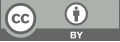
An analysis of machine learning's role in stock price prediction
- 1 Beijing LiZe International Academy
* Author to whom correspondence should be addressed.
Abstract
The volatility and uncertainty of the stock market can reflect the economic trends of today's society. With the improvement of computing power and the development of data processing, it has been found that machine learning can improve the accuracy, flexibility and interpretability of stock price predictions. To conduct an in-depth study, this paper introduces three methods in machine learning: neural networks, support vector machines and random forest. These three algorithms can predict the stock market from multiple perspectives, complementing each other’s weaknesses. The results show that, while neural networks tend to overfit, random forests are resistant to overfitting. By combining neural networks with random forests, researchers can enhance prediction accuracy. Additionally, the random forest algorithm has strong data processing capabilities, and it can effectively address the issue of low computational efficiency in the support vector machine algorithm.
Keywords
Machine Learning, Neural Networks, Support Vector Machines, Random Forest, Sock Price Prediction
[1]. Wang Jiazeng. 2023. Research on stock price prediction and stock portfolio based on improved LSTM neural network[D]. Xi'an University of Architecture and Technology, 2023. DOI: 10.27393/d.cnki.gxazu.2023.000894
[2]. Gao Ziyi. 2024. Random Forest-based Stock Price Trend Prediction Research.
[3]. F.-F. Li, A. Karpathy, and J. Johnson, “Stanford CS class CS231n: Convolutional Neural Networks for Visual Recogni tion,” http://cs231n.stanford.edu/.
[4]. Steinwart, I., & Christmann, A. (2008). Support vector machines. Springer Science & Business Media.
[5]. Getting Started with the Python Random Forest Algorithm and Performance Evaluation https://zhuanlan.zhihu.com/p/70233215
[6]. Peng Yan, Liu Yuhong, Zhang Rongfen. 2019. Modeling and analysis of stock price prediction based on LSTM. Computer Engineering and Applications, 55(11):209-212
[7]. Z.H. Chen. 2024. Research on stock price prediction based on machine learning algorithm[D]. Dongguan Institute of Technology. DOI: 10.44357/d.cnki.gdgut.2024.000171.
[8]. Deng Yun. Time selection strategy for the Shanghai and Shenzhen 300 Index based on wavelet denoising and random forest algorithm (Doctoral dissertation, Huazhong University of Science and Technology)
[9]. Jabed, Mahfuz Islam Khan. (2024). Stock Market Price Prediction using Machine Learning Techniques. American International Journal of Sciences and Engineering Research. 7. 1-6. 10.46545/aijser. v7i1.308.
[10]. Zhang Lei. 2022. Stock price prediction based on investor sentiment [D]. Qufu Normal University. doi: 10.27267/d.cnki.gqfsu.2022.000576.
[11]. Hou, Yani. (2021). Stock Price Prediction Based on Bi-LSTM Deep Learning. Statistics and Application.10.538-546.10.12677/SA.2021.103055.
Cite this article
Meng,X. (2024). An analysis of machine learning's role in stock price prediction. Applied and Computational Engineering,92,115-120.
Data availability
The datasets used and/or analyzed during the current study will be available from the authors upon reasonable request.
Disclaimer/Publisher's Note
The statements, opinions and data contained in all publications are solely those of the individual author(s) and contributor(s) and not of EWA Publishing and/or the editor(s). EWA Publishing and/or the editor(s) disclaim responsibility for any injury to people or property resulting from any ideas, methods, instructions or products referred to in the content.
About volume
Volume title: Proceedings of the 6th International Conference on Computing and Data Science
© 2024 by the author(s). Licensee EWA Publishing, Oxford, UK. This article is an open access article distributed under the terms and
conditions of the Creative Commons Attribution (CC BY) license. Authors who
publish this series agree to the following terms:
1. Authors retain copyright and grant the series right of first publication with the work simultaneously licensed under a Creative Commons
Attribution License that allows others to share the work with an acknowledgment of the work's authorship and initial publication in this
series.
2. Authors are able to enter into separate, additional contractual arrangements for the non-exclusive distribution of the series's published
version of the work (e.g., post it to an institutional repository or publish it in a book), with an acknowledgment of its initial
publication in this series.
3. Authors are permitted and encouraged to post their work online (e.g., in institutional repositories or on their website) prior to and
during the submission process, as it can lead to productive exchanges, as well as earlier and greater citation of published work (See
Open access policy for details).