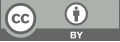
ADHD fMRI classification through CBAM-Autoencoder framework
- 1 Sichuan University
* Author to whom correspondence should be addressed.
Abstract
This work presents a novel method for leveraging resting-state functional magnetic resonance imaging (fMRI) data to accurately detect Attention Deficit Hyperactivity Disorder (ADHD). The proposed method integrates the Convolutional Block Attention Module (CBAM) with a lightweight Autoencoder network to effectively extract and highlight salient features within fMRI scans. By leveraging attention mechanisms, the model focuses on important local details while filtering out irrelevant information, thereby enhancing diagnostic precision. Extensive experimentation on the ADHD-200 dataset showcases the efficacy of the proposed approach, demonstrating its ability to improve classification performance significantly. Specifically, the method achieved an average accuracy of 91.7% across the NYU, 93.8% across the KKI, 86.4% across the NI, 89.1% across the PU, and 83.5% across the PU_1 datasets. This research underscores the potential of attention-based deep learning techniques in advancing ADHD diagnosis using neuroimaging data.
Keywords
Convolutional Block Attention Module, Autoencoder, Deep Learning, Attention Deficit Hyperactivity Disorder, fMRI
[1]. American Psychiatric Association. (2013). Diagnostic and Statistical Manual of Mental Disorders: DSM-5 (5th ed.). Arlington, VA: American Psychiatric Association.
[2]. Tamam, L., Karakus, G., & Ozpoyraz, N. (2008). Comorbidity of adult attention-deficit hyperactivity disorder and bipolar disorder: Prevalence and clinical correlates. European Archives of Psychiatry and Clinical Neuroscience, 258, 385–393. https://doi.org/10.1007/s00406-008-0807-x.
[3]. Klassen, L. J., Katzman, M. A., & Chokka, P. (2009). Adult ADHD and its comorbidities, with a focus on bipolar disorder. Journal of Affective Disorders, 124(1–2), 1–8.
[4]. Conners, C. K., Pitkanen, J., & Rzepa, S. R. (2011). Conners (Conners 3; Conners 2008) (3rd ed.). New York, NY, USA: Springer. pp. 675-678.
[5]. Loh, H. W., Ooi, C. P., Barua, P. D., Palmer, E. E., Molinari, F., & Acharya, U. R. (2022). Automated detection of ADHD: Current trends and future perspective. Computers in Biology and Medicine, 146, 105525. doi: 10.1016/j.compbiomed.2022.105525.
[6]. Milham, M. P., Fair, D., Mennes, M., & Mostofsky, S. H. (2012). The ADHD-200 consortium: A model to advance the translational potential of neuroimaging in clinical neuroscience. Frontiers in Systems Neuroscience, 6, 62.
[7]. Huettel, S. A., Song, A. W., & McCarthy, G. (2009). Functional Magnetic Resonance Imaging. Yale Journal of Biology and Medicine, 82(4), 233.
[8]. Eslami, T., Almuqhim, F., Raiker, J. S., & Saeed, F. (2021). Machine learning methods for diagnosing autism spectrum disorder and attention-deficit/hyperactivity disorder using functional and structural MRI: A survey. Frontiers in Neuroinformatics, 14. doi: 10.3389/fninf.2020.623543.
[9]. Dey, S., Rao, A. R., & Shah, M. (2014). Attributed graph distance measure for automatic detection of attention deficit hyperactive disordered subjects. Frontiers in Neural Circuits, 8. https://doi.org/10.3389/fncir.2014.00064.
[10]. Colby, J., Rudie, J., Brown, J., Douglas, P., Cohen, M., & Shehzad, Z. (2012). Insights into multimodal imaging classification of ADHD. Frontiers in Systems Neuroscience, 6. https://doi.org/10.3389/fnsys.2012.00059
[11]. Riaz, A., Alonso, E., & Slabaugh, G. (2016). Phenotypic Integrated Framework for Classification of ADHD Using fMRI Image Analysis and Recognition. In Proceedings of the International Conference on Medical Image Computing and Computer-Assisted Intervention (MICCAI), Volume 9730 (pp. [page range]). ISBN: 978-3-319-41500-0.
