Abstract
Born from the machine learning (ML) subfield of neural networks (NN), deep learning (DL) has many advantages over other ML algorithms and has become more significant today. As one of the most essential model architectures of DL, the convolutional neural network (CNN) has attracted the attention of many researchers, especially in recent years. Meanwhile, sentiment analysis has become more renowned since the rapid development of various online platforms like blogs, social networks, etc. To study these two heated topics together, this article selects a particular CNN model designed for sentiment analysis and explores its width’s potential influence on the result. During the experiment, four CNN models are created based on the same structure but with increasing width. By forwarding the pre-processed datasets to the four models and comparing their performances from different perspectives using different metrics, it’s concluded that the more expansive the model's width, the better it performs in the training, validation, and testing sections.
Keywords
Sentiment analysis, Deep learning, Convolutional neural network
1. Introduction
A tiny subset of artificial intelligence (AI), commonly called machine learning (ML), has transformed numerous fields during the past few decades since the 1950s [1]. Deep Learning (DL) was born from the ML subfield of neural networks (NN). DL refers to a particular area of ML that emphasizes learning successive layers of progressively more essential representations from data, offering a novel approach to learning and extracting features from it [2]. There are many different architecture models in DL, and the Convolutional Neural Network (CNN) is one of them. The CNN is a feedforward neural network that can identify data characteristics with convolutional patterns and has been making promising achievements [3]. For example, CNN-based computer vision has allowed people to do things like facial recognition, self-driving cars, self-service supermarkets, and intelligent medical treatments—things thought unattainable just a few generations ago [3]. Recently, researchers worldwide have conducted numerous experiments from different perspectives in the CNN domain. For example, regarding applying the CNN model to an entirely new domain, aiming to identify individual appliances' power consumption, a CNN-based nonintrusive load monitoring algorithm has been proposed to extract the energy demand of each individual device [4]. Moreover, aiming to achieve intelligent waste identification and recycling, a novel CNN model has been proposed to recognize and localize multilabel waste simultaneously and demonstrated excellent performance [5]. During the process of broadening the applications of different kinds of CNN models, instead of utilizing existing CNN models, researchers may decide to create new CNN models as well. For example, a new CNN model called BrainMRNet has been proposed by [6] to detect brain tumors.
However, many studies on CNN nowadays, like the ones shown above, are either focused on applying CNN to a new application field or proposing a new architecture of the CNN model and discussing its performance during practical application. Meanwhile, more research is needed on the fundamentals of CNN, such as how the performance of the CNN model can be improved by adjusting its structure, like width or depth.
CNN has been applied to many application situations, and one valuable and meaningful application scenario needs to be chosen to conduct the target research purpose. In this article, the sentiment analysis is selected. The process of obtaining and analyzing people's views, ideas, and perceptions concerning various subjects, products, and services is known as sentiment analysis [7]. Nowadays, people produce numerous comments and reviews regarding goods, services, and daily activities because of the rapid expansion of Internet-based applications such as blogs, social networks, and websites. Sentiment analysis can be utilized as a potent tool to gather and examine these reviews and moods to improve parties like researchers’ decision-making stage and gain business insights [8]. It’s instrumental across multiple application domains like business intelligence, recommendation systems, government intelligence, and healthcare and medicine [8]. Therefore, it’s meaningful to conduct experiments under this background.
Therefore, aiming to navigate the fundamentals of the CNN model in terms of sentiment analysis, this article selects a particular CNN model designed for sentiment analysis and conducts experiments to examine its width’s potential influences on training, validation, and testing results.
2. Method
2.1. Dataset and preprocessing
The dataset containing 50K Internet Movie Database (IMDB) movie reviews downloaded from Kaggle [9] is implemented in this experiment. Aiming to mark the sentiment behind the text, each review among the dataset is tagged as either positive or negative, and the number of these two categories is evenly distributed, with 25K each.
The following preprocessing steps are conducted to convert the raw text to the format that can be forwarded to the model. First, all letters in the text will be converted to lowercase. Second, all punctuation marks like commas will be removed. Afterward, the text will be split into individual words called tokens. Finally, stop words among the tokens will be deleted.
So far, the preprocessing of the raw text has been completed, and a matrix of tokens’ lists has been generated. However, the matrix is still not the final input format. All the tokens within the matrix will be converted to sequences using the tokenizer provided by the TensorFlow library. Furthermore, all the sequences will be padded into the same length since the input dimension of the model should be fixed. For the corresponding tags, binary encoding is utilized, transferring the “positive” tag to integer one and “negative” to zero.
