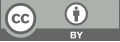
Revolutionizing education with AI: The adaptive cognitive enhancement model (ACEM) for personalized cognitive development
- 1 Xiangnan University, Hunian, China
* Author to whom correspondence should be addressed.
Abstract
The integration of artificial intelligence (AI) into education has opened doors to personalized learning experiences. This paper introduces the Adaptive Cognitive Enhancement Model (ACEM), a cutting-edge AI-driven framework designed to personalize cognitive development for students. Leveraging advanced machine learning algorithms and quantitative analysis, ACEM adapts educational content and learning strategies to individual cognitive needs. The model encompasses five key components: cognitive profiling, adaptive learning paths, intelligent feedback, motivational strategies, and longitudinal tracking. Through quantitative analysis and mathematical modeling, the paper demonstrates how ACEM significantly enhances learning outcomes compared to traditional education models. The discussion section provides a detailed exploration of each model component, its architecture, and its role in optimizing personalized cognitive development. Furthermore, challenges such as data privacy, scalability, and model interpretability are examined, alongside potential solutions. The conclusion underscores the transformative potential of ACEM in revolutionizing education.
Keywords
Abstract. The integration of artificial intelligence (AI) into education has opened doors to personalized learning experiences. This paper introduces the Adaptive Cognitive Enhancement Model (ACEM), a cutting-edge AI-driven framework designed to personalize cognitive development for students. Leveraging advanced machine learning algorithms and quantitative analysis, ACEM adapts educational content and learning strategies to individual cognitive needs. The model encompasses five key components: cognitive profiling, adaptive learning paths, intelligent feedback, motivational strategies, and longitudinal tracking. Through quantitative analysis and mathematical modeling, the paper demonstrates how ACEM significantly enhances learning outcomes compared to traditional education models. The discussion section provides a detailed exploration of each model component, its architecture, and its role in optimizing personalized cognitive development. Furthermore, challenges such as data privacy, scalability, and model interpretability are examined, alongside potential solutions. The conclusion underscores the transformative potential of ACEM in revolutionizing education.
[1]. Breines, Markus Roos, and Michael Gallagher. "A return to Teacherbot: rethinking the development of educational technology at the University of Edinburgh." Teaching in Higher Education 28.3 (2023): 517-531.
[2]. Adıgüzel, Tufan, Mehmet Haldun Kaya, and Fatih Kürşat Cansu. "Revolutionizing education with AI: Exploring the transformative potential of ChatGPT." Contemporary Educational Technology (2023).
[3]. Kovalchuk, Vasyl I., Svitlana V. Maslich, and Larysa H. Movchan. "Digitalization of vocational education under crisis conditions." Educational Technology Quarterly 2023.1 (2023): 1-17.
[4]. Khamidovna, Pulatova Odina. "Features of the Use of Modern Didactic Tools in Technical Higher Educational Institutions." Telematique 21 (2023): 7630-7634.
[5]. Antonietti, Chiara, et al. "Development and validation of the ICAP Technology Scale to measure how teachers integrate technology into learning activities." Computers & Education 192 (2023): 104648.
[6]. Chan, Cecilia Ka Yuk, and Wenjie Hu. "Students’ voices on generative AI: Perceptions, benefits, and challenges in higher education." International Journal of Educational Technology in Higher Education 20.1 (2023): 43.
[7]. Gillani, Nabeel, et al. "Unpacking the “Black Box” of AI in education." Educational Technology & Society 26.1 (2023): 99-111.
[8]. Rajendran, Rajashree Manjulalayam. "Code-driven Cognitive Enhancement: Customization and Extension of Azure Cognitive Services in. NET." Journal of Science & Technology 4.6 (2023): 45-54.
[9]. Wan, Haipeng, and Shengquan Yu. "A recommendation system based on an adaptive learning cognitive map model and its effects." Interactive Learning Environments 31.3 (2023): 1821-1839.
[10]. Pachankis, John E., et al. "A model for adapting evidence-based interventions to be LGBQ-affirmative: Putting minority stress principles and case conceptualization into clinical research and practice." Cognitive and Behavioral Practice 30.1 (2023): 1-17.
[11]. Oyebode, Oladapo, et al. "Machine learning techniques in adaptive and personalized systems for health and wellness." International Journal of Human–Computer Interaction 39.9 (2023): 1938-1962.
Cite this article
Luo,Y. (2024). Revolutionizing education with AI: The adaptive cognitive enhancement model (ACEM) for personalized cognitive development. Applied and Computational Engineering,82,71-76.
Data availability
The datasets used and/or analyzed during the current study will be available from the authors upon reasonable request.
Disclaimer/Publisher's Note
The statements, opinions and data contained in all publications are solely those of the individual author(s) and contributor(s) and not of EWA Publishing and/or the editor(s). EWA Publishing and/or the editor(s) disclaim responsibility for any injury to people or property resulting from any ideas, methods, instructions or products referred to in the content.
About volume
Volume title: Proceedings of the 2nd International Conference on Machine Learning and Automation
© 2024 by the author(s). Licensee EWA Publishing, Oxford, UK. This article is an open access article distributed under the terms and
conditions of the Creative Commons Attribution (CC BY) license. Authors who
publish this series agree to the following terms:
1. Authors retain copyright and grant the series right of first publication with the work simultaneously licensed under a Creative Commons
Attribution License that allows others to share the work with an acknowledgment of the work's authorship and initial publication in this
series.
2. Authors are able to enter into separate, additional contractual arrangements for the non-exclusive distribution of the series's published
version of the work (e.g., post it to an institutional repository or publish it in a book), with an acknowledgment of its initial
publication in this series.
3. Authors are permitted and encouraged to post their work online (e.g., in institutional repositories or on their website) prior to and
during the submission process, as it can lead to productive exchanges, as well as earlier and greater citation of published work (See
Open access policy for details).