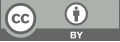
Coordination of NPCs in multi-agent systems based on behavior trees
- 1 Shanghai Jiao Tong University, Shanghai, China
- 2 University of Liverpool, Liverpool, England
- 3 North China Electric Power University, Beijing, China
* Author to whom correspondence should be addressed.
Abstract
This paper addresses the design and coordination challenges of controlling non-player characters' (NPCs) behaviors in multi-agent systems (MAS) using behavior trees (BTs), which are preferred over finite state machines (FSMs) due to their hierarchical structure and ease of maintenance. While BTs effectively resolve the question of "What to do next?" for individual NPCs, their application in MAS, particularly with the integration of a blackboard system for central control, reveals limitations in efficiency, robustness, and heuristic capacity as system complexity increases. To explore solutions to these challenges, this study analyzes various algorithms that enhance the functionality of behavior trees within MAS. The research focuses on three primary areas: the optimization of behavior trees, the development of behavior tree search algorithms, and the improvement of communication algorithms within BTs. Methods involve a comparative analysis of existing and new algorithmic approaches to identify and address inefficiencies in NPC coordination. The findings indicate that advanced behavior tree configurations, when combined with innovative search and communication strategies, significantly improve the coordination, robustness, and operational efficiency of MAS. These enhancements allow for more dynamic and responsive NPC interactions in complex gaming environments.
Keywords
Behavior Tree, Multi-Agent System, Non-Player Character.
[1]. Alex J. Champandard and Philip Dunstan. “The Behavior Tree Starter Kit”. In: Game AI Pro 360 (2019) . URL: https: //api. semanticscholar. org/CorpusID: 208121216.
[2]. Parasumanna Gokulan Balaji and Dipti Srinivasan. “An introduction to multi-agent systems”. In: Innovations in multi-agent systems and applications-1(2010) , pp. 1–27.
[3]. Li, M., Cai, Z., Yang, W., Wu, L.-X., Xu, Y. and Wang, J. (2021). Dec-SGTS: Decentralized Sub-Goal Tree Search for Multi-Agent Coordination. 35(13), pp.11282–11289. doi:https://doi.org/10.1609/aaai.v35i13.17345.
[4]. Sanjay Oruganti, Ramviyas Parasuraman, and Ra-mana Pidaparti. “IKT-BT: Indirect Knowledge Trans-fer Behavior Tree Framework for Multi-Robot Sys-tems Through Communication Eavesdropping”. In: arXiv preprint arXiv: 2312. 11802 (2023) .
[5]. Anja Johansson and Pierangelo Dell’Acqua. “Emotional behavior trees”. In: 2012 IEEE Conference on Computational Intelligence and Games (CIG) . 2012, pp. 355–362. DOI: 10. 1109/CIG. 2012. 6374177.
[6]. Ramiro A. Agis, Sebastian Gottifredi, and Alejandro Javier Garca. “An event-driven behavior trees extension to facilitate non-player multi-agent coordination in video games”. In: Expert Syst. Appl. 155 (2020) , p. 113457. URL: https: //api. semanticscholar. org/CorpusID: 218995637.
[7]. Andradi, S., Karunananda, A. and Ranawana, R. (2014). Human Behaviour Modelling for Games using Agents. International Conference on Advances in Engineering and Technology (ICAET’2014) March 29-30, 2014 Singapore. [online] doi:https://doi.org/10.15242/iie.e0314005.
[8]. Browne, C.B., Powley, E., Whitehouse, D., Lucas, S.M., Cowling, P.I., Rohlfshagen, P., Tavener, S., Perez, D., Samothrakis, S. and Colton, S. (2012). A Survey of Monte Carlo Tree Search Methods. IEEE Transactions on Computational Intelligence and AI in Games, [online] 4(1), pp.1–43. doi:https://doi.org/10.1109/tciaig.2012.2186810.
[9]. Best, G., Cliff, O.M., Patten, T., Mettu, R.R. and Fitch, R. (2018). Dec-MCTS: Decentralized planning for multi-robot active perception. The International Journal of Robotics Research, 38(2-3), pp.316–337. doi:https://doi.org/10.1177/0278364918755924.
[10]. Sukkar, F., Best, G., Yoo, C. and Fitch, R. (2019). Multi-Robot Region-of-Interest Reconstruction with Dec-MCTS. doi:https://doi.org/10.1109/icra.2019.8793560.
[11]. Ratnabala, L., Peter, R. and Charles, (2023). Evolutionary Swarm Robotics: Dynamic Subgoal-Based Path Formation and Task Allocation for Exploration and Navigation in Unknown Environments. [online] arXiv.org. Available at: https://doi.org/10.48550/arXiv.2312.16606 [Accessed 28 Sep. 2024].
[12]. Wang, Y. and Gelly, S. (2007). Modifications of UCT and sequence-like simulations for Monte-Carlo Go. 2007 IEEE Symposium on Computational Intelligence and Games. doi:https://doi.org/10.1109/cig.2007.368095.
[13]. Oruganti, S., Parasuraman, R. and Pidaparti, R. (2023). IKT-BT: Indirect Knowledge Transfer Behavior Tree Framework for Multi-Robot Systems Through Communication Eavesdropping. [online] arXiv.org. Available at: https://doi.org/10.48550/arXiv.2312.11802 [Accessed 28 Sep. 2024].
[14]. Skarzynski, K., Stepniak, M., Bartyna, W. and Ambroszkiewicz, S. (2017). SO-MRS: a multi-robot system architecture based on the SOA paradigm and ontology. [online] arXiv.org. Available at: https://doi.org/10.48550/arXiv.1709.03300 [Accessed 28 Sep. 2024].
[15]. Iovino, M., Scukins, E., Styrud, J., Ögren, P. and Smith, C. (2022). A survey of Behavior Trees in robotics and AI. Robotics and Autonomous Systems, 154, p.104096. doi:https://doi.org/10.1016/j.robot.2022.104096.
Cite this article
Hu,C.;Wu,R.;Deng,X. (2024). Coordination of NPCs in multi-agent systems based on behavior trees. Applied and Computational Engineering,95,126-140.
Data availability
The datasets used and/or analyzed during the current study will be available from the authors upon reasonable request.
Disclaimer/Publisher's Note
The statements, opinions and data contained in all publications are solely those of the individual author(s) and contributor(s) and not of EWA Publishing and/or the editor(s). EWA Publishing and/or the editor(s) disclaim responsibility for any injury to people or property resulting from any ideas, methods, instructions or products referred to in the content.
About volume
Volume title: Proceedings of the 6th International Conference on Computing and Data Science
© 2024 by the author(s). Licensee EWA Publishing, Oxford, UK. This article is an open access article distributed under the terms and
conditions of the Creative Commons Attribution (CC BY) license. Authors who
publish this series agree to the following terms:
1. Authors retain copyright and grant the series right of first publication with the work simultaneously licensed under a Creative Commons
Attribution License that allows others to share the work with an acknowledgment of the work's authorship and initial publication in this
series.
2. Authors are able to enter into separate, additional contractual arrangements for the non-exclusive distribution of the series's published
version of the work (e.g., post it to an institutional repository or publish it in a book), with an acknowledgment of its initial
publication in this series.
3. Authors are permitted and encouraged to post their work online (e.g., in institutional repositories or on their website) prior to and
during the submission process, as it can lead to productive exchanges, as well as earlier and greater citation of published work (See
Open access policy for details).