1. Introduction
With the rapidly development of Internet, the number of information and products that users can access has sharply increased. How to find content that users are interested in from massive amounts of messages has become a challenge. These days, a growing number of information overload problems in fields such as entertainment, social media, and e-commerce can be solved by Recommendation systems (RS). In current research, most of the RSs are designed based on machine learning algorithms and techniques. Machine learning (ML) based RSs have been applied to various kinds of Internet content platforms. Therefore, it is meaningful to summarize the research results of existing ML and RS design for future innovative development. This paper aims to comprehensively discuss and analyze recent research results, in order to provide inspiration and ideas for future researchers.
The ML-based RS can collect and analyze user behavior data on the Internet, such as click, browse, purchase, score, etc. Based on the learning of these behavioral data, it can accurately summarize user behavior and preferences, thus recommending personalized content to users. Researchers in this field broadly classify machine learning algorithms into four directions: K-Nearest Neighbor (K-NN), Content-based Recommendation (CR), Collaborative Filtering (CF), Hybrid Filtering (HF) [1]. These algorithms respectively serve the push of relevant information and information filtering. With the continuous updating of technology, ML has extended into a subfield called deep learning (DL). Its main innovation lies in the study of using multi-layer neural networks to analyze and predict complex data. In recent years, many researchers have focused on the application of DL in research fields such as computer vision and natural language processing. As claimed by previous researchers, DL not only has excellent performance, but also provides a way to learn feature representations from scratch [2]. This is due to its imitation of the neural network structure of the human brain, which learns the complex patterns and features of data samples through a multi-layer network structure. Previous researchers have proposed advanced algorithms and models for personalized customization and information filtering to optimize the performance of RSs.
The primary objective of this article is to organize and summarize the key concepts and background of ML-based information systems. A significant focus will be on DL as an essential module within ML. This article delves into the core technologies of DL, exploring its fundamental principles. Additionally, it includes an analysis of the experimental performance of key technologies related to similarity algorithms and information filtering models. Throughout this article, the design of RS for the modern Internet is discussed as a relatively mature field. While there are still some limitations in terms of accuracy, many researchers believe that it represents a highly innovative direction for future development.
This section provides a general introduction to the entire article. Section 2 analyzes the core concepts and principles of machine learning and deep learning methods. Then, in section 3, an analysis and discussion were conducted on the relevant experimental results mentioned in the review. The fourth section will summarize and generalize the professional knowledge involved in this article.
2. Methodology
2.1. Dataset Description and Preprocessing
The most widely used dataset in recommendation system research is MovieLens. It provides multiple versions of the dataset, which contains a large amount of user rating data for movies. This includes basic information of users, basic information of movies, ratings and tags, user interaction behavior, and so on. Due to the large scale, clear structure, and wide coverage of the MovieLens dataset, researchers can use it to test content-based recommendation algorithms, cold start problems, hybrid recommendation methods [3]. Moreover, the Netflix Prize dataset and Amazon Review dataset are often used as tools to test the functionality of recommendation systems. They are datasets created by large companies Netflix and Amazon respectively, covering user feedback data on their dramas and products, and are applied to algorithm training. Some researchers also use these two datasets to validate their research findings [3].
2.2. Proposed Approach
The objective of this review article is to organize and summarize current research findings on RS. The study's pipeline is illustrated in Figure 1. The introduction section elaborates on the research background of RSs based on ML and DL, and provides an overview of previous achievements and related technological developments in this field. The methodology section details the working principles of ML and DL, as well as common RS algorithms and models, including the datasets used by researchers to validate their findings. This section also explains the core technologies of ML and DL and their applications in specific RS models. The Results and Discussion section analyzes and discusses the test results of several mature RS models, providing a basic evaluation of their performance. It also examines the advantages and disadvantages of these RS models, as well as their potential application areas, based on their principles and performance. Finally, the Conclusion section summarizes the research content of the entire article and offers prospects for future research directions.