2.2. Model architecture
Four models of increasing width are implemented. Their primary structures are all based on the CNN-non-static architecture proposed by [10]. Figure 1 illustrates its components.
The model utilizes pre-trained word vectors generated by word2vec. These vectors are trainable and will be fine-tuned for each task. The first critical component of the model is the embedding layer, which will create an n*k matrix representation for each sentence based on the pre-trained embedding matrix and indexes of each token within it. For example, in Figure 1, the sentence ‘This is your pen’s n*k matrix representation is generated. After getting the matrix representation of each sentence, it will be passed through the convolutional layer with different kernel sizes for feature extraction. Afterward, these feature maps will be passed through the max pooling and dense layer sequentially to get the predicted possibility of whether the inputted sentence is a positive or negative review.
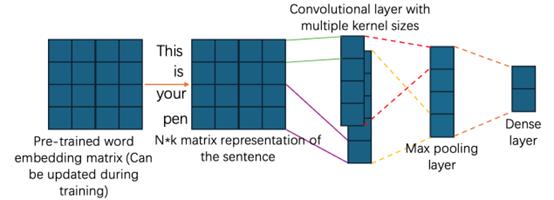
Figure 1. Basic architecture of the four models with different widths (Figure Credits: Original).
2.3. Evaluation metrics
The training and validation processes measure different models' performances using the loss, accuracy, precision, and recall scores. The final accuracy score evaluates different models’ performances on the testing dataset.
3. Experiment and Result
3.1. Training details
Four models sharing the same structure but with increasing widths are designed for the experiment. Model A’s word vector’s length for each token is 100, and each sentence's max length is set to 100 when performing padding. On the contrary, model B’s value for these two hyperparameters is 200. Model C's is 300, and model D’s is 325. The number of training epochs for the four models is 20. The batch size of one iteration is 3072. The number of filters within the convolutional layer is 250, while the kernels’ lengths are 2, 3, 4, and 5. The model has two dense layers, with 250 neurons in the first and 1 unit in the last. The regularization strength of the L2 regularization is 0.05. The verbose value for the training and validation process is 1, displaying a progress bar updating each epoch and showing each metric's progress throughout a specific epoch. The verbose number for the testing stage is 0, evaluating the model’s testing performance silently without displaying any output during the process.
Two sub-datasets are split from the initial dataset: training and testing. The training dataset is implemented for the training process. For the validation and testing section, the testing dataset is utilized. The training and testing dataset proportion is 80% and 20%. The execution platform of the experiment is Google Colab.
3.2. Result comparison
Tables below illustrates the comparison results from different metric perspectives of the four models with increasing width. Aiming to simplify the comparison process and make it more intuitive, the values shown in the table are the returning results after the whole training process, 20 epochs in this experiment. Table 1 compares the four model’s performances during the training process. Table 2 demonstrates their performances throughout the validation phase. Furthermore, the final testing accuracy scores are shown in Table 3.
Table 1. Performance comparison of four models during training.
Metrics |
Model A |
Model B |
Model C |
Model D |
Loss |
0.6654 |
0.4706 |
0.3512 |
0.2275 |
Accuracy |
0.9258 |
0.9653 |
0.9697 |
0.9796 |
Precision |
0.9248 |
0.9654 |
0.9688 |
0.9783 |
Recall |
0.9267 |
0.9649 |
0.9705 |
0.9810 |
Table 2. Performance comparison of four models during validation.
Metrics |
Model A |
Model B |
Model C |
Model D |
Loss |
0.7628 |
0.5819 |
0.4666 |
0.3784 |
Accuracy |
0.8511 |
0.8929 |
0.8960 |
0.8970 |
Precision |
0.9315 |
0.9123 |
0.8940 |
0.8764 |
Recall |
0.7605 |
0.8712 |
0.9004 |
0.9262 |
Table 3. Performance comparison of four models during testing.
Metrics |
Model A |
Model B |
Model C |
Model D |
Accuracy |
0.8511 |
0.8929 |
0.8960 |
0.8970 |
4. Discussion
Table 1 illustrates the performance of different models after the entire training stage. The model with a broader width has fewer losses and scores higher accuracy, precision, and recall scores than the other models. In this experiment, model D, designed to have the most expansive width among the four models, performs best since it has the fewest losses and the highest accuracy, precision, and recall scores. By contrast, model A (with the narrowest width) is the worst performer since its loss is the highest while the accuracy, precision, and recall scores are the lowest.
