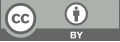
Advances in deep learning-based intelligent receivers
- 1 School of Computer and Communication Engineering, Northeastern University at Qinhuangdao, Hebei, China
- 2 School of Computer and Communication Engineering, Northeastern University at Qinhuangdao, Hebei, China
* Author to whom correspondence should be addressed.
Abstract
This paper presents a summary of the progress of deep learning in the research of intelligent receivers and predicts its future development direction. First, the background of the era of intelligent receivers is introduced. With the rapid development of communication technology, intelligent receivers show insufficient flexibility, low accuracy, and spectral efficiency in detecting current radio signals. This is a challenge that is difficult to overcome in order to meet the needs of modern signal recovery. With the advent of artificial intelligence technologies such as deep learning, academia and industry began to explore the potential of applying these techniques to the field of communication receivers, leading to the emergence of intelligent receivers. This paper then introduces the fundamentals of wireless communication systems and deep learning neural networks, laying the groundwork for a deeper understanding of the subsequent content. Chapter 3 provides a comprehensive overview of the application of intelligent receivers in signal reception, including channel estimation, signal detection, modulation identification, demodulation and decoding. Finally, the paper considers the practical applications of intelligent receivers and speculates on their future development.
Keywords
wireless communication, intelligent receivers, signal detection, deep learning.
[1]. Bhatia V, Tripathy M R, Ranjan P. Deep learning for massive MIMO: Challenges and future prospects[C]. //2020 IEEE 9th International Conference on Communication Systems and Network Technologies (CSNT). IEEE, 2020: 26-31.
[2]. He H, Wen C K, Jin S, et al. A model-driven deep learning network for MIMO detection[C]. //2018 IEEE Global Conference on Signal and Information Processing (GlobalSIP). IEEE, 2018: 584-588.
[3]. Kabacı A, Başaran M, Çırpan H A. Low-Complex AI-Empowered Receiver for Spatial Media-Based Modulation MIMO Systems[J]. IEEE Transactions on Vehicular Technology, 2023.
[4]. Zhang H, Zhang L, Cheng J, et al. An intelligent detection based on deep learning for multilevel code shifted differential chaos shift keying system with M-ary modulation[J]. IEEE Transactions on Cognitive Communications and Networking, 2021, 8(1): 155-169.
[5]. Xuan Q, Zhou J, Qiu K, et al. AvgNet: Adaptive visibility graph neural network and its application in modulation classification[J]. IEEE Transactions on Network Science and Engineering, 2022, 9(3): 1516-1526. Zhang H, Zhang L, Cheng J, et al. An intelligent detection based on deep learning for multilevel code shifted differential chaos shift keying system with M-ary modulation[J]. IEEE Transactions on Cognitive Communications and Networking, 2021, 8(1): 155-169.
[6]. Xiang B, Hu D, Wu J. Deep Learning-Based Downlink Channel Estimation for FDD Massive MIMO Systems[J]. IEEE Wireless Communications Letters, 2023, 12(4): 699-702.
[7]. He H, Wen C K, Jin S, et al. Deep learning-based channel estimation for beamspace mmWave massive MIMO systems[J]. IEEE Wireless Communications Letters, 2018, 7(5): 852-855.
[8]. Wei Y, Zhao M M, Zhao M, et al. An AMP-based network with deep residual learning for mmWave beamspace channel estimation[J]. IEEE Wireless Communications Letters, 2019, 8(4): 1289-1292.
[9]. Bai Q, Wang J, Zhang Y, et al. Deep learning-based channel estimation algorithm over time selective fading channels[J]. IEEE Transactions on Cognitive Communications and Networking, 2019, 6(1): 125-134.
[10]. Yoo S, Seo J, Park S, et al. Inter-Mode-Interference-Aware OAM Detector via Deep Learning[C]. //2023 IEEE 34th Annual International Symposium on Personal, Indoor and Mobile Radio Communications (PIMRC). IEEE, 2023: 1-6.
[11]. Zou J, Tao Y, Fang Y, et al. Receiver Design for ICI-CSK System: A New Perspective Based on GRU Neural Network[J]. IEEE Communications Letters, 2023.
[12]. Wei F, Zheng S, Zhou X, et al. Detection of direct sequence spread spectrum signals based on deep learning[J]. IEEE Transactions on Cognitive Communications and Networking, 2022, 8(3): 1399-1410.
[13]. Dong Y, Wu C, Zhu H, et al. A Weak Signal Detection Method Based on Spatial Spectrum-LSTM Neural Network[C]. //2022 5th International Conference on Information Communication and Signal Processing (ICICSP). IEEE, 2022: 1-6.