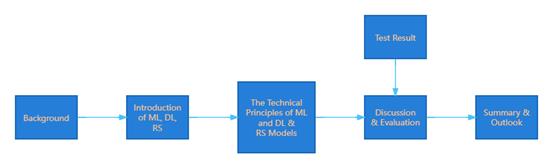
Figure 1. The pipeline of the study
2.2.1. Overview of Basic Technologies
ML is a core technology in artificial intelligence, using algorithms and statistical models to identify patterns in data for predictions and decision-making without explicit programming. Essential in recommendation systems, ML tackles five main problems: classification (e.g., image recognition), regression (e.g., housing price forecasting), ranking (e.g., search engine results), clustering (e.g., social media connections), and dimensionality reduction (simplifying high-dimensional data) [4]. ML employs four types of learning: supervised (training for tasks like classification and regression based on labeled data), unsupervised (analyzing unlabeled data for clustering and dimensionality reduction), semi-supervised (combining a little labeled data with a quantity of unlabeled data), and reinforcement (learning through interaction and reward mechanisms, often used in gaming and decision-making systems). These approaches enable ML to handle various complex tasks, enhancing its applicability in diverse fields [5].
Furthermore, DL is a subset of ML [5]. DL covers three main types: supervised learning, unsupervised learning, and reinforcement learning. Each type is suitable for different data and task scenarios. By using multi-layer neural networks to automatically extract features and patterns from data, current researchers mainly focus on four different types of algorithms for DL development. Convolutional neural networks (CNNs) are mainly used for processing image and video data. It has convolutional and pooling layers for feature extraction and pattern recognition [6,7]. Recurrent neural networks (RNNs) are mainly used to process sequential data. Like time series and natural language, capturing temporal dependencies through loop structures [6,7]. Generative Adversarial Networks (GANs) mainly consist of two adversarial networks: generators and discriminators. It is used to generate realistic data samples [4]. Autoencoders (AE) are mainly used for unsupervised learning. It performs feature extraction and dimensionality reduction through encoding and decoding processes [4,7]. In the architecture of real recommendation systems, DL has made many new contributions in natural language processing (NLP) and image classification.
2.2.2. The Mainstream Technology Model of Machine Learning Based Recommendation System
ML-based recommendation systems aim to automatically provide personalized products, services, or suggestions to users based on their preferences and behavior patterns. By analyzing users' historical and behavioral data, this RS can predict content that users may be interested in, thereby improving user experience and satisfaction. To construct a complete RS, researchers typically use three main recommendation methods: CR method, CF method, HF method. At the same time, the cold start problem is an important issue in RS design that requires the use of these recommendation algorithms to solve. The relationship between these methods and algorithms is shown in Figure 2.
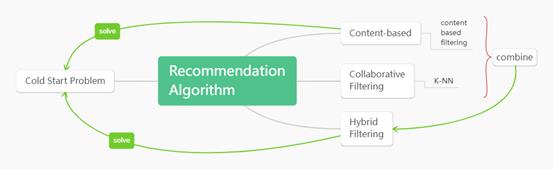
Figure 2. The relationship map in the recommendation algorithm
The cold start problem refers to the difficulty in providing accurate personalized recommendations in RSs when new users or items lack sufficient historical data. In this case, traditional recommendation methods may fail because they rely on a large amount of user behavior data for recommendations. This problem is mainly reflected in the emergence of new users, new data, and sparse data. Content-based recommendation recommends new items to users that are similar to their historical favorites by analyzing the specific attributes of each item and user behavior records [8]. Its working principle is to first complete feature extraction, then model user preferences, and finally generate targeted recommendations. For first-time registered users, there is no previous relevant data with other users or behavioral data. Therefore, RS usually requires users to submit initial preferences to match appropriate items. This method mainly relies on the content information of items, rather than the behavioral similarity between users. This allows it to alleviate the cold start problem to some extent.
CF predicts user preferences by analyzing large datasets, dividing into user-based and item-based approaches. User-based CF calculates similarities between users to recommend items that similar users enjoy. Item-based CF identifies similar items to those a user likes and recommends them accordingly [9]. The strength of CF lies in its reliance on relevance rather than content analyzability, making it effective for recommending complex items like movies. Common CF techniques include the K-NN algorithm, which uses metrics like Euclidean distance, cosine similarity, and Jaccard similarity to find the most similar users or items for recommendations. HF combines content-based and CF methods to improve recommendation accuracy. One HF approach performs independent content-based and CF predictions and merges them, leveraging both methods' strengths [10]. Another approach integrates content-based features into CF or vice versa, known as content-based filtering (CBF). This method refines recommendations based on content preferences in addition to CF. HF can also incorporate weight calculations, blending two recommendation lists based on predefined weights to generate the final list, effectively addressing cold start problems.