[12]. Riaz, A., Asad, M., Alonso, E., & Slabaugh, G. (2018). Fusion of fMRI and non-imaging data for ADHD classification. Computerized Medical Imaging and Graphics, 65, 115–128.
[13]. Riaz, A., Asad, M., Alonso, E., & Slabaugh, G. (2018). Fusion of fMRI and non-imaging data for ADHD classification. Computerized Medical Imaging and Graphics, 65, 115–128.
[14]. Miao, B., Zhang, L. L., Guan, J. L., Meng, Q. F. M., & Zhang, Y. L. (2019). Classification of ADHD individuals and neurotypicals using reliable RELIEF: A resting-state study. IEEE Access, 7, 62163–62171.
[15]. Zou, L., Zheng, J., Miao, C., McKeown, M. J., & Wang, Z. J. (2017). 3D CNN Based Automatic Diagnosis of Attention Deficit Hyperactivity Disorder Using Functional and Structural MRI. IEEE Access, 5, 23626-23636. doi: 10.1109/ACCESS.2017.2762703.
[16]. Mao, Z., Su, Y., Xu, G., Wang, X., Huang, Y., Yue, W., Sun, L., & Xiong, N. (2019). Spatio-temporal deep learning method for ADHD fMRI classification. Information Sciences, 499, 1-11. https://doi.org/10.1016/j.ins.2019.05.043.
[17]. Riaz, A., Asad, M., Alonso, E., & Slabaugh, G. (2020). DeepFMRI: End-to-end deep learning for functional connectivity and classification of ADHD using fMRI. Journal of Neuroscience Methods, 335, 108506.
[18]. Zhang, J., Zhou, L., Wang, L., Liu, M., & Shen, D. (2022). Diffusion Kernel Attention Network for Brain Disorder Classification. IEEE Transactions on Medical Imaging, 41(10), 2814-2827. doi: 10.1109/TMI.2022.3170701.
[19]. Qiang, N., Dong, Q., Liang, H., et al. (2022). A novel ADHD classification method based on resting state temporal templates (RSTT) using spatiotemporal attention auto-encoder. Neural Computing & Applications, 34, 7815–7833. https://doi.org/10.1007/s00521-021-06868-w.
[20]. Woo, S., Park, J., Lee, J. Y., & Kweon, I. S. (2018). Cbam: Convolutional block attention module. In Proceedings of the European conference on computer vision (ECCV) (pp. 3-19).
Cite this article
Hong,S. (2024). ADHD fMRI classification through CBAM-Autoencoder framework. Applied and Computational Engineering,88,12-19.
Data availability
The datasets used and/or analyzed during the current study will be available from the authors upon reasonable request.
Disclaimer/Publisher's Note
The statements, opinions and data contained in all publications are solely those of the individual author(s) and contributor(s) and not of EWA Publishing and/or the editor(s). EWA Publishing and/or the editor(s) disclaim responsibility for any injury to people or property resulting from any ideas, methods, instructions or products referred to in the content.
About volume
Volume title: Proceedings of the 6th International Conference on Computing and Data Science
© 2024 by the author(s). Licensee EWA Publishing, Oxford, UK. This article is an open access article distributed under the terms and
conditions of the Creative Commons Attribution (CC BY) license. Authors who
publish this series agree to the following terms:
1. Authors retain copyright and grant the series right of first publication with the work simultaneously licensed under a Creative Commons
Attribution License that allows others to share the work with an acknowledgment of the work's authorship and initial publication in this
series.
2. Authors are able to enter into separate, additional contractual arrangements for the non-exclusive distribution of the series's published
version of the work (e.g., post it to an institutional repository or publish it in a book), with an acknowledgment of its initial
publication in this series.
3. Authors are permitted and encouraged to post their work online (e.g., in institutional repositories or on their website) prior to and
during the submission process, as it can lead to productive exchanges, as well as earlier and greater citation of published work (See
Open access policy for details).