNN. Journal of Theory and Practice of Engineering Science, 3(12), 36–42. https://doi.org/10.53469/jtpes.2023.03(12).06
Cite this article
Xu,X.;Wu,Y.;Liang,P.;He,Y.;Wang,H. (2024). Emerging synergies between large language models and machine learning in e-commerce recommendations. Applied and Computational Engineering,69,57-63.
Data availability
The datasets used and/or analyzed during the current study will be available from the authors upon reasonable request.
Disclaimer/Publisher's Note
The statements, opinions and data contained in all publications are solely those of the individual author(s) and contributor(s) and not of EWA Publishing and/or the editor(s). EWA Publishing and/or the editor(s) disclaim responsibility for any injury to people or property resulting from any ideas, methods, instructions or products referred to in the content.
About volume
Volume title: Proceedings of the 6th International Conference on Computing and Data Science
© 2024 by the author(s). Licensee EWA Publishing, Oxford, UK. This article is an open access article distributed under the terms and
conditions of the Creative Commons Attribution (CC BY) license. Authors who
publish this series agree to the following terms:
1. Authors retain copyright and grant the series right of first publication with the work simultaneously licensed under a Creative Commons
Attribution License that allows others to share the work with an acknowledgment of the work's authorship and initial publication in this
series.
2. Authors are able to enter into separate, additional contractual arrangements for the non-exclusive distribution of the series's published
version of the work (e.g., post it to an institutional repository or publish it in a book), with an acknowledgment of its initial
publication in this series.
3. Authors are permitted and encouraged to post their work online (e.g., in institutional repositories or on their website) prior to and
during the submission process, as it can lead to productive exchanges, as well as earlier and greater citation of published work (See
Open access policy for details).