2.2.3. The Mainstream Technology Model of Recommendation System Based on DL
On the basis of ML, many RSs further use DL architecture to implement CF, CBF, and HF. There are research results indicating that the most widely used DL architecture in RS is AE [3]. Further, CNNs and RNNs models are also frequently used [3]. These three DL models can automatically extract features from data, abstracting them layer by layer, from low-level features to high-level features. Compared to traditional ML methods, DL methods reduce the dependence of RS on artificial feature engineering and improve generalization ability.
The basic structure of AE includes an input layer, one or more hidden layers, and an output layer. In order to facilitate coding, information needs to be processed before entering the input layer; After a series of encoding and decoding processes, the output content should be the same as the original input. AEs learn the distribution of data by mapping input data to a low dimensional space (encoder) and then reconstructing the input data from the low dimensional space (decoder) [3,7]. The composition principle of AE is shown in Figure 3. AE has three variants. Sparse autoencoder (SAE) can be used to mitigate the impact of cold start issues. This is because sparsity constraints are added to AE's loss function, allowing it to learn sparse representations of data [3]. Denoising autoencoder (DAE) adds noise to the input data and then trains the autoencoder to reconstruct the original data [3]. Stacked Autoencoder (SAE): stacks multiple autoencoders together, trains them layer by layer, and finally forms a deep network structure [3].
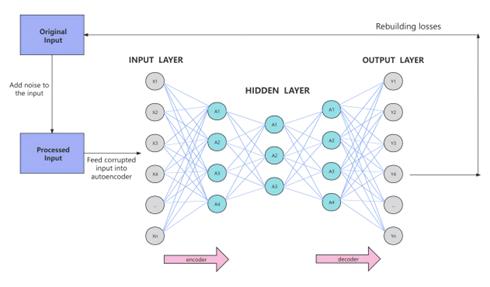
Figure 3. The composition principle of AE
A typical combined model is that some researchers use stacked denoising autoencoders (SDAE) to extract content features of target items [11]. SDAE is composed of multiple DAEs stacked together, with each layer of DAE trained by minimizing the reconstruction error of the input. The training process is divided into two parts: encoding and decoding: the encoding part learns the representation of input features, and the decoding part reconstructs the input. By training layer by layer, researchers ultimately obtain low dimensional content feature vectors of the project in the middle layer of the network. The research results indicate that SDAE can assist in solving the problems of complete cold start (CCS) and incomplete cold start (ICS) [11]. In recent years, some professors have proposed an attention model called Longest Short-Term Memory CNN based attention model (LCNA), aimed at solving the cold start problem in CF processing [6]. In order to improve the accuracy of the model's recommendations, deep attention layers and cosine similarity methods are introduced for semantic ranking. The workflow of LCNA is shown in Figure 4.
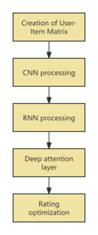
Figure 4. The workflow of LCNA
In the LCNA model, the input data is divided into item and user latent factor matrices, which are then combined into an interaction matrix. This matrix undergoes nonlinear mapping via a CNN to generate a user rating matrix. Training data is then fed in parallel into an LSTM-RNN, providing short-term memory and semantic sorting. The sorted data from the CNN is input into a deep attention layer, where cosine similarity is used to further optimize the scores. By comparing deep attention layers and semantic ratings, the performance of the recommendation system is enhanced. This integration of CNN and RNN allows the system to utilize both local and sequential features for recommendations, enhancing its performance, particularly with complex and dynamic data. By extracting nonlinear features through CNN and capturing time series features through RNN, the LCNA model delivers more accurate and personalized recommendations, improving the marketing strategies of e-commerce platforms.