Afterward, from the validation process shown in Table 2, like the training phase, the model with the broadest width (model D) has the lowest loss among the four models. Moreover, model D also scores the highest in accuracy and recall scores. However, unlike the training process, it has the lowest score in precision, and model A’s precision score is the highest. The conflict between the precision and recall scores is reasonable since they evaluate the models through two distinctive dimensions. Recall is the proportion of Real Positive cases that are correctly Predicted Positive. Conversely, Precision denotes the percentage of Predicted Positive cases that are correctly Real Positives [11].
In this case, when the recall score is high, the number of reviews that are negative themselves but predicted as positive ones is increased. Furthermore, its increasing speed is faster than that of True Positives (the number of reviews that are positive themselves and predicted as positive ones correctly). As a result, the precision score will be decreased.
For Table 3, since model D scores the highest accuracy, its performance is the best among the four models.
Therefore, based on the results of the above tables, it’s concluded that in this situation, the more expansive the model’s width, the better it demonstrates for the training, validation, and testing stage.
However, some potential limitations and issues in the experiment still need to be addressed and improved during future work. First, the dataset implemented in the experiment is relatively small, and its diversity needs to be more significant. The number of reviews within the dataset is 50K, categorized as positive or negative, which is not enough compared to today’s online reviews. The dataset's limited size may result in overfitting, which is wanted to be avoided for DL tasks [12]. Therefore, aiming to make the experiment more convincing and scientific, the number of reviews within the dataset is expected to be enlarged, and more category tags are required, such as happy, upset, angry, etc. The second is about the experiment itself. The optimal width for the model has yet to be found, and future work needs to be done to identify when the width is increased and up to which point the model's performance is no longer improved. Besides, rather than simply changing the model's width or depth, other parts, like the pre-trained word embeddings and the number of kernel sizes, can also be adjusted in future work to examine the model’s performance, making the experiment more detailed and comprehensive.
5. Conclusion
To conclude, the article utilized an existing CNN model designed for sentiment analysis and explored its different width value’s potential influences on the training, validation, and testing result. Four models with increasing width are generated during the experiment to examine the result. Through forwarding the pre-processed dataset to the four models with narrow to wide widths and recording as well as comparing their final performances using different metrics, since wider models have higher metric scores in accuracy and lower scores in loss, it is concluded that in this scenario, the wider the model's width, the better it performs in the training, validation, and testing sections. Although much work has been done during the experiment process, there is still much work to be done based on it, and some parts can also be improved. Currently, scholars around DL are conducting a lot of research. Hopefully, more research can be employed to investigate the fundamentals of the DL models, such as their width, depth, and other hyperparameters. Since DL has been applied to various application scenarios and is closely related to people’s daily lives, it’s necessary and meaningful to try to improve the DL model’s performance by adjusting its primary structures.
References
[1]. Alom, M. Z., Taha, T. M., Yakopcic, C., Westberg, S., Sidike, P., Nasrin, M. S., ... & Asari, V. K. (2019). A state-of-the-art survey on deep learning theory and architectures. electronics, 8(3), 292.
[2]. Chollet, F. (2021). Deep learning with Python. Simon and Schuster.
[3]. Li, Z., Liu, F., Yang, W., Peng, S., & Zhou, J. (2021). A survey of convolutional neural networks: analysis, applications, and prospects. IEEE transactions on neural networks and learning systems, 33(12), 6999-7019.
[4]. Ciancetta, F., Bucci, G., Fiorucci, E., Mari, S., & Fioravanti, A. (2020). A new convolutional neural network-based system for NILM applications. IEEE Transactions on Instrumentation and Measurement, 70, 1-12.
[5]. Zhang, Q., Yang, Q., Zhang, X., Wei, W., Bao, Q., Su, J., & Liu, X. (2022). A multi-label waste detection model based on transfer learning. Resources, Conservation and Recycling, 181, 106235.
[6]. Toğaçar, M., Ergen, B., & Cömert, Z. (2020). BrainMRNet: Brain tumor detection using magnetic resonance images with a novel convolutional neural network model. Medical hypotheses, 134, 109531.
[7]. Wankhade, M., Rao, A. C. S., & Kulkarni, C. (2022). A survey on sentiment analysis methods, applications, and challenges. Artificial Intelligence Review, 55(7), 5731-5780.
[8]. Birjali, M., Kasri, M., & Beni-Hssane, A. (2021). A comprehensive survey on sentiment analysis: Approaches, challenges and trends. Knowledge-Based Systems, 226, 107134.
