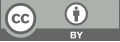
Enhancing WGAN performance by architectural and optimizer variations for image generation
- 1 School of Computer Science and Technology, East China Normal University, Shanghai, 200241, China
* Author to whom correspondence should be addressed.
Abstract
Generative model has opened up the area of image generation and has become a hot topic in recent years. Among the most famous generative models, Generative Adversarial Network (GAN) is outstanding among them, offering extensive avenues for exploration. The Wasserstein GAN (WGAN), as one of the GANs, introduces an innovative framework for training GANs based on the Earth Mover’s (Wasserstein) distance, providing a steadier training process. The experiment tried various modifications to WGAN, including changing the optimizers and the network architecture. Specifically, this work tried replacing the original Root Mean Square Prop (RMSprop) with another optimizers. Also, this work tried to add residual blocks to the network structure. These modifications provided interesting results, providing supplementary validation of the original WGAN structure, and providing some possibilities of optimization. According to the results, it could be found that the results of some modifications are very positive. However, some of the changes presented very unsatisfactory results, which gave us some insight.
Keywords
Image generation, Generative adversarial network, Optimizer
[1]. Creswell, A., White, T., Dumoulin, V., Arulkumaran, K., Sengupta, B., & Bharath, A. A. (2018). Generative adversarial networks: An overview. IEEE signal processing magazine, 35(1), 53-65.
[2]. Gui, J., Sun, Z., Wen, Y., Tao, D., & Ye, J. (2021). A review on generative adversarial networks: Algorithms, theory, and applications. IEEE transactions on knowledge and data engineering, 35(4), 3313-3332.
[3]. Goodfellow, I., Pouget-Abadie, J., Mirza, M., Xu, B., Warde-Farley, D., et, al. (2020). Generative adversarial networks. Communications of the ACM, 63(11), 139-144.
[4]. Aggarwal, A., Mittal, M., & Battineni, G. (2021). Generative adversarial network: An overview of theory and applications. International Journal of Information Management Data Insights, 1(1), 100004.
[5]. Durall, R., Chatzimichailidis, A., Labus, P., & Keuper, J. (2020). Combating mode collapse in gan training: An empirical analysis using hessian eigenvalues. arXiv preprint arXiv:2012.09673.
[6]. Martin A., Soumith C., Léon B. (2017). Wasserstein GAN. arXiv preprint arXiv: 1701.07875.
[7]. Rüschendorf, L. (1985). The Wasserstein distance and approximation theorems. Probability Theory and Related Fields, 70(1), 117-129.
[8]. He, K., Zhang, X., Ren, S., & Sun, J. (2016). Deep residual learning for image recognition. In Proceedings of the IEEE conference on computer vision and pattern recognition, 770-778.
[9]. Huang, G., Liu, Z., Van Der Maaten, L., & Weinberger, K. Q. (2017). Densely connected convolutional networks. In Proceedings of the IEEE conference on computer vision and pattern recognition, 4700-4708.
[10]. Yu, F., Seff, A., Zhang, Y., Song, S., Funkhouser, T., & Xiao, J. (2015). Lsun: Construction of a large-scale image dataset using deep learning with humans in the loop. arXiv preprint arXiv:1506.03365.
[11]. Cifar-10 Dataset. URL: https://www.cs.toronto.edu/~kriz/cifar.html. Last Accessed:2024/08/23.
Cite this article
Wang,L. (2024). Enhancing WGAN performance by architectural and optimizer variations for image generation. Applied and Computational Engineering,83,26-32.
Data availability
The datasets used and/or analyzed during the current study will be available from the authors upon reasonable request.
Disclaimer/Publisher's Note
The statements, opinions and data contained in all publications are solely those of the individual author(s) and contributor(s) and not of EWA Publishing and/or the editor(s). EWA Publishing and/or the editor(s) disclaim responsibility for any injury to people or property resulting from any ideas, methods, instructions or products referred to in the content.
About volume
Volume title: Proceedings of CONF-MLA 2024 Workshop: Semantic Communication Based Complexity Scalable Image Transmission System for Resource Constrained Devices
© 2024 by the author(s). Licensee EWA Publishing, Oxford, UK. This article is an open access article distributed under the terms and
conditions of the Creative Commons Attribution (CC BY) license. Authors who
publish this series agree to the following terms:
1. Authors retain copyright and grant the series right of first publication with the work simultaneously licensed under a Creative Commons
Attribution License that allows others to share the work with an acknowledgment of the work's authorship and initial publication in this
series.
2. Authors are able to enter into separate, additional contractual arrangements for the non-exclusive distribution of the series's published
version of the work (e.g., post it to an institutional repository or publish it in a book), with an acknowledgment of its initial
publication in this series.
3. Authors are permitted and encouraged to post their work online (e.g., in institutional repositories or on their website) prior to and
during the submission process, as it can lead to productive exchanges, as well as earlier and greater citation of published work (See
Open access policy for details).