3. Result and Discussion
This chapter examines and compares the results of various popular RS schemes, focusing on key performance attributes: prediction accuracy, coverage, ranking quality, and the ability to address the cold start problem. Prediction accuracy measures how well the RS forecasts user ratings or behaviors, while coverage assesses the system's ability to encompass a wide range of items and users, reflecting its comprehensiveness and diversity. Ranking quality evaluates how well the recommended list matches user preferences, impacting user satisfaction and experience [12]. To ensure reliable results, statistical significance tests and confidence intervals are calculated. Performance indicators include root mean square error (RMSE), mean absolute error (MAE), recall rate, Gini index, and Shannon entropy. This chapter contrasts the performance of advanced DL algorithms with traditional ML algorithms on the same dataset, and examines how different DL models perform when trained on various datasets
3.1. Performance Comparison between DL Algorithm and Traditional ML Algorithm
Mu, Y. et al. constructed a personalized movie recommendation system by introducing multimodal data analysis into DL methods and trained it using a single MovieLens dataset [8]. Subsequently, the researchers collected the RMSE of this DL algorithm obtained on the MovieLens 100K and 1M datasets, and compared its performance with traditional CF algorithms [8]. The specific data is shown in Table 1.
The RMSE of Item-based CF (Item-CF) on the 100K dataset is 1.073, and on the 1M dataset it is 0.970. This indicates that this algorithm has a larger error when processing less data, and the error decreases when the amount of data increases. The RMSE of user-based CF (User-CF) is 1.034 on the 100K dataset and 0.940 on the 1M dataset. Compared to Item-CF, User-CF performs slightly better on both datasets. The RMSE of singular value decomposition (SVD) is 0.963 on the 100K dataset and 0.930 on the 1M dataset. The performance of SVD algorithm is superior to traditional CF algorithms, especially when the data volume is large, the error is significantly reduced. The RMSE of poster free deep learning is 0.9908 on the 100K dataset and 0.9096 on the 1M dataset. Although the error is slightly higher than SVD in the case of small data volume (100K), it performs well and significantly reduces the error in the case of large data volume (1M). The RMSE of multimodal with posters is 0.986 on the 100K dataset and 0.905 on the 1M dataset. This shows that after adding multimodal data, the error of the recommendation system is further reduced, and the performance is better than that of the single mode deep learning algorithm.
Table 1. RMSE obtained on MovieLens 100K and 1M datasets
Algorithms |
RMSE (100K) |
RMSE (1M) |
Item-CF |
1.073 |
0.97 |
User-CF |
1.034 |
0.94 |
SVD |
0.963 |
0.93 |
DL (without posters) |
0.9908 |
0.9096 |
Multimodal (with posters) |
0.986 |
0.905 |
3.2. Performance Comparison of Multiple DL Models in RS
Zhang, S. et al tested several core models in DL using different datasets and obtained detailed results [2]. The specific experimental results are shown in Table 2. The multi-layer perceptron (MLP) trained on the MovieLens dataset achieved an accuracy of 0.785 and a recall of 0.748. AE was trained on the Netflix dataset, and the calculated MAE was 0.823 and RMSE was 1.032. CNN trained on the Amazon dataset achieved an accuracy of 0.812 and a recall of 0.769. The RNN trained on the MovieLens dataset achieved an accuracy of 0.798 and a recall of 0.755. The MAE and RMSE obtained from training the Restricted Boltzmann Machine (RBM) on the Netflix dataset were 0.845 and 1.059, respectively. Deep reinforcement learning (DRL) was trained on the Amazon dataset and achieved accuracy and recall rates of 0.825 and 0.778, respectively.
From these data, it can be seen that the performance of different DL models varies on different datasets. In terms of accuracy and recall: CNN and DRL models perform well on the Amazon dataset, with higher accuracy and recall than other models; The performance of the RNN model on the MovieLens dataset should not be underestimated, as the results show that it has high accuracy and recall. In terms of MAE and RMSE: AE and RBM models perform well on the Netflix dataset, but RMSE is slightly higher, indicating significant errors in some predictions. The MLP model performs well on the MovieLens dataset, but its recall rate is slightly lower than that of CNN and RNN models.
Table 2. The experimental results of DL models
Models |
Dataset |
Accuracy |
Recall |
MAE |
RMSE |
MLP |
MovieLens |
0.785 |
0.748 |
- |
- |
AE |
Netflix |
- |
- |
0.823 |
1.032 |
CNN |
Amazon |
0.812 |
0.769 |
- |
- |
RNN |
MovieLens |
0.798 |
0.755 |
- |
- |
RBM |
Netflix |
- |
- |
0.845 |
1.059 |
DRL |
Amazon |
0.825 |
0.778 |
- |
- |
3.3. Discussion
ML and DL each offer distinct advantages and are suited for different types of problems and data scenarios. ML excels with small-scale, low-dimensional data and applications needing interpretability, while DL shines with large-scale, high-dimensional data and complex tasks, though it demands more computing resources and data. Research by Mu et al. shows that traditional collaborative filtering methods, such as Item-CF and user CF, struggle with data sparsity and cold start issues, which matrix decomposition techniques like SVD can partially mitigate [8]. DL algorithms handle high-dimensional and nonlinear data effectively, particularly with large datasets exceeding 1 million entries, and integrating multimodal data further enhances recommendation accuracy and user experience.