[9]. IMDB Dataset of 50K Movie Reviews. (2019) URL: https://www.kaggle.com/datasets/lakshmi25npathi/imdb-dataset-of-50k-movie-reviews. Last Accessed 2024/08/23
[10]. Kim, Y. (2014). Convolutional neural networks for sentence classification. rXiv preprint arXiv: 1408.5882
[11]. Powers, D. M. (2020). Evaluation: from precision, recall and F-measure to ROC, informedness, markedness and correlation. arXiv preprint arXiv:2010.16061.
[12]. Ying, X. (2019). An overview of overfitting and its solutions. In Journal of physics: Conference series, 1168, 022022.
Cite this article
Xiao,C. (2024). Exploring the impact of width in convolutional neural network-based architectures for sentiment analysis. Applied and Computational Engineering,94,1-5.
Data availability
The datasets used and/or analyzed during the current study will be available from the authors upon reasonable request.
Disclaimer/Publisher's Note
The statements, opinions and data contained in all publications are solely those of the individual author(s) and contributor(s) and not of EWA Publishing and/or the editor(s). EWA Publishing and/or the editor(s) disclaim responsibility for any injury to people or property resulting from any ideas, methods, instructions or products referred to in the content.
About volume
Volume title: Proceedings of CONF-MLA 2024 Workshop: Securing the Future: Empowering Cyber Defense with Machine Learning and Deep Learning
© 2024 by the author(s). Licensee EWA Publishing, Oxford, UK. This article is an open access article distributed under the terms and
conditions of the Creative Commons Attribution (CC BY) license. Authors who
publish this series agree to the following terms:
1. Authors retain copyright and grant the series right of first publication with the work simultaneously licensed under a Creative Commons
Attribution License that allows others to share the work with an acknowledgment of the work's authorship and initial publication in this
series.
2. Authors are able to enter into separate, additional contractual arrangements for the non-exclusive distribution of the series's published
version of the work (e.g., post it to an institutional repository or publish it in a book), with an acknowledgment of its initial
publication in this series.
3. Authors are permitted and encouraged to post their work online (e.g., in institutional repositories or on their website) prior to and
during the submission process, as it can lead to productive exchanges, as well as earlier and greater citation of published work (See
Open access policy for details).
References
[1]. Alom, M. Z., Taha, T. M., Yakopcic, C., Westberg, S., Sidike, P., Nasrin, M. S., ... & Asari, V. K. (2019). A state-of-the-art survey on deep learning theory and architectures. electronics, 8(3), 292.
[2]. Chollet, F. (2021). Deep learning with Python. Simon and Schuster.
[3]. Li, Z., Liu, F., Yang, W., Peng, S., & Zhou, J. (2021). A survey of convolutional neural networks: analysis, applications, and prospects. IEEE transactions on neural networks and learning systems, 33(12), 6999-7019.
[4]. Ciancetta, F., Bucci, G., Fiorucci, E., Mari, S., & Fioravanti, A. (2020). A new convolutional neural network-based system for NILM applications. IEEE Transactions on Instrumentation and Measurement, 70, 1-12.
[5]. Zhang, Q., Yang, Q., Zhang, X., Wei, W., Bao, Q., Su, J., & Liu, X. (2022). A multi-label waste detection model based on transfer learning. Resources, Conservation and Recycling, 181, 106235.
[6]. Toğaçar, M., Ergen, B., & Cömert, Z. (2020). BrainMRNet: Brain tumor detection using magnetic resonance images with a novel convolutional neural network model. Medical hypotheses, 134, 109531.
[7]. Wankhade, M., Rao, A. C. S., & Kulkarni, C. (2022). A survey on sentiment analysis methods, applications, and challenges. Artificial Intelligence Review, 55(7), 5731-5780.
[8]. Birjali, M., Kasri, M., & Beni-Hssane, A. (2021). A comprehensive survey on sentiment analysis: Approaches, challenges and trends. Knowledge-Based Systems, 226, 107134.
[9]. IMDB Dataset of 50K Movie Reviews. (2019) URL: https://www.kaggle.com/datasets/lakshmi25npathi/imdb-dataset-of-50k-movie-reviews. Last Accessed 2024/08/23
[10]. Kim, Y. (2014). Convolutional neural networks for sentence classification. rXiv preprint arXiv: 1408.5882
[11]. Powers, D. M. (2020). Evaluation: from precision, recall and F-measure to ROC, informedness, markedness and correlation. arXiv preprint arXiv:2010.16061.
[12]. Ying, X. (2019). An overview of overfitting and its solutions. In Journal of physics: Conference series, 1168, 022022.