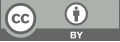
Machine learning-based football match prediction system
- 1 Department of Statistics and Data Science, Faculty of Science and Technology, BNU-HKBU United International College, Zhuhai, China
- 2 Department of Statistics and Data Science, Faculty of Science and Technology, BNU-HKBU United International College, Zhuhai, China
* Author to whom correspondence should be addressed.
Abstract
This study develops a machine learning-based system to predict English Premier League (EPL) outcomes, employing models such as Principal Component Analysis (PCA), K-Nearest Neighbors (KNN), Random Forests, and Support Vector Machines (SVM). The analysis covered a large dataset of matches, with the data normalized to ensure consistency and accuracy across models. Among the methods used, Random Forests showed the most robust performance in predicting match outcomes, particularly in forecasting wins and losses. However, both Random Forests and SVM encountered difficulties in accurately predicting draws, which points to areas where further refinement is needed. The prediction probabilities largely fell within a specific range, indicating the models' ability to identify patterns, but significant overfitting was observed in the models. This overfitting suggests that while the models performed well on the training data, they struggled to generalize to new, unseen data, highlighting the importance of implementing more effective regularization techniques to prevent overfitting and improve the models' overall predictive accuracy in real-world scenarios.
Keywords
Machine Learning Techniques, Football Data Analytics, Predictive Modeling, Team Performance Metrics
[1]. E. G. Dunning, J. A. Maguire, and R. E. Pearton, The Sports Process: A Comparative and Developmental Approach. Champaign, IL: Human Kinetics Publishers, 1993.
[2]. E. Ong and A. Flitman, “Using neural networks to predict binary outcomes,” in Proc. 1997 IEEE Int. Conf. on Intelligent Processing Systems (Cat. No. 97TH8335), vol. 1, IEEE, 1997, pp. 427–431.
[3]. J. Hucaljuk and A. Rakipović, “Predicting football scores using machine learning techniques,” in Proc. 2011 34th Int. Convention MIPRO, IEEE, 2011, pp. 1623–1627.
[4]. A. Joseph, N. E. Fenton, and M. Neil, “Predicting football results using Bayesian nets and other machine learning techniques,” Knowledge-Based Systems, vol. 19, no. 7, pp. 544–553, 2006.
[5]. J. Gomes, F. Portela, and M. F. Santos, “Decision support system for predicting football game result,” in Computers-19th Int. Conf. on Circuits, Systems, Communications and Computers-Intelligent Systems and Applications Special Sessions, vol. 32, 2015, pp. 348–353.
[6]. F. Rodrigues and Â. Pinto, “Prediction of football match results with machine learning,” Procedia Computer Science, vol. 204, pp. 463–470, 2022.
Cite this article
Wang,T.;Zhang,Z. (2024). Machine learning-based football match prediction system. Applied and Computational Engineering,92,181-186.
Data availability
The datasets used and/or analyzed during the current study will be available from the authors upon reasonable request.
Disclaimer/Publisher's Note
The statements, opinions and data contained in all publications are solely those of the individual author(s) and contributor(s) and not of EWA Publishing and/or the editor(s). EWA Publishing and/or the editor(s) disclaim responsibility for any injury to people or property resulting from any ideas, methods, instructions or products referred to in the content.
About volume
Volume title: Proceedings of the 6th International Conference on Computing and Data Science
© 2024 by the author(s). Licensee EWA Publishing, Oxford, UK. This article is an open access article distributed under the terms and
conditions of the Creative Commons Attribution (CC BY) license. Authors who
publish this series agree to the following terms:
1. Authors retain copyright and grant the series right of first publication with the work simultaneously licensed under a Creative Commons
Attribution License that allows others to share the work with an acknowledgment of the work's authorship and initial publication in this
series.
2. Authors are able to enter into separate, additional contractual arrangements for the non-exclusive distribution of the series's published
version of the work (e.g., post it to an institutional repository or publish it in a book), with an acknowledgment of its initial
publication in this series.
3. Authors are permitted and encouraged to post their work online (e.g., in institutional repositories or on their website) prior to and
during the submission process, as it can lead to productive exchanges, as well as earlier and greater citation of published work (See
Open access policy for details).