[14]. Huynh-The T, Pham Q V, Nguyen T V, et al. Automatic modulation classification: A deep architecture survey[J]. IEEE Access, 2021, 9: 142950-142971.
[15]. Zhou Q, Zhang R, Mu J, et al. Amcrn: Few-shot learning for automatic modulation classification[J]. IEEE Communications Letters, 2021, 26(3): 542-546.
[16]. Kim S H, Moon C B, Kim J W, et al. A hybrid deep learning model for automatic modulation classification[J]. IEEE Wireless Communications Letters, 2021, 11(2): 313-317.
[17]. Chia K, Baskaran V M. Deep learning-based demodulation of radio signal[C]. //2022 International Symposium on Intelligent Signal Processing and Communication Systems (ISPACS). IEEE, 2022: 1-4.
[18]. Zhang L, Zhang H, Jiang Y, et al. Intelligent and reliable deep learning LSTM neural networks-based OFDM-DCSK demodulation design[J]. IEEE Transactions on Vehicular Technology, 2020, 69(12): 16163-16167.
[19]. Qi P, Zhou X, Zheng S, et al. Automatic modulation classification based on deep residual networks with multimodal information[J]. IEEE Transactions on Cognitive Communications and Networking, 2020, 7(1): 21-33.
[20]. Shlezinger N, Fu R, Eldar Y C. DeepSIC: Deep soft interference cancellation for multiuser MIMO detection[J]. IEEE Transactions on Wireless Communications, 2020, 20(2): 1349-1362.
[21]. Mohammadkarimi M, Mehrabi M, Ardakani M, et al. Deep learning-based sphere decoding[J]. IEEE transactions on wireless communications, 2019, 18(9): 4368-4378.
[22]. Xu W, Zhang L, Wang T, et al. Deep learning for joint noncoherent differential detection and channel decoding[C]. //2021 IEEE/CIC International Conference on Communications in China (ICCC). IEEE, 2021: 589-594.
[23]. Besseghier M, Ghouali S, Djebbar A B. Optimized Greedy Detection for OFDM-IM Systems[J]. IEEE Communications Letters, 2023.
[24]. Kim J, Ro H, Park H. Deep learning-based detector for dual mode OFDM with index modulation[J]. IEEE Wireless Communications Letters, 2021, 10(7): 1562-1566.
[25]. Kim J, Park H. Deep Learning-Based Detector With Modulation Parameter-Independent Structure for OFDM With Index Modulation[J]. IEEE Access, 2023, 11: 130358-130367.
[26]. Xie Y, Teh K C, Kot A C. Deep learning-based joint detection for OFDM-NOMA scheme[J]. IEEE Communications Letters, 2021, 25(8): 2609-2613.
[27]. Emir A, Kara F, Kaya H, et al. Deep learning-based flexible joint channel estimation and signal detection of multi-user OFDM-NOMA[J]. Physical Communication, 2021, 48: 101443.
[28]. Wang B, Yuan Z, Zheng S, et al. Data-Driven Intelligent Receiver for OTFS Communication in Internet of Vehicles[J]. IEEE Transactions on Vehicular Technology, 2023.
[29]. Wang T, Wen C K, Wang H, et al. Deep learning for wireless physical layer: Opportunities and challenges[J]. China Communications, 2017, 14(11): 92-111.
Cite this article
Hu,J.;Li,M. (2024). Advances in deep learning-based intelligent receivers. Applied and Computational Engineering,95,95-106.
Data availability
The datasets used and/or analyzed during the current study will be available from the authors upon reasonable request.
Disclaimer/Publisher's Note
The statements, opinions and data contained in all publications are solely those of the individual author(s) and contributor(s) and not of EWA Publishing and/or the editor(s). EWA Publishing and/or the editor(s) disclaim responsibility for any injury to people or property resulting from any ideas, methods, instructions or products referred to in the content.
About volume
Volume title: Proceedings of the 6th International Conference on Computing and Data Science
© 2024 by the author(s). Licensee EWA Publishing, Oxford, UK. This article is an open access article distributed under the terms and
conditions of the Creative Commons Attribution (CC BY) license. Authors who
publish this series agree to the following terms:
1. Authors retain copyright and grant the series right of first publication with the work simultaneously licensed under a Creative Commons
Attribution License that allows others to share the work with an acknowledgment of the work's authorship and initial publication in this
series.
2. Authors are able to enter into separate, additional contractual arrangements for the non-exclusive distribution of the series's published
version of the work (e.g., post it to an institutional repository or publish it in a book), with an acknowledgment of its initial
publication in this series.
3. Authors are permitted and encouraged to post their work online (e.g., in institutional repositories or on their website) prior to and
during the submission process, as it can lead to productive exchanges, as well as earlier and greater citation of published work (See
Open access policy for details).