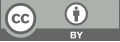
Application of large language models based on knowledge graphs in question-answering systems: A review
- 1 School of Mathematics and Computer Science, Northwest Minzu University, Chengguan District, Lanzhou, 730030, China
* Author to whom correspondence should be addressed.
Abstract
The integration of Knowledge Graphs (KGs) and Large Language Models (LLMs) is emerging as a transformative advancement in AI, particularly within Question Answering (QA) systems. Traditional QA systems, constrained by static knowledge bases, have struggled with multimodal queries and personalized responses. The deep integration of KGs and LLMs offers a novel approach, combining the structured, contextual understanding of KGs with the semantic parsing capabilities of LLMs. This review explores the methodologies, algorithms, datasets, and applications of KG-LLM integration in QA systems, highlighting key research that enhances model performance, reasoning, and domain-specific applications. It addresses technical challenges such as data consistency, semantic understanding, and system optimization. Future directions focus on improving intrinsic model performance and achieving deeper integration of KGs and LLMs. This comprehensive overview underscores the potential of KG-LLM synergy to significantly improve service quality across diverse industries, including education and healthcare.
Keywords
Knowledge Graphs, KGs Large Language Models, LLMs Question Answering
[1]. Jovanović, M., & Campbell, M. (2023). Connecting AI: Merging large language models and knowledge graph. Computer, 56(11), 103-108.
[2]. Yasunaga, M., Bosselut, A., Ren, H. Y., et al. (2021). QA-GNN: Reasoning with language models and knowledge graphs for question answering. arXiv:2104.06378.
[3]. Hu, N., Wu, Y., Qi, G., Min, D., Chen, J., Pan, J. Z., & Ali, Z. (2023). An empirical study of pre-trained language models in simple knowledge graph question answering. World Wide Web, 26(5), 2855-2886.
[4]. Wu, Q., & Wang, Y. (2023). Research on intelligent question-answering systems based on large language models and knowledge graphs. 2023 16th International Symposium on Computational Intelligence and Design (ISCID). IEEE.
[5]. Shannon, C. E. (1948). A mathematical theory of communication. Bell System Technical Journal, 27(3), 379-423.
[6]. Page, L., & Brin, S. (1998). The PageRank citation ranking: Bringing order to the web. Stanford Information Sciences and Engineering Report, 98-03.
[7]. Devlin, J., Chang, M., Lee, K., et al. (2019). BERT: Pre-training of deep bidirectional transformers for language understanding. In Proceedings of the 2019 Conference of the North American Chapter of the Association for Computational Linguistics: Human Language Technologies (pp. 4171-4186). Stroudsburg, PA: Association for Computational Linguistics.
[8]. Li, X. X., Zhao, R. C., Chia, Y. K., et al. (2023). Chain of knowledge: A framework for grounding large language models with structured knowledge bases. arXiv:2305.13269.
[9]. Taffa, T. A., & Usbeck, R. (2023). Leveraging LLMs in scholarly knowledge graph question answering. arXiv:2311.09841.
[10]. Zhang, Y. C., Chen, Z., Fang, Y., et al. (2023). Knowledgeable preference alignment for LLMs in domain-specific question answering. arXiv:2311.06503.
[11]. Agarwal, D., Das, R., Khosla, S., et al. (2023). Bring your own KG: Self-supervised program synthesis for zero-shot KGQA. arXiv:2311.12788.
[12]. Jiang, P. C., Xiao, C., Cross, A., et al. (2023). GraphCare: Enhancing healthcare predictions with personalized knowledge graphs. arXiv:2305.12788.
[13]. Wan, Z., Cheng, F., Mao, Z. Y., et al. (2023). GPT-RE: In-context learning for relation extraction using large language models. arXiv:2305.02105.
[14]. Zhu, Y. H., Ren, C. Y., Xie, S. Y., et al. (2023). REALM: RAG driven enhancement of multimodal.
[15]. Li, X., Henriksson, A., & Duneld, J. W. Y. (2024). Evaluating embeddings from pre-trained language models and knowledge graphs for educational content recommendation. Future Internet, 16(1).
[16]. Yang, X., Yumer, E., Asente, P., Kraley, M., Kifer, D., & Lee Giles, C. (2017). Learning to extract semantic structure from documents using multimodal fully convolutional neural networks. In Proceedings of the IEEE conference on computer vision and pattern recognition (pp. 5315-5324).
[17]. Zhang, Q. G., Dong, J. N., Chen, H., et al. (2024). KnowGPT: Black-box knowledge injection for large language models. arXiv:2312.06185.
[18]. Sun, Y. Q., Shi, Q., Qi, L., et al. (2021). JointLK: Joint reasoning with language models and knowledge graphs for commonsense question answering. arXiv:2112.15027.
[19]. Yasunaga, M., Ren, H., Bosselut, A., Liang, P., & Leskovec, J. (2021). QA-GNN: Reasoning with language models and knowledge graphs for question answering. arXiv:2104.06378.
[20]. Yasunaga, M., Bosselut, A., Ren, H. Y., et al. (2022). Deep bidirectional language-knowledge graph pretraining. Advances in Neural Information Processing Systems, 35, 37309-37323.
Cite this article
Wang,Y. (2024). Application of large language models based on knowledge graphs in question-answering systems: A review. Applied and Computational Engineering,71,78-82.
Data availability
The datasets used and/or analyzed during the current study will be available from the authors upon reasonable request.
Disclaimer/Publisher's Note
The statements, opinions and data contained in all publications are solely those of the individual author(s) and contributor(s) and not of EWA Publishing and/or the editor(s). EWA Publishing and/or the editor(s) disclaim responsibility for any injury to people or property resulting from any ideas, methods, instructions or products referred to in the content.
About volume
Volume title: Proceedings of the 6th International Conference on Computing and Data Science
© 2024 by the author(s). Licensee EWA Publishing, Oxford, UK. This article is an open access article distributed under the terms and
conditions of the Creative Commons Attribution (CC BY) license. Authors who
publish this series agree to the following terms:
1. Authors retain copyright and grant the series right of first publication with the work simultaneously licensed under a Creative Commons
Attribution License that allows others to share the work with an acknowledgment of the work's authorship and initial publication in this
series.
2. Authors are able to enter into separate, additional contractual arrangements for the non-exclusive distribution of the series's published
version of the work (e.g., post it to an institutional repository or publish it in a book), with an acknowledgment of its initial
publication in this series.
3. Authors are permitted and encouraged to post their work online (e.g., in institutional repositories or on their website) prior to and
during the submission process, as it can lead to productive exchanges, as well as earlier and greater citation of published work (See
Open access policy for details).