Research by Zhang et al. highlights that each DL model has unique strengths and weaknesses. MLP are straightforward and can improve performance by increasing hidden layers and nodes but may falter with high-dimensional sparse data. AE handle sparse data well and address cold start problems but may have high reconstruction errors, impacting recommendation accuracy and extending training times [2]. CNN excel at capturing local features, making them suitable for content recommendations, while RNN are effective for predicting user behavior and time-sensitive recommendations. Choosing the right DL models and datasets is essential for optimizing recommendation system performance.
Looking at the current RS designs, in addition to the indispensable cold start problem, there are also some other issues that need improvement. Firstly, DL typically has high complexity and computational cost, requiring a large amount of training data and time. Secondly, RS needs to enhance transparency and interpretability to increase user trust. Finally, the RS algorithm contains a large number of hyperparameters, requiring extensive experimentation and tuning work. To address these current issues, researchers can consider enhancing the data preprocessing capability and feature engineering of RS, compressing and accelerating DL models, enhancing the interpretability of DL models through techniques such as attention mechanism interpretability networks, and attempting to introduce automated hyperparameter tuning. Future research should focus more on efforts to improve data processing, optimize model structure, increase computational efficiency, and enhance model interpretability.
4. Conclusion
This paper offers a comprehensive review of ML and DL applications in RS design, highlighting core technologies. The challenge of information overload on the Internet impacts user efficiency, but effective RS can significantly enhance user experience by delivering precise content recommendations. Consequently, RS design has become a prominent research area with substantial growth potential. Traditional ML methods are reliable for small-scale, low-dimensional data, but DL techniques have demonstrated considerable advantages with increasing data volume and complexity. Despite the availability of advanced DL methods, challenges such as cold start issues, model transparency, interpretability, and extensive hyperparameter tuning persist. Future research should focus on improving data preprocessing and feature engineering, compressing and accelerating DL models, enhancing model interpretability through attention mechanisms and interpretive networks, and exploring automatic hyperparameter tuning. This study aims to advance ML and DL technologies to develop smarter and more efficient RS, ultimately delivering better services to users.
References
[1]. Chate, P. J. (2019). The Use of Machine Learning Algorithms in Recommender Systems: A Systematic Review. IJRAR-International Journal of Research and Analytical Reviews (IJRAR), 6(2), 671-681.
[2]. Zhang, S., Yao, L., Sun, A., & Tay, Y. (2019). Deep learning based recommender system: A survey and new perspectives. ACM computing surveys (CSUR), 52(1), 1-38.
[3]. Da’u, A., & Salim, N. (2020). Recommendation system based on deep learning methods: a systematic review and new directions. Artificial Intelligence Review, 53(4), 2709-2748.
[4]. Mu, R. (2018). A survey of recommender systems based on deep learning. Ieee Access, 6, 69009-69022.
[5]. Janiesch, C., Zschech, P., & Heinrich, K. (2021). Machine learning and deep learning. Electronic Markets, 31(3), 685-695.
[6]. Sethi, V., Kumar, R., Mehla, S., Gandhi, A. B., Nagpal, S., & Rana, S. (2024). Original Research Article LCNA-LSTM CNN based attention model for recommendation system to improve marketing strategies on e-commerce. Journal of Autonomous Intelligence, 7(1).
[7]. Singhal, A., Sinha, P., & Pant, R. (2017). Use of deep learning in modern recommendation system: A summary of recent works. arXiv preprint arXiv:1712.07525.
[8]. Mu, Y., & Wu, Y. (2023). Multimodal movie recommendation system using deep learning. Mathematics, 11(4), 895.
[9]. Nawrocka, A., Kot, A., & Nawrocki, M. (2018, May). Application of machine learning in recommendation systems. In 2018 19th International carpathian control conference (ICCC), 328-331.
[10]. Thorat, P. B., Goudar, R. M., & Barve, S. (2015). Survey on collaborative filtering, content-based filtering and hybrid recommendation system. International Journal of Computer Applications, 110(4), 31-36.
[11]. Wei, J., He, J., Chen, K., Zhou, Y., & Tang, Z. (2017). Collaborative filtering and deep learning based recommendation system for cold start items. Expert Systems with Applications, 69, 29-39.
[12]. Shani, G., & Gunawardana, A. (2011). Evaluating recommendation systems. Recommender systems handbook, 257-297.
Cite this article
Song,J. (2024). Analysis on recommendation systems based on ML and DL approaches. Applied and Computational Engineering,88,150-157.
Data availability
The datasets used and/or analyzed during the current study will be available from the authors upon reasonable request.
Disclaimer/Publisher's Note
The statements, opinions and data contained in all publications are solely those of the individual author(s) and contributor(s) and not of EWA Publishing and/or the editor(s). EWA Publishing and/or the editor(s) disclaim responsibility for any injury to people or property resulting from any ideas, methods, instructions or products referred to in the content.
About volume
Volume title: Proceedings of the 6th International Conference on Computing and Data Science
© 2024 by the author(s). Licensee EWA Publishing, Oxford, UK. This article is an open access article distributed under the terms and
conditions of the Creative Commons Attribution (CC BY) license. Authors who
publish this series agree to the following terms:
1. Authors retain copyright and grant the series right of first publication with the work simultaneously licensed under a Creative Commons
Attribution License that allows others to share the work with an acknowledgment of the work's authorship and initial publication in this
series.
2. Authors are able to enter into separate, additional contractual arrangements for the non-exclusive distribution of the series's published
version of the work (e.g., post it to an institutional repository or publish it in a book), with an acknowledgment of its initial
publication in this series.
3. Authors are permitted and encouraged to post their work online (e.g., in institutional repositories or on their website) prior to and
during the submission process, as it can lead to productive exchanges, as well as earlier and greater citation of published work (See
Open access policy for details).
References
[1]. Chate, P. J. (2019). The Use of Machine Learning Algorithms in Recommender Systems: A Systematic Review. IJRAR-International Journal of Research and Analytical Reviews (IJRAR), 6(2), 671-681.
[2]. Zhang, S., Yao, L., Sun, A., & Tay, Y. (2019). Deep learning based recommender system: A survey and new perspectives. ACM computing surveys (CSUR), 52(1), 1-38.
[3]. Da’u, A., & Salim, N. (2020). Recommendation system based on deep learning methods: a systematic review and new directions. Artificial Intelligence Review, 53(4), 2709-2748.
[4]. Mu, R. (2018). A survey of recommender systems based on deep learning. Ieee Access, 6, 69009-69022.
[5]. Janiesch, C., Zschech, P., & Heinrich, K. (2021). Machine learning and deep learning. Electronic Markets, 31(3), 685-695.
[6]. Sethi, V., Kumar, R., Mehla, S., Gandhi, A. B., Nagpal, S., & Rana, S. (2024). Original Research Article LCNA-LSTM CNN based attention model for recommendation system to improve marketing strategies on e-commerce. Journal of Autonomous Intelligence, 7(1).
[7]. Singhal, A., Sinha, P., & Pant, R. (2017). Use of deep learning in modern recommendation system: A summary of recent works. arXiv preprint arXiv:1712.07525.
[8]. Mu, Y., & Wu, Y. (2023). Multimodal movie recommendation system using deep learning. Mathematics, 11(4), 895.
[9]. Nawrocka, A., Kot, A., & Nawrocki, M. (2018, May). Application of machine learning in recommendation systems. In 2018 19th International carpathian control conference (ICCC), 328-331.
[10]. Thorat, P. B., Goudar, R. M., & Barve, S. (2015). Survey on collaborative filtering, content-based filtering and hybrid recommendation system. International Journal of Computer Applications, 110(4), 31-36.
[11]. Wei, J., He, J., Chen, K., Zhou, Y., & Tang, Z. (2017). Collaborative filtering and deep learning based recommendation system for cold start items. Expert Systems with Applications, 69, 29-39.
[12]. Shani, G., & Gunawardana, A. (2011). Evaluating recommendation systems. Recommender systems handbook, 